Mode connectivity in the loss landscape of parameterized quantum circuits
Quantum Machine Intelligence(2022)
摘要
Variational training of parameterized quantum circuits (PQCs) underpins many workflows employed on near-term noisy intermediate scale quantum (NISQ) devices. It is a hybrid quantum-classical approach that minimizes an associated cost function in order to train a parameterized ansatz. In this paper we adapt the qualitative loss landscape characterization for neural networks introduced in Goodfellow et al. ( 2014 ); Li et al. ( 2017 ) and tests for connectivity used in Draxler et al. ( 2018 ) to study the loss landscape features in PQC training. We present results for PQCs trained on a simple regression task, using the bilayer circuit ansatz, which consists of alternating layers of parameterized rotation gates and entangling gates. Multiple circuits are trained with 3 different batch gradient optimizers: stochastic gradient descent, the quantum natural gradient, and Adam. We identify large features in the landscape that can lead to faster convergence in training workflows.
更多查看译文
关键词
Quantum machine learning, Gradient-based optimization, Visualization, Circuit design, Mode connectivity
AI 理解论文
溯源树
样例
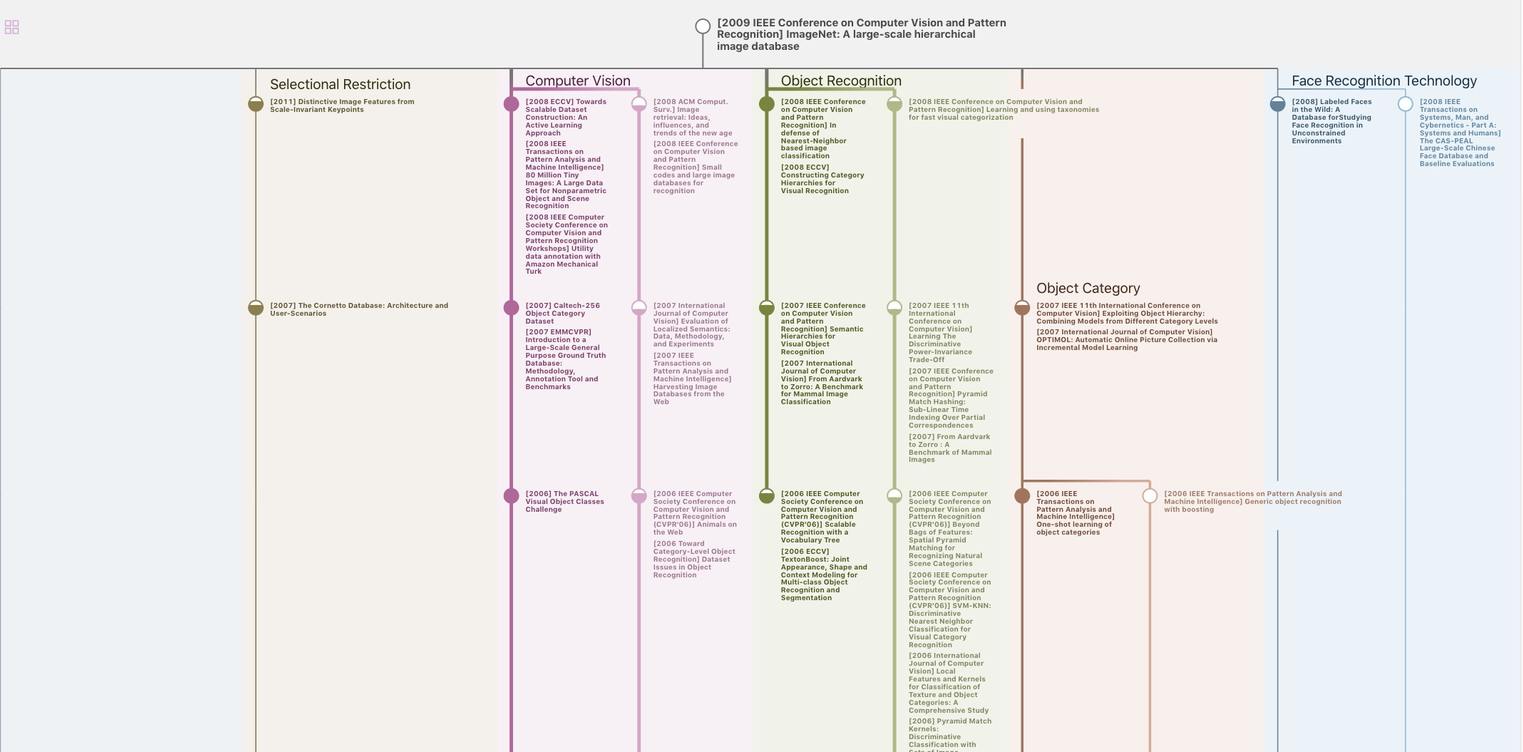
生成溯源树,研究论文发展脉络
Chat Paper
正在生成论文摘要