Joint Learning of Feature Detector and Descriptor for Visual SLAM
2021 32ND IEEE INTELLIGENT VEHICLES SYMPOSIUM (IV)(2021)
摘要
Visual Simultaneous Localization and Mapping is one of the main challenges for robotics and automated vehicles. In the state-of-the-art approaches, pixel-level correspondences are mostly used. In this paper, we address the problem of finding stable and repeatable pixel-level correspondences under challenging conditions. As a network basis, we use the D2-Net, which can select keypoints at positions that can be matched easily. Several techniques are implemented in this work to improve the keypoint detection and description performance. We first feed the network with rotated images to achieve a higher rotation invariance of point detections. Furthermore, we analyze the impact of a ranking of score values, adopting a cosine similarity and enforcing more dominant detections by defining a peakiness. The evaluation shows that combining a ranking with a peakiness can achieve the best result, especially to illumination changes. By using this combination, we achieve a mean matching accuracy increase of 12.5% on illumination scenes (9% overall) and a 9% higher repeatability rate at extremely low costs by only modifying the loss function.
更多查看译文
关键词
network basis,D2-Net,keypoint detection,description performance,rotated images,higher rotation invariance,point detections,score values,cosine similarity,dominant detections,peakiness,mean matching accuracy increase,higher repeatability rate,joint learning,feature detector,visual SLAM,Visual Simultaneous Localization,robotics,stable pixel-level correspondences,repeatable pixel-level correspondences
AI 理解论文
溯源树
样例
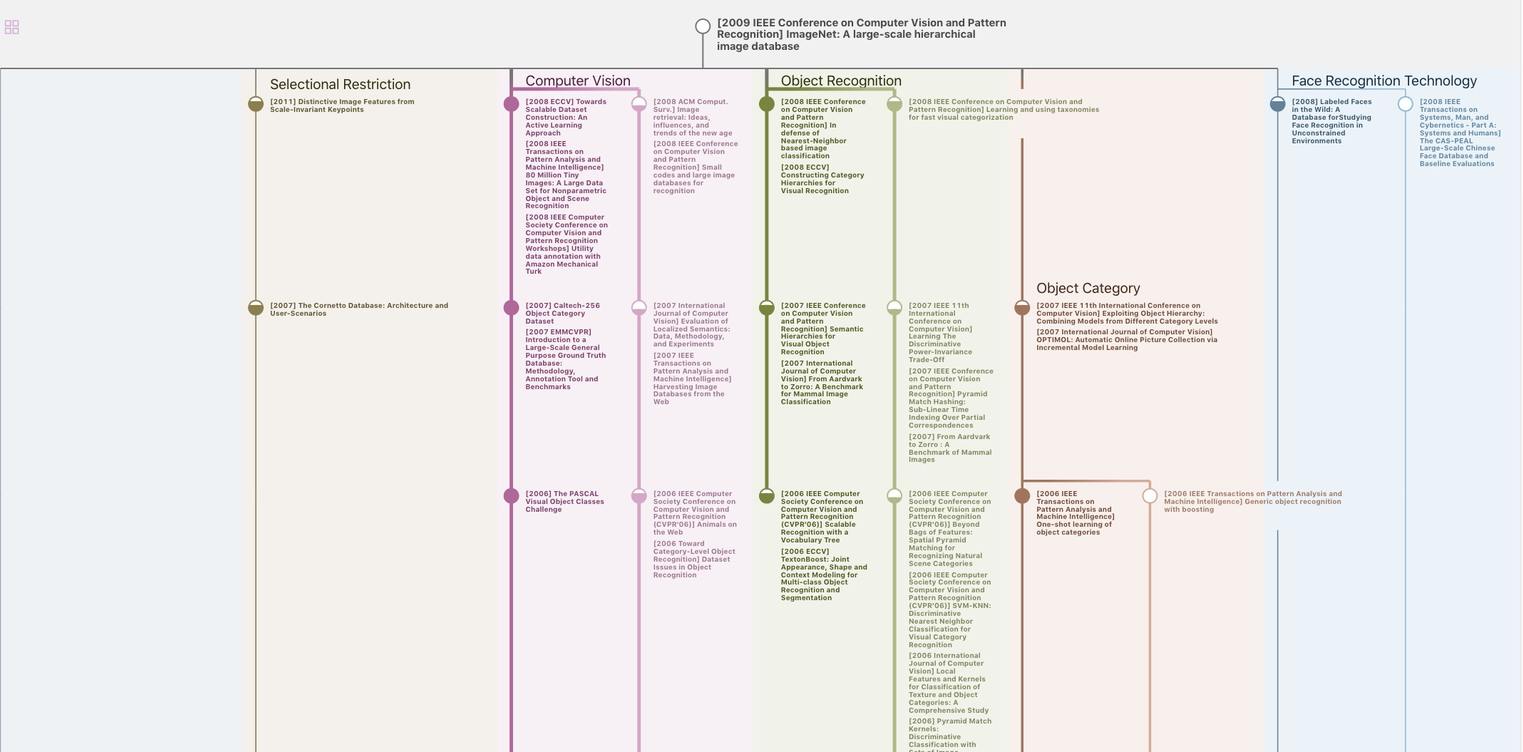
生成溯源树,研究论文发展脉络
Chat Paper
正在生成论文摘要