Revalidating US Army soldiers' perceptions of combat arms job tasks: Frequencies, importance and expectations of performance
WORK-A JOURNAL OF PREVENTION ASSESSMENT & REHABILITATION(2021)
摘要
BACKGROUND: In 2013 the U.S. Army began developing physical tests to predict a recruit's ability to perform the critical physically demanding tasks (CPDTs) of combat arms jobs not previously open to women. OBJECTIVE: To revalidate 15 CPDTs chosen by subject matter experts (SMEs) and researchers through questionnaires pertaining to task performance frequency, perceived importance, and performance expectations. METHOD: Web-administered job analysis questionnaires were completed by 2,090 soldiers. Seventy-three percent ranged between 25-38 years of age, 66% were staff sergeants or above, and 73% were in service for 7 + years. RESULTS: Overall, the nine SME-endorsed CPDTs were conducted more frequently and rated as more important than the six tasks identified by researchers. Foot march, dragging a casualty to safety, and connecting a tow bar (vehicle transport) were identified as the combined most important, most frequently performed and highly expected CPDTs to be performed. The canonical correlation between task performance frequencies and ratings of task importance across all 15 CPDTs was 0.82 (p < 0.001). Expectations of task completion were strongly associated with more frequent task performance (Cramer's Vs ranged 0.22 to 0.71; all p's < 0.001), but not task importance (only four CPDTs at p < 0.05). CONCLUSION: This study revalidates the value of CPDTs chosen by SMEs and researchers. Soldier readiness should reflect tasks identified by incumbents as important to success (e.g., evacuating a casualty) be trained more often, whereas others classified as frequently performed, but less important (e.g., filling sandbags), be deemphasized while ensuring that standards are met.
更多查看译文
关键词
Military effectiveness, common tasks, military tasks, physical demands, job analysis
AI 理解论文
溯源树
样例
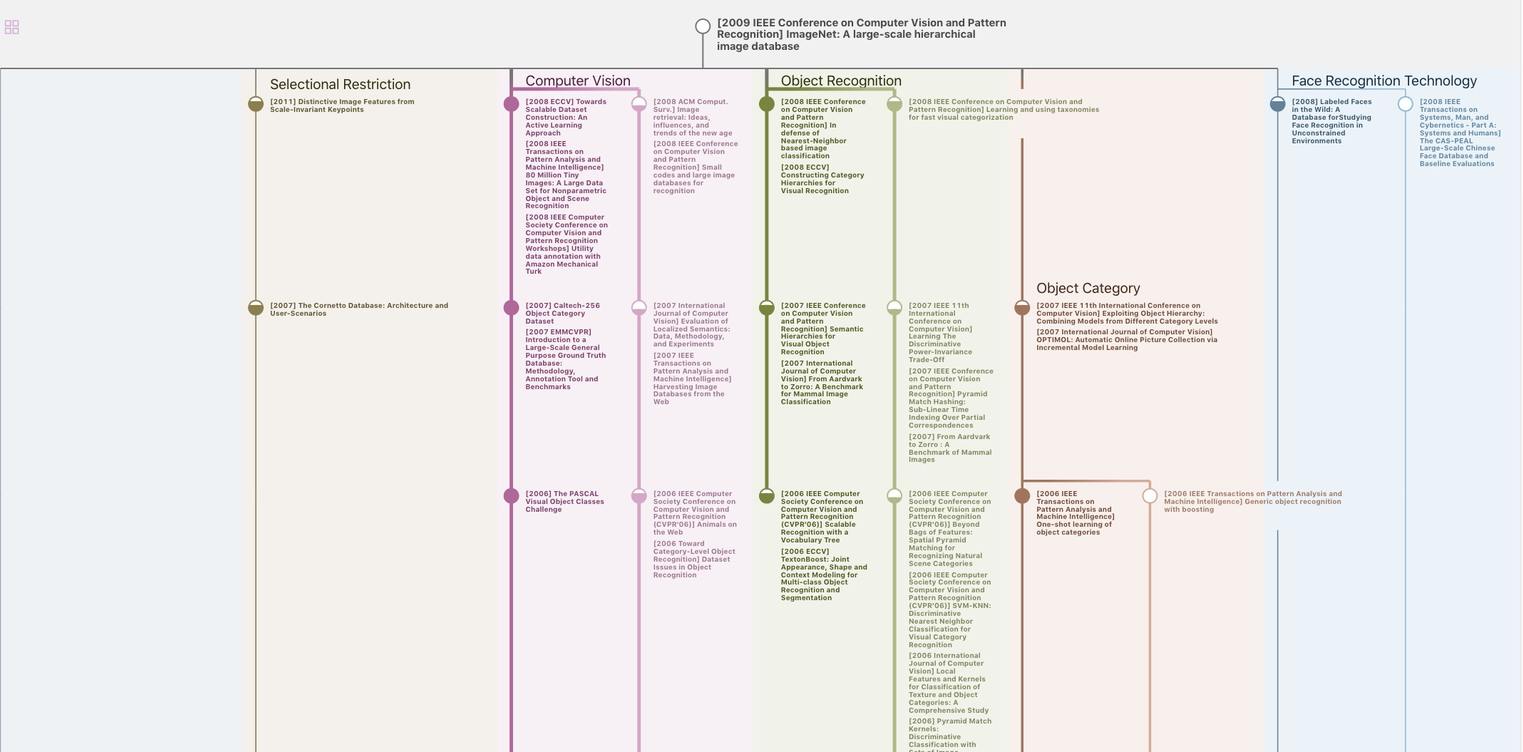
生成溯源树,研究论文发展脉络
Chat Paper
正在生成论文摘要