Data-Driven Prediction with Stochastic Data: Confidence Regions and Minimum Mean-Squared Error Estimates
2022 EUROPEAN CONTROL CONFERENCE (ECC)(2022)
摘要
Recently, direct data-driven prediction has found important applications for controlling unknown systems, par-ticularly in predictive control. Such an approach provides exact prediction using behavioral system theory when noise-free data are available. For stochastic data, although approximate predictors exist based on different statistical criteria, they fail to provide statistical guarantees of prediction accuracy. In this paper, confidence regions are provided for these stochastic pre-dictors based on the prediction error distribution. Leveraging this, an optimal predictor which achieves minimum mean-squared prediction error is also proposed to enhance prediction accuracy. These results depend on some true model parameters, but they can also be replaced with an approximate data-driven formulation in practice. Numerical results show that the derived confidence region is valid and smaller prediction errors are observed for the proposed minimum mean-squared error estimate, even with the approximate data-driven formulation.
更多查看译文
关键词
prediction,stochastic data-driven,confidence regions,mean-squared
AI 理解论文
溯源树
样例
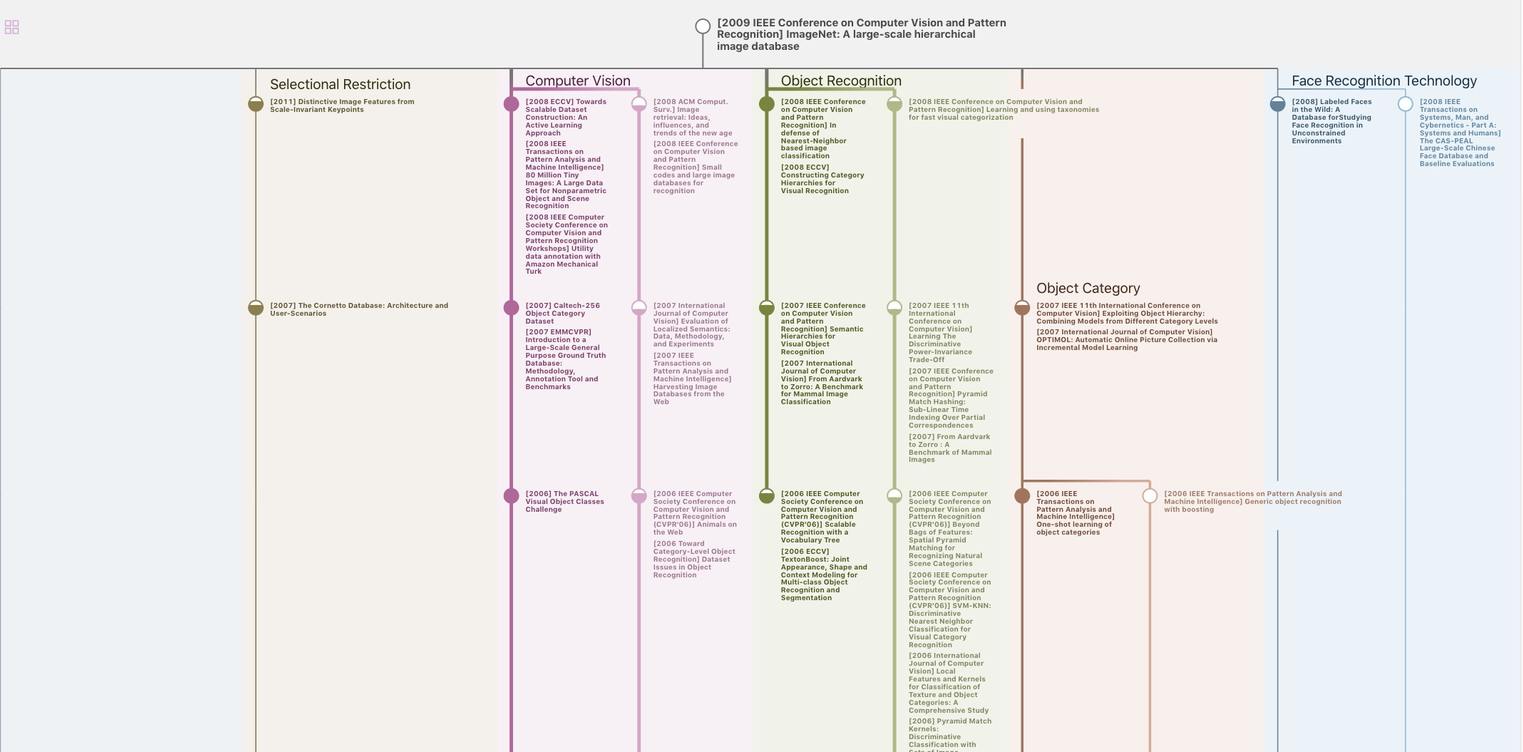
生成溯源树,研究论文发展脉络
Chat Paper
正在生成论文摘要