Improving Generalization of Deepfake Detection with Domain Adaptive Batch Normalization
International Multimedia Conference(2021)
摘要
ABSTRACTDeepfake, a well-known face forgery technique, has raised serious concerns about personal privacy and social media security. Therefore, a plenty of deepfake detection methods come out and achieve outstanding performance in the single dataset case. However, current deepfake detection methods fail to perform strong generalization ability in cross-dataset case due to the domain gap. To tackle this issue, we propose Domain Adaptive Batch Normalization (DABN) strategy to mitigate the domain distribution gap on different datasets. Specifically, DABN utilizes the distribution statistics of the testing dataset in replace of the original counterparts so as to avoid distribution mismatch and restore the effectiveness of BN layers. Equipped with our DABN, detection method can be more robust when generalized into a broader usage. Note that our method is flexible and can be further employed on most existing deepfake detection methods during testing, which shows a great practical value. Extensive experiments on multiple datasets and models demonstrate the effectiveness of DABN. The proposed method achieves an average accuracy improvement by nearly 20% of existing strategies on Celeb-DF dataset under black-box settings, indicating strong enhancement of generalization ability of the deepfake detection models.
更多查看译文
AI 理解论文
溯源树
样例
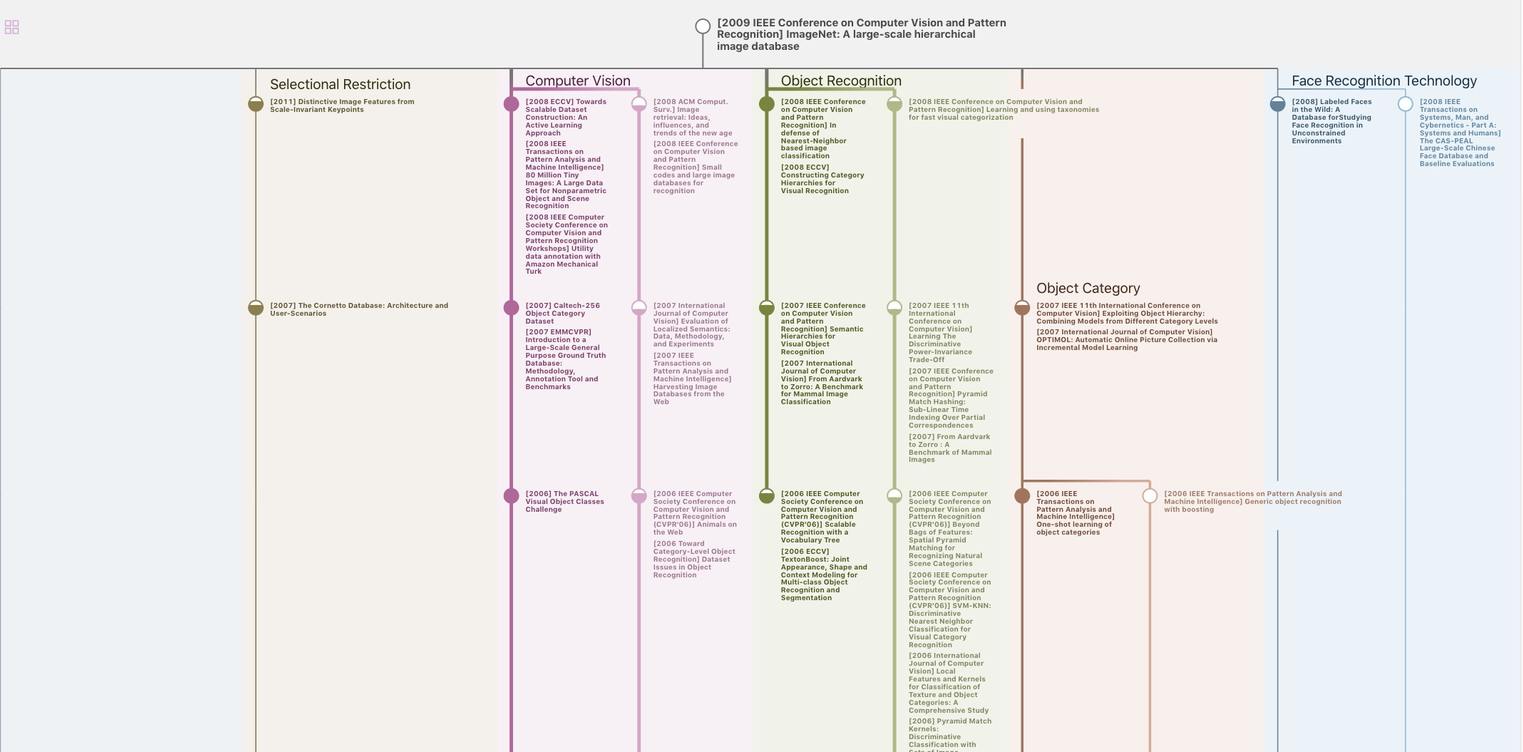
生成溯源树,研究论文发展脉络
Chat Paper
正在生成论文摘要