Linear convergence of the Douglas-Rachford algorithm via a generic error bound condition
arxiv(2021)
摘要
We provide new insight into the convergence properties of the Douglas-Rachford algorithm for the problem $\min_x \{f(x)+g(x)\}$, where $f$ and $g$ are convex functions. Our approach relies on and highlights the natural primal-dual symmetry between the above problem and its Fenchel dual $\min_{u} \{ f^*(u) + g_*(u)\}$ where $g_*(u):=g^*(-u)$. Our main development is to show the linear convergence of the algorithm when a natural error bound condition on the Douglas-Rachford operator holds. We leverage our error bound condition approach to show and estimate the algorithm's linear rate of convergence for three special classes of problems. The first one is when $f$ or$g$ and $f^*$ or $g_*$ are strongly convex relative to the primal and dual optimal sets respectively. The second one is when~$f$ and~$g$ are piecewise linear-quadratic functions. The third one is when~$f$ and~$g$ are the indicator functions of closed convex cones. In all three cases the rate of convergence is determined by a suitable measure of well-posedness of the problem. In the conic case, if the two closed convex cones are a linear subspace $L$ and $\mathbb{R}^n_+$, we establish the following stronger {\em finite termination} result: the Douglas-Rachford algorithm identifies the {\em maximum support sets} for $L\cap \mathbb{R}^n_+$ and $L^{\perp}\cap\mathbb{R}^n_+$ in finitely many steps. Our developments have straightforward extensions to the more general linearly constrained problem $\min_{x,y} \{f(x) + g( y):Ax + By = b\}$ thereby highlighting a direct and straightforward relationship between the Douglas-Rachford algorithm and the alternating direction method of multipliers (ADMM).
更多查看译文
关键词
linear convergence,algorithm,douglas-rachford
AI 理解论文
溯源树
样例
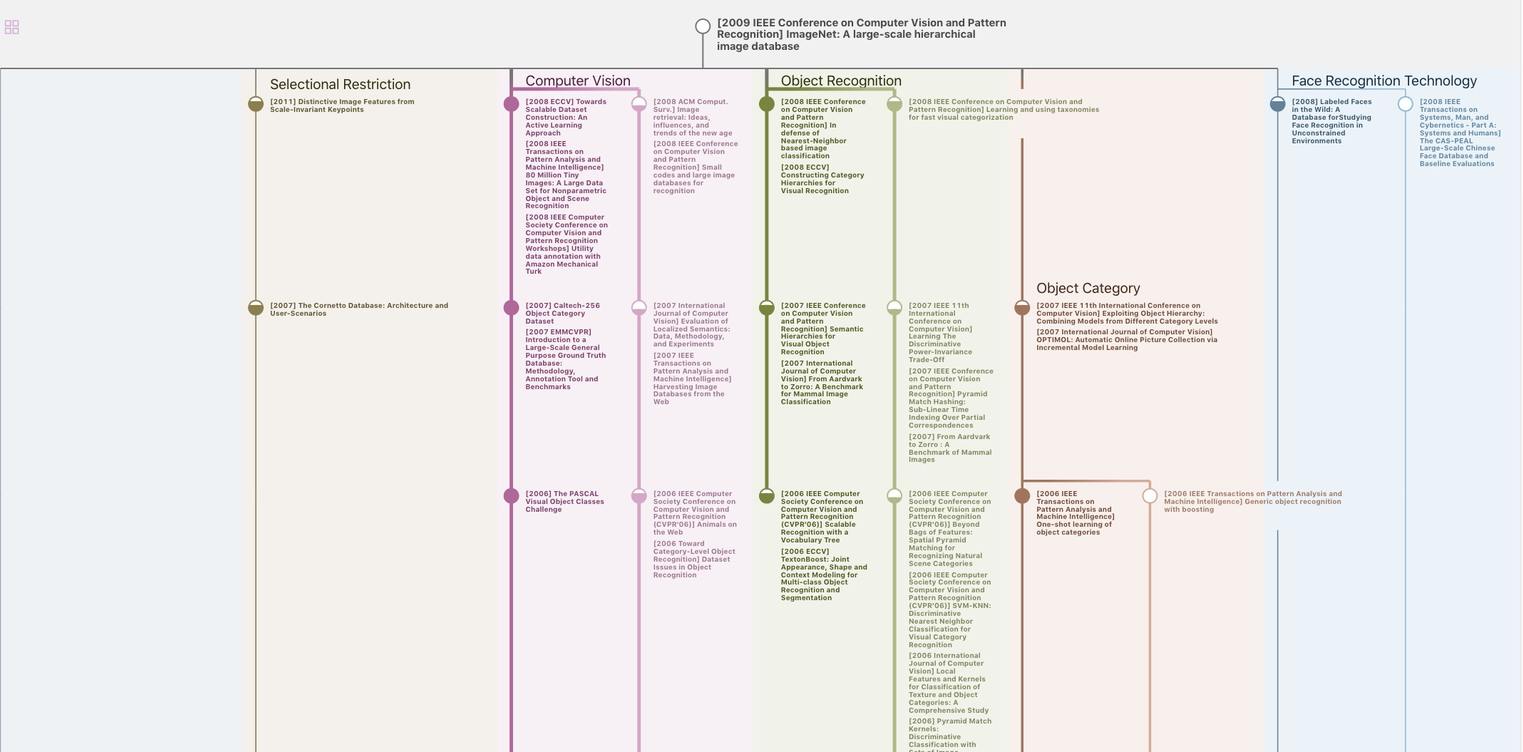
生成溯源树,研究论文发展脉络
Chat Paper
正在生成论文摘要