Traffic4D: Single View Reconstruction of Repetitious Activity Using Longitudinal Self-Supervision
2021 32ND IEEE INTELLIGENT VEHICLES SYMPOSIUM (IV)(2021)
摘要
Reconstructing 4D vehicular activity (3D space and time) from cameras is useful for autonomous vehicles, commuters and local authorities to plan for smarter and safer cities. Traffic is inherently repetitious over long periods, yet current deep learning-based 3D reconstruction methods have not considered such repetitions and have difficulty generalizing to new intersection-installed cameras. We present a novel approach exploiting longitudinal (long-term) repetitious motion as self-supervision to reconstruct 3D vehicular activity from a video captured by a single fixed camera. Starting from off-the-shelf 2D keypoint detections, our algorithm optimizes 3D vehicle shapes and poses, and then clusters their trajectories in 3D space. The 2D keypoints and trajectory clusters accumulated over long-term are later used to improve the 2D and 3D keypoints via self-supervision without any human annotation. Our method improves reconstruction accuracy over state of the art on scenes with a significant visual difference from the keypoint detector's training data, and has many applications including velocity estimation, anomaly detection and vehicle counting. We demonstrate results on traffic videos captured at multiple city intersections, collected using our smartphones, YouTube, and other public datasets.
更多查看译文
关键词
single view reconstruction,repetitious activity,longitudinal self-supervision,4D vehicular activity,autonomous vehicles,local authorities,smarter cities,safer cities,current deep learning-based 3D reconstruction methods,intersection-installed cameras,3D vehicular activity,single fixed camera,off-the-shelf 2D keypoint detections,trajectory clusters,reconstruction accuracy,keypoint detector,anomaly detection,vehicle counting,traffic videos,multiple city intersections
AI 理解论文
溯源树
样例
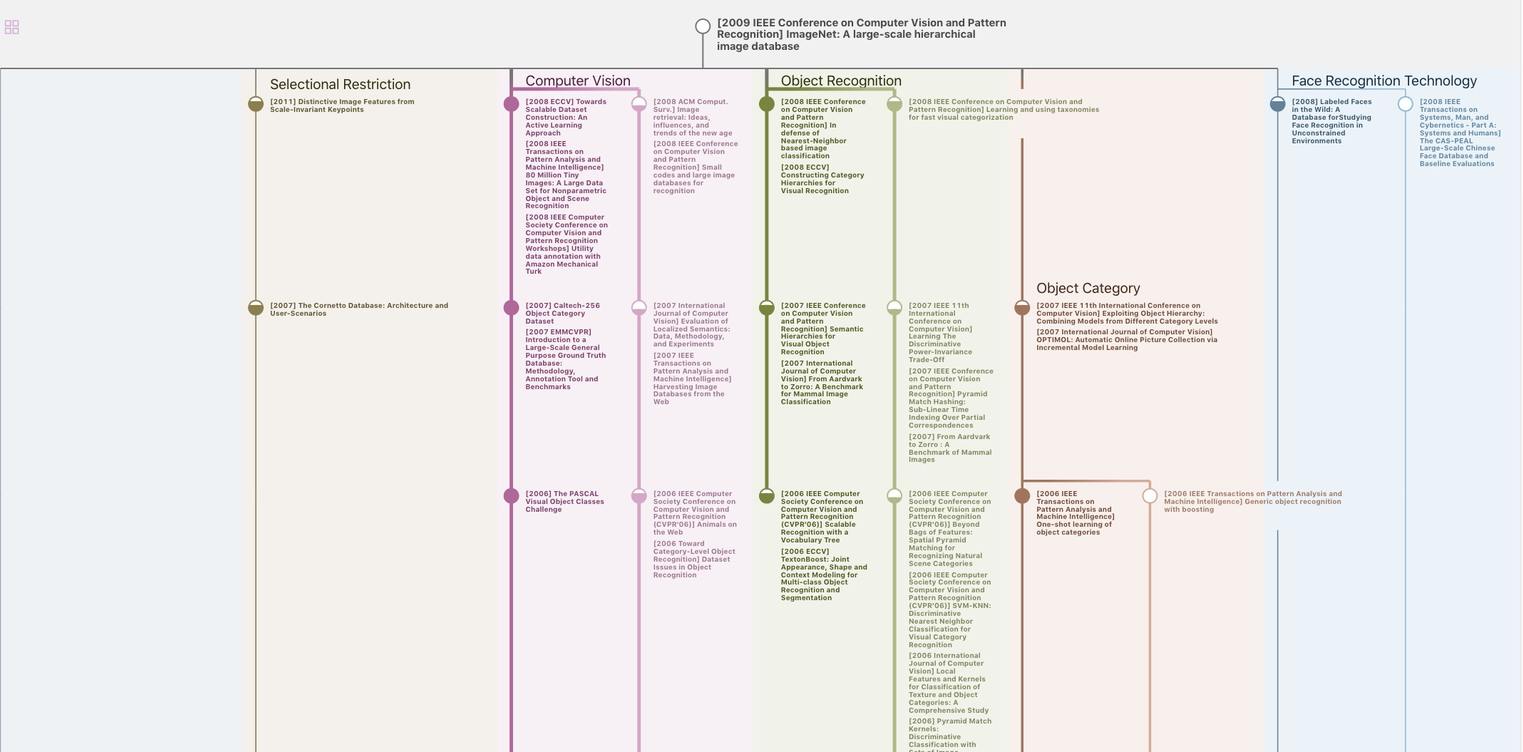
生成溯源树,研究论文发展脉络
Chat Paper
正在生成论文摘要