GFST - Gender-Filtered Self-Training for More Accurate Gender in Translation.
EMNLP(2021)
摘要
Targeted evaluations have found that machine translation systems often output incorrect gender in translations, even when the gender is clear from context. Furthermore, these incorrectly gendered translations have the potential to reflect or amplify social biases. We propose gender-filtered self-training (GFST) to improve gender translation accuracy on unambiguously gendered inputs. Our GFST approach uses a source monolingual corpus and an initial model to generate gender-specific pseudo-parallel corpora which are then filtered and added to the training data. We evaluate GFST on translation from English into five languages, finding that it improves gender accuracy without damaging generic quality. We also show the viability of GFST on several experimental settings, including re-training from scratch, fine-tuning, controlling the gender balance of the data, forward translation, and back-translation.
更多查看译文
关键词
more accurate gender-filtered,translation,self-training
AI 理解论文
溯源树
样例
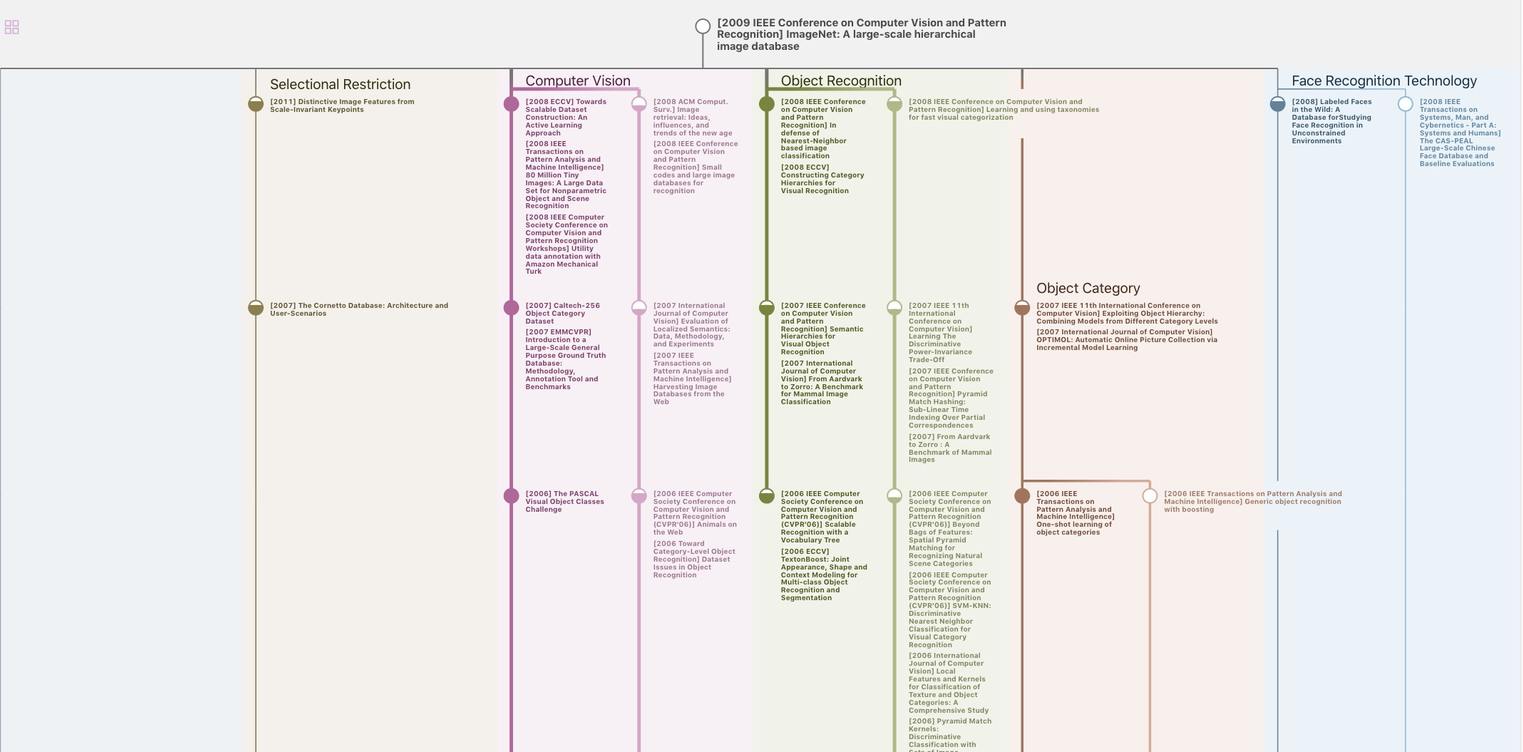
生成溯源树,研究论文发展脉络
Chat Paper
正在生成论文摘要