A Private and Computationally-Efficient Estimator for Unbounded Gaussians.
Annual Conference on Computational Learning Theory(2022)
摘要
We give the first polynomial-time, polynomial-sample, differentially private estimator for the mean and covariance of an arbitrary Gaussian distribution $N(\mu,\Sigma)$ in $\R^d$. All previous estimators are either nonconstructive, with unbounded running time, or require the user to specify a priori bounds on the parameters $\mu$ and $\Sigma$. The primary new technical tool in our algorithm is a new differentially private preconditioner that takes samples from an arbitrary Gaussian $N(0,\Sigma)$ and returns a matrix $A$ such that $A \Sigma A^T$ has constant condition number
更多查看译文
关键词
unbounded gaussians,computationally-efficient
AI 理解论文
溯源树
样例
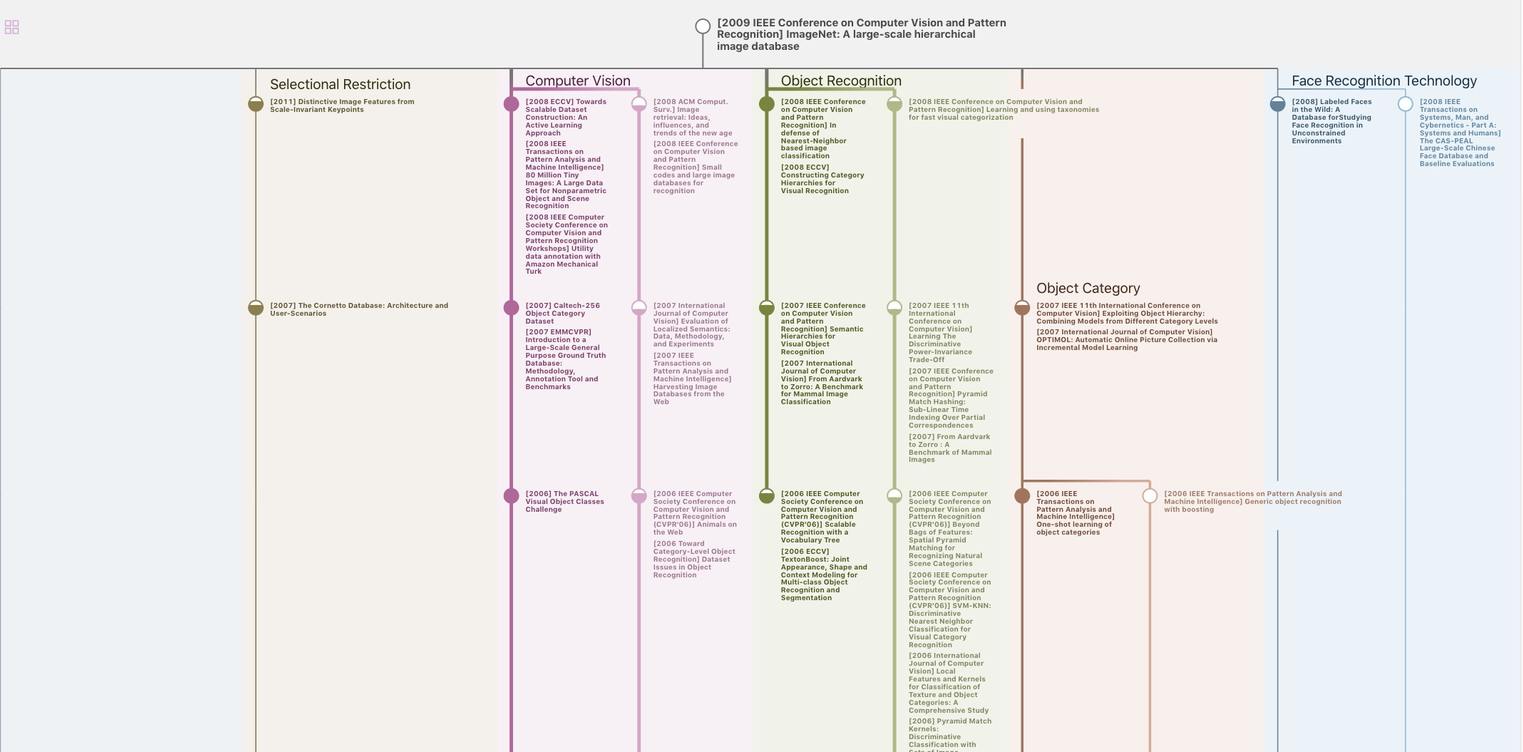
生成溯源树,研究论文发展脉络
Chat Paper
正在生成论文摘要