Unsupervised Learning of Local Equivariant Descriptors for Point Clouds
IEEE Transactions on Pattern Analysis and Machine Intelligence(2022)
摘要
Correspondences between 3D keypoints generated by matching local descriptors are a key step in 3D computer vision and graphic applications. Learned descriptors are rapidly evolving and outperforming the classical handcrafted approaches in the field. Yet, to learn effective representations they require supervision through labeled data, which are cumbersome and time-consuming to obtain. Unsupervised alternatives exist, but they lag in performance. Moreover, invariance to viewpoint changes is attained either by relying on data augmentation, which is prone to degrading upon generalization on unseen datasets, or by learning from handcrafted representations of the input which are already rotation invariant but whose effectiveness at training time may significantly affect the learned descriptor. We show how learning an
equivariant
3D local descriptor instead of an invariant one can overcome both issues. LEAD (Local EquivAriant Descriptor) combines Spherical CNNs to learn an equivariant representation together with plane-folding decoders to learn without supervision. Through extensive experiments on standard surface registration datasets, we show how our proposal outperforms existing unsupervised methods by a large margin and achieves competitive results against the supervised approaches, especially in the practically very relevant scenario of transfer learning.
更多查看译文
关键词
Deep learning on point clouds,local features,equivariant,unsupervised,feature learning,registration
AI 理解论文
溯源树
样例
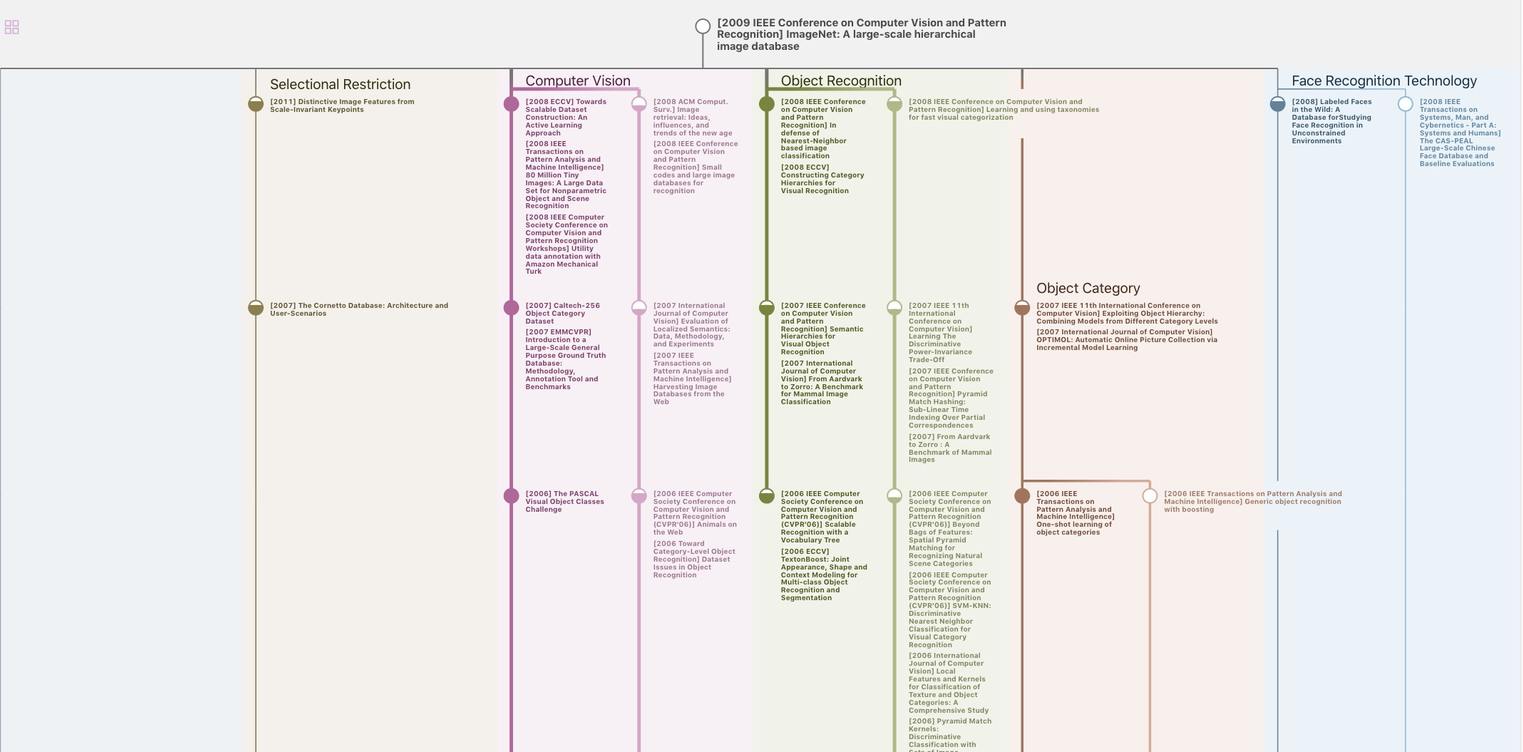
生成溯源树,研究论文发展脉络
Chat Paper
正在生成论文摘要