Changepoint detection in non-exchangeable data
Stat. Comput.(2022)
摘要
Changepoint models typically assume the data within each segment are independent and identically distributed conditional on some parameters that change across segments. This construction may be inadequate when data are subject to local correlation patterns, often resulting in many more changepoints fitted than preferable. This article proposes a Bayesian changepoint model that relaxes the assumption of exchangeability within segments. The proposed model supposes data within a segment are m -dependent for some unknown m ⩾ 0 that may vary between segments, resulting in a model suitable for detecting clear discontinuities in data that are subject to different local temporal correlations. The approach is suited to both continuous and discrete data. A novel reversible jump Markov chain Monte Carlo algorithm is proposed to sample from the model; in particular, a detailed analysis of the parameter space is exploited to build proposals for the orders of dependence. Two applications demonstrate the benefits of the proposed model: computer network monitoring via change detection in count data, and segmentation of financial time series.
更多查看译文
关键词
Changepoint detection,Dependent data,Reversible jump MCMC
AI 理解论文
溯源树
样例
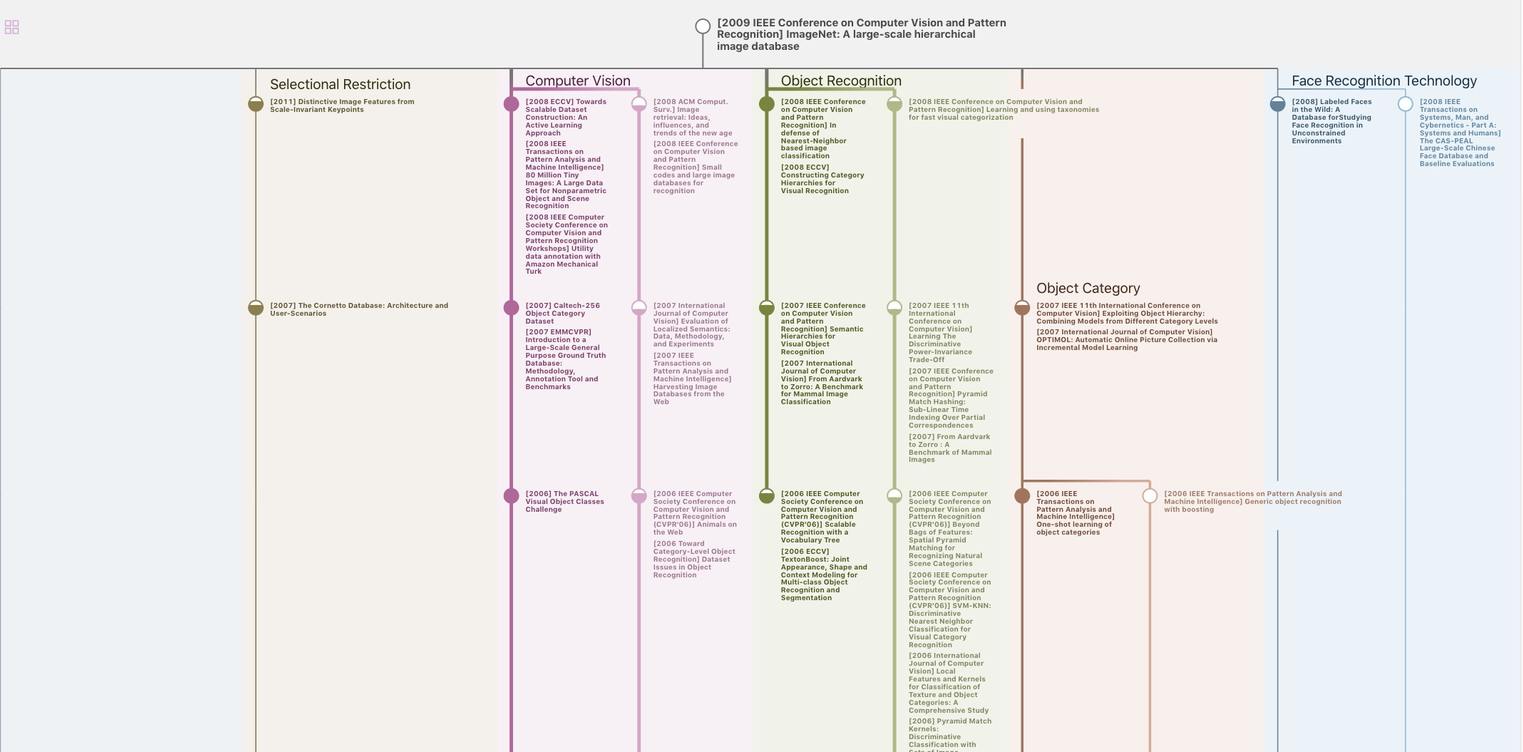
生成溯源树,研究论文发展脉络
Chat Paper
正在生成论文摘要