Effective Convolutional Attention Network for Multi-label Clinical Document Classification.
EMNLP(2021)
摘要
Multi-label document classification (MLDC) problems can be challenging, especially for long documents with a large label set and a long-tail distribution over labels. In this paper, we present an effective convolutional attention network for the MLDC problem with a focus on medical code prediction from clinical documents. Our innovations are three-fold: (1) we utilize a deep convolution-based encoder with the squeeze-and-excitation networks and residual networks to aggregate the information across the document and learn meaningful document representations that cover different ranges of texts; (2) we explore multilayer and sum-pooling attention to extract the most informative features from these multiscale representations; (3) we combine binary cross entropy loss and focal loss to improve performance for rare labels. We focus our evaluation study on MIMIC-III, a widely used dataset in the medical domain Our models outperform prior work on medical coding and achieve new state-of-the-art results on multiple metrics. We also demonstrate the language independent nature of our approach by applying it to two non-English datasets. Our model outperforms prior best model and a multilingual Transformer model by a substantial margin.
更多查看译文
AI 理解论文
溯源树
样例
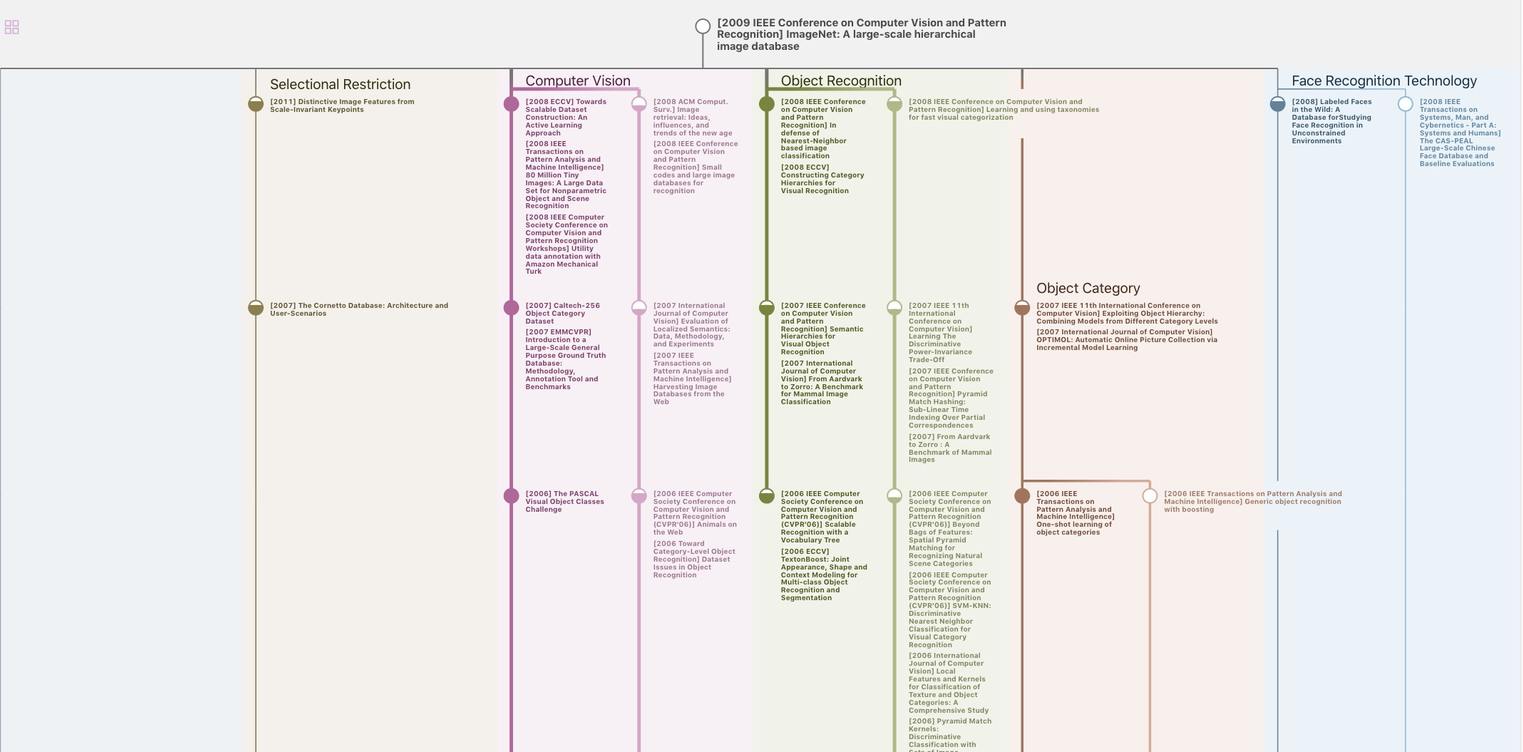
生成溯源树,研究论文发展脉络
Chat Paper
正在生成论文摘要