Refine for Semantic Segmentation Based on Parallel Convolutional Network with Attention Model
Neural Process. Lett.(2021)
摘要
High-precision semantic segmentation methods require global information and more detailed local features. It is difficult for ordinary convolutional neural networks to efficiently use this information. In response to the above issues, this paper uses the attention to scale method and proposes a novel attention model for semantic segmentation, which aggregates multi-scale and context features to refine prediction. Specifically, the skeleton convolutional neural network framework takes in multiple different scales inputs, by which means the CNN can get representations in different scales. The proposed attention model will handle the features from different scale streams respectively and integrate them. Then location attention branch of the model learns to softly weight the multi-scale features at each pixel location. Moreover, we add an recalibrating branch, parallel to where location attention comes out, to recalibrate the score map per class. We achieve quite competitive results on PASCAL VOC 2012 and ADE20K datasets, which surpass baseline and related works.
更多查看译文
关键词
Semantic segmentation,Parallel convolutional network,Attention model,Multi-scale,Multi-dilation
AI 理解论文
溯源树
样例
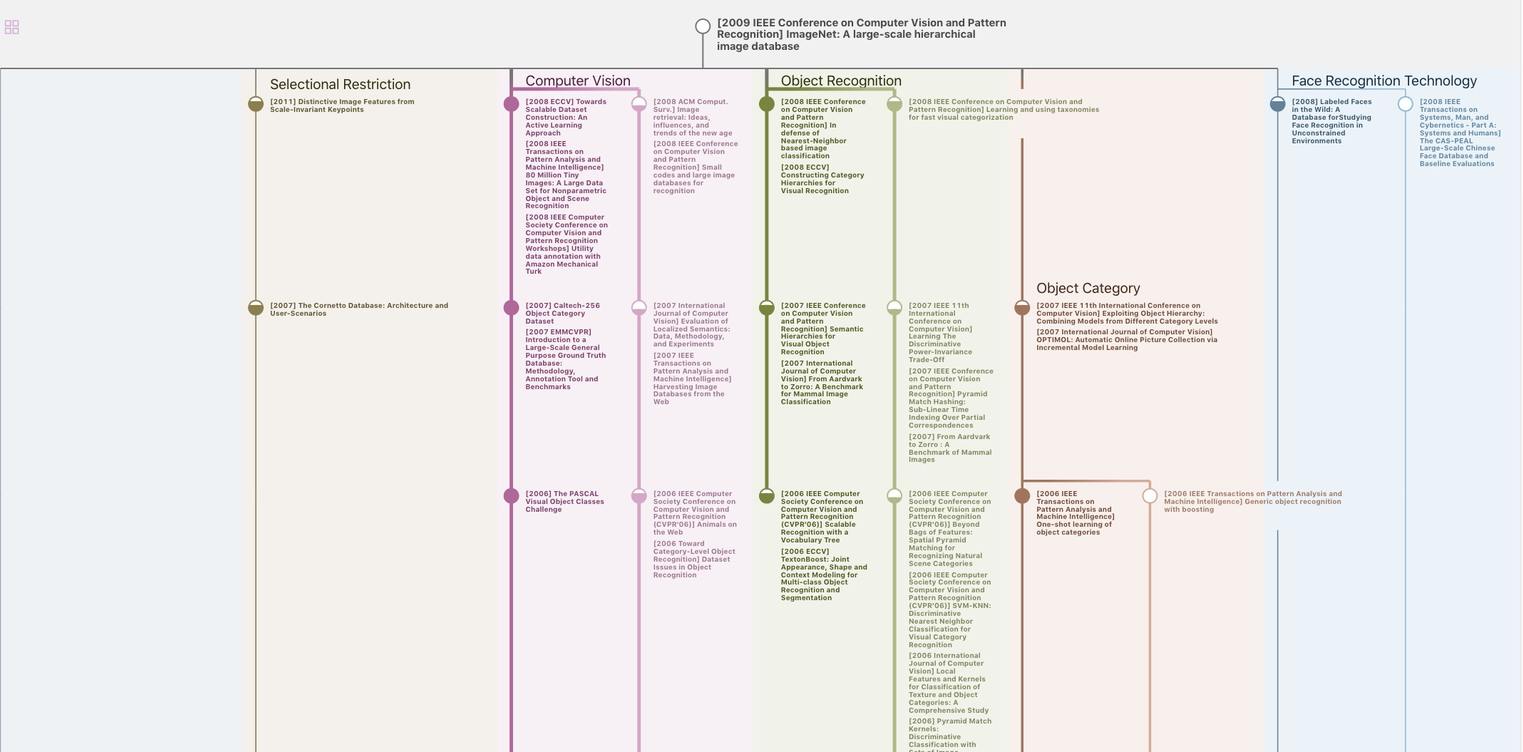
生成溯源树,研究论文发展脉络
Chat Paper
正在生成论文摘要