Nonlinear fault detection for batch processes via improved chordal kernel tensor locality preserving projections
Control Engineering Practice(2020)
摘要
The quality and stability of products are seriously influenced by the process conditions. A large number of modern production processes can be considered as batch processes, with nonlinear relationships between the process variables. How to troubleshoot batch processes has attracted considerable attention in the literature. The research object of batch processes is expressed as the third-order tensor data of batch × variable × time. The traditional methods convert the tensors into second-order forms through matrix expansion. A novel method named improved chordal kernel tensor locality preserving projections (ICK-TLPP) is proposed for fault detection of batch processes. First, the chordal distance is introduced as a measurement of the similarity of matrix, and an improved method is proposed for describing the variation of time series data. Then, the chordal kernel function is introduced to preserve the spatial structure of the tensor data without the information loss caused by vectorization, and describe the nonlinear correlation during the multivariate control system. Next, the locality preserving projections algorithm is applied to detect the intrinsic manifold structure. Parallel analysis is applied to optimize the hyper-parameters in the model. Finally, Granger causality analysis is performed to locate the root cause of the process fault. The proposed method is validated on two datasets, penicillin fermentation process and the hot strip rolling process. The best results of false alarm rate and fault detection rate are 16% and 94% respectively. The proposed method performs better compared with the traditional algorithms.
更多查看译文
关键词
Batch processes,Fault detection,Tensor space,Chordal kernel,Locality preserving projections,Parallel analysis
AI 理解论文
溯源树
样例
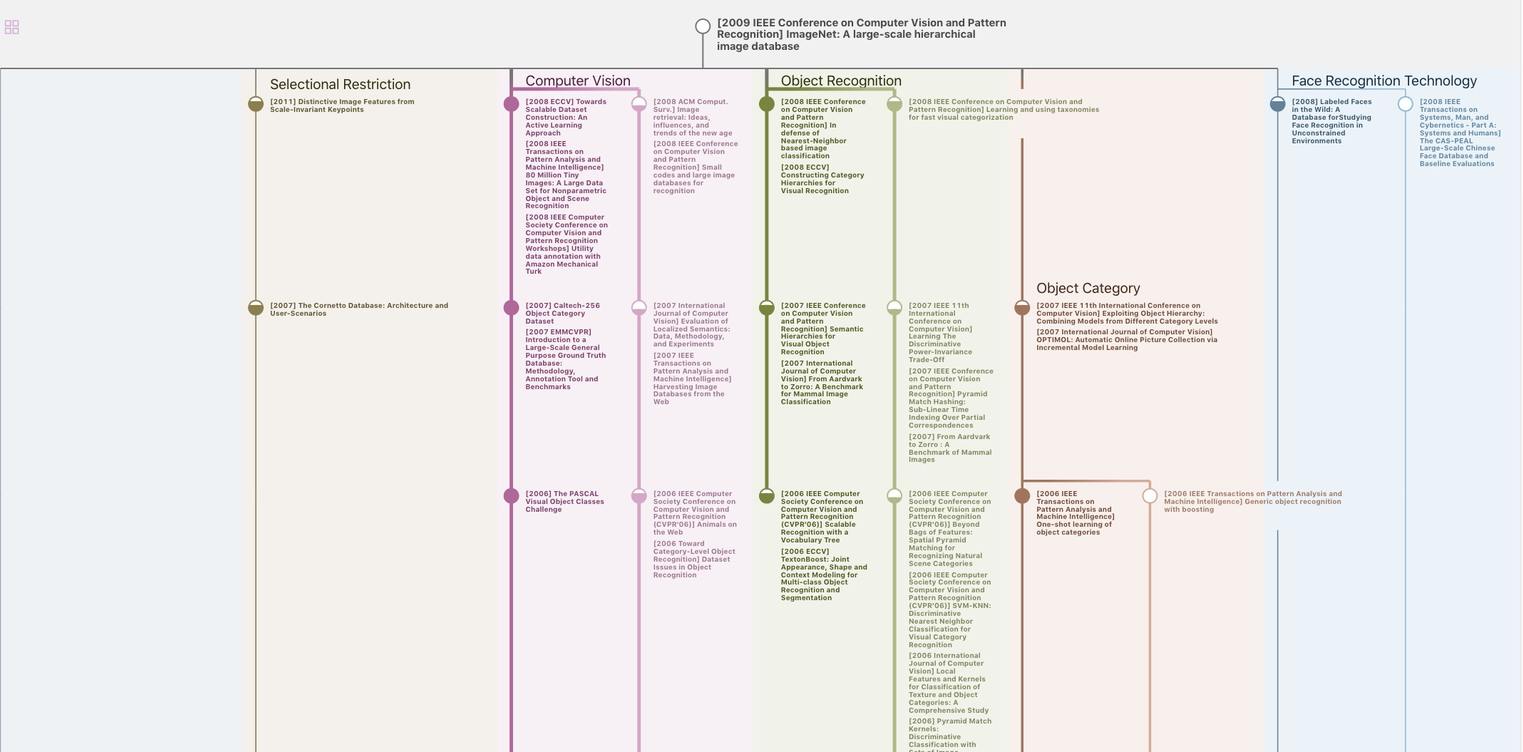
生成溯源树,研究论文发展脉络
Chat Paper
正在生成论文摘要