Learning from Multiple Time Series: A Deep Disentangled Approach to Diversified Time Series Forecasting
arxiv(2021)
摘要
Time series forecasting is a significant problem in many applications, e.g., financial predictions and business optimization. Modern datasets can have multiple correlated time series, which are often generated with global (shared) regularities and local (specific) dynamics. In this paper, we seek to tackle such forecasting problems with DeepDGL, a deep forecasting model that disentangles dynamics into global and local temporal patterns. DeepDGL employs an encoder-decoder architecture, consisting of two encoders to learn global and local temporal patterns, respectively, and a decoder to make multi-step forecasting. Specifically, to model complicated global patterns, the vector quantization (VQ) module is introduced, allowing the global feature encoder to learn a shared codebook among all time series. To model diversified and heterogenous local patterns, an adaptive parameter generation module enhanced by the contrastive multi-horizon coding (CMC) is proposed to generate the parameters of the local feature encoder for each individual time series, which maximizes the mutual information between the series-specific context variable and the long/short-term representations of the corresponding time series. Our experiments on several real-world datasets show that DeepDGL outperforms existing state-of-the-art models.
更多查看译文
关键词
deep disentangled approach,multiple time series,learning
AI 理解论文
溯源树
样例
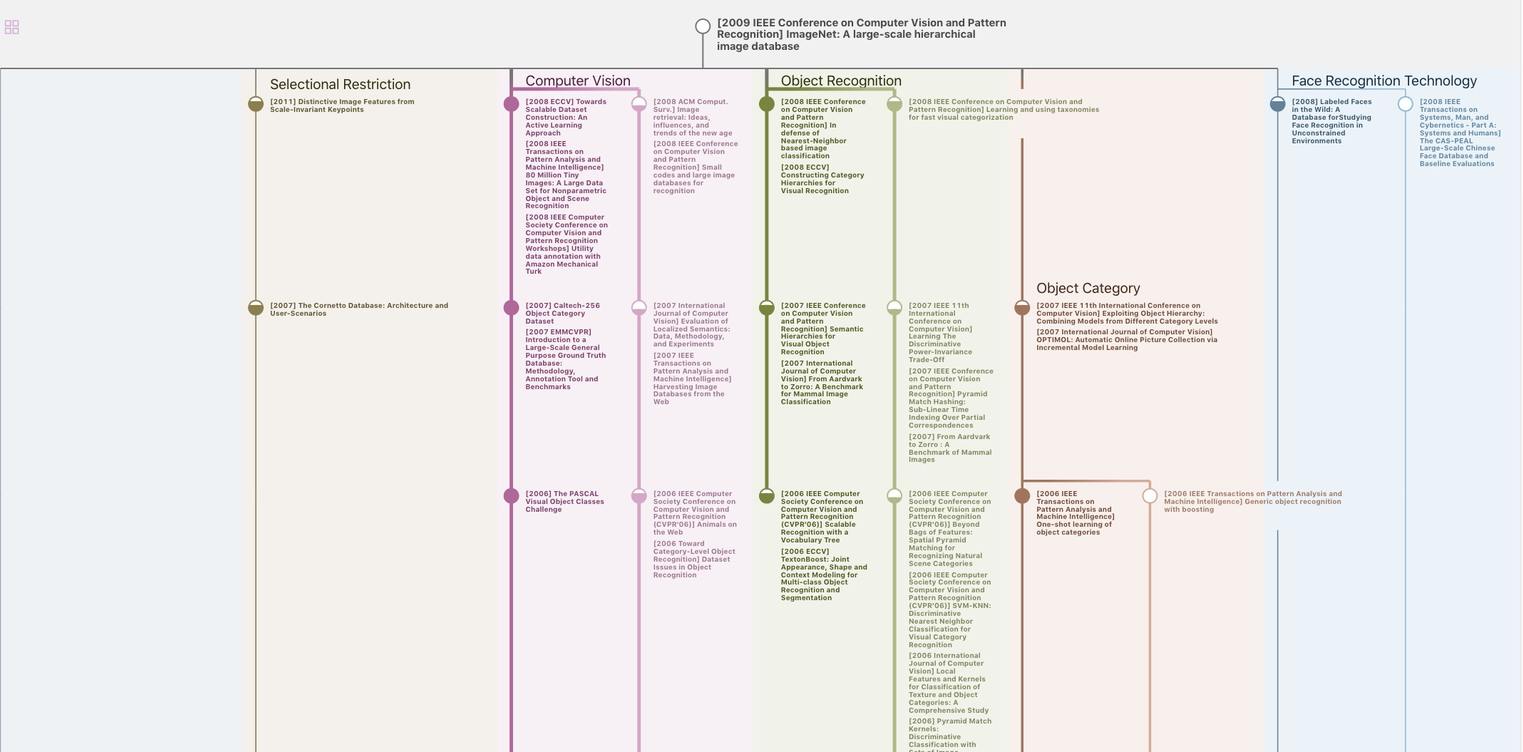
生成溯源树,研究论文发展脉络
Chat Paper
正在生成论文摘要