Few-Shot Text Ranking with Meta Adapted Synthetic Weak Supervision.
59TH ANNUAL MEETING OF THE ASSOCIATION FOR COMPUTATIONAL LINGUISTICS AND THE 11TH INTERNATIONAL JOINT CONFERENCE ON NATURAL LANGUAGE PROCESSING (ACL-IJCNLP 2021), VOL 1(2021)
摘要
The effectiveness of Neural Information Retrieval (Neu-IR) often depends on a large scale of in-domain relevance training signals, which are not always available in real-world ranking scenarios. To democratize the benefits of NeuIR, this paper presents MetaAdaptRank, a domain adaptive learning method that generalizes Neu-IR models from label-rich source domains to few-shot target domains. Drawing on source-domain massive relevance supervision, MetaAdaptRank contrastively synthesizes a large number of weak supervision signals for target domains and meta-learns to reweight these synthetic "weak" data based on their benefits to the target-domain ranking accuracy of Neu-IR models. Experiments on three TREC benchmarks in the web, news, and biomedical domains show that MetaAdaptRank significantly improves the few-shot ranking accuracy of Neu-IR models. Further analyses indicate that MetaAdaptRank thrives from both its contrastive weak data synthesis and meta-reweighted data selection.
更多查看译文
关键词
text,meta,few-shot
AI 理解论文
溯源树
样例
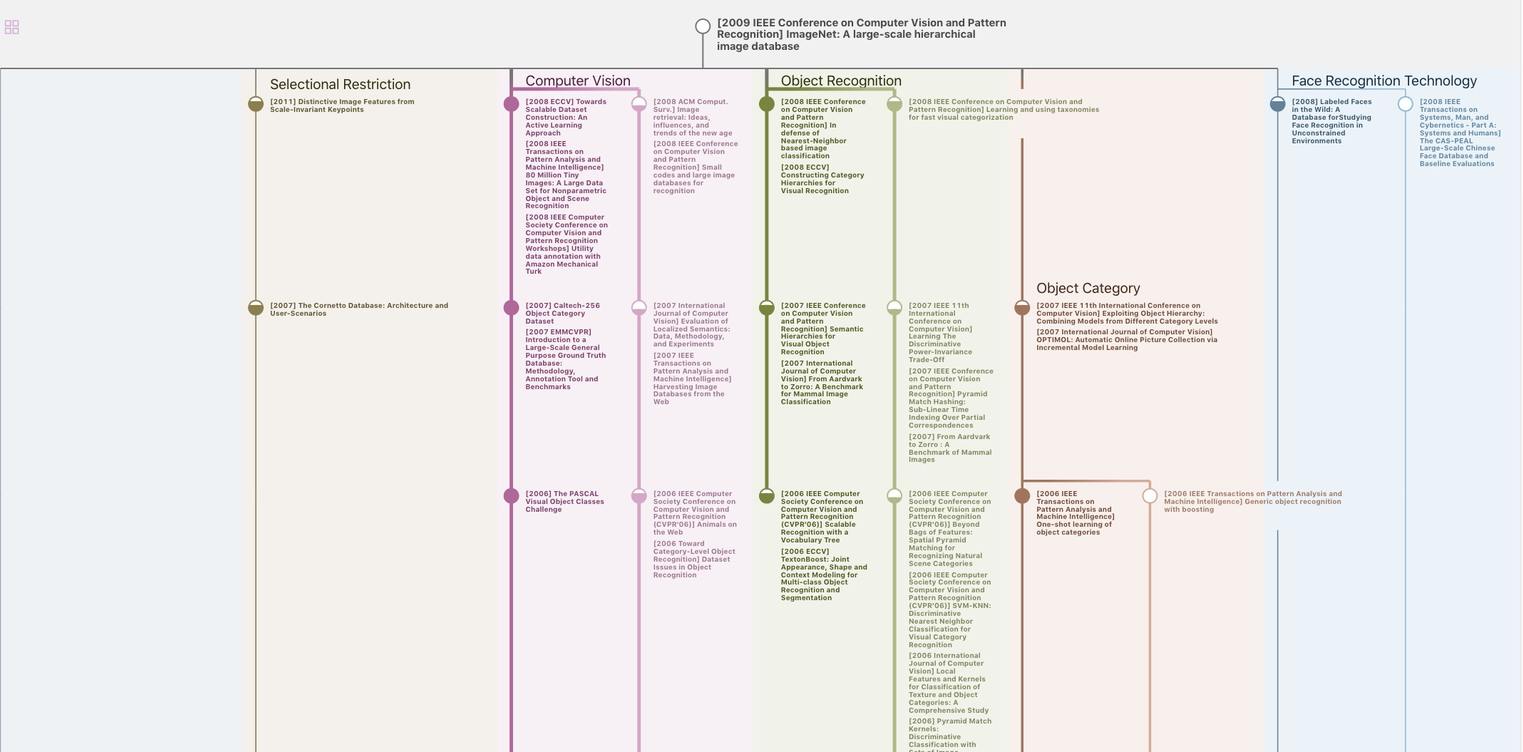
生成溯源树,研究论文发展脉络
Chat Paper
正在生成论文摘要