Reinforcement Learning for Mobile Robotics Exploration: A Survey
IEEE transactions on neural networks and learning systems(2023)
摘要
Efficient exploration of unknown environments is a fundamental precondition for modern autonomous mobile robot applications. Aiming to design robust and effective robotic exploration strategies, suitable to complex real-world scenarios, the academic community has increasingly investigated the integration of robotics with reinforcement learning (RL) techniques. This survey provides a comprehensive review of recent research works that use RL to design unknown environment exploration strategies for single and multirobots. The primary purpose of this study is to facilitate future research by compiling and analyzing the current state of works that link these two knowledge domains. This survey summarizes: what are the employed RL algorithms and how they compose the so far proposed mobile robot exploration strategies; how robotic exploration solutions are addressing typical RL problems like the exploration-exploitation dilemma, the curse of dimensionality, reward shaping, and slow learning convergence; and what are the performed experiments and software tools used for learning and testing. Achieved progress is described, and a discussion about remaining limitations and future perspectives is presented.
更多查看译文
关键词
Robots,Planning,Mathematical models,Computational modeling,Numerical models,Navigation,Electrical engineering,Cooperative exploration,mobile robot exploration,multirobot exploration,multirobot systems (MRSs),reinforcement learning (RL),single robot exploration
AI 理解论文
溯源树
样例
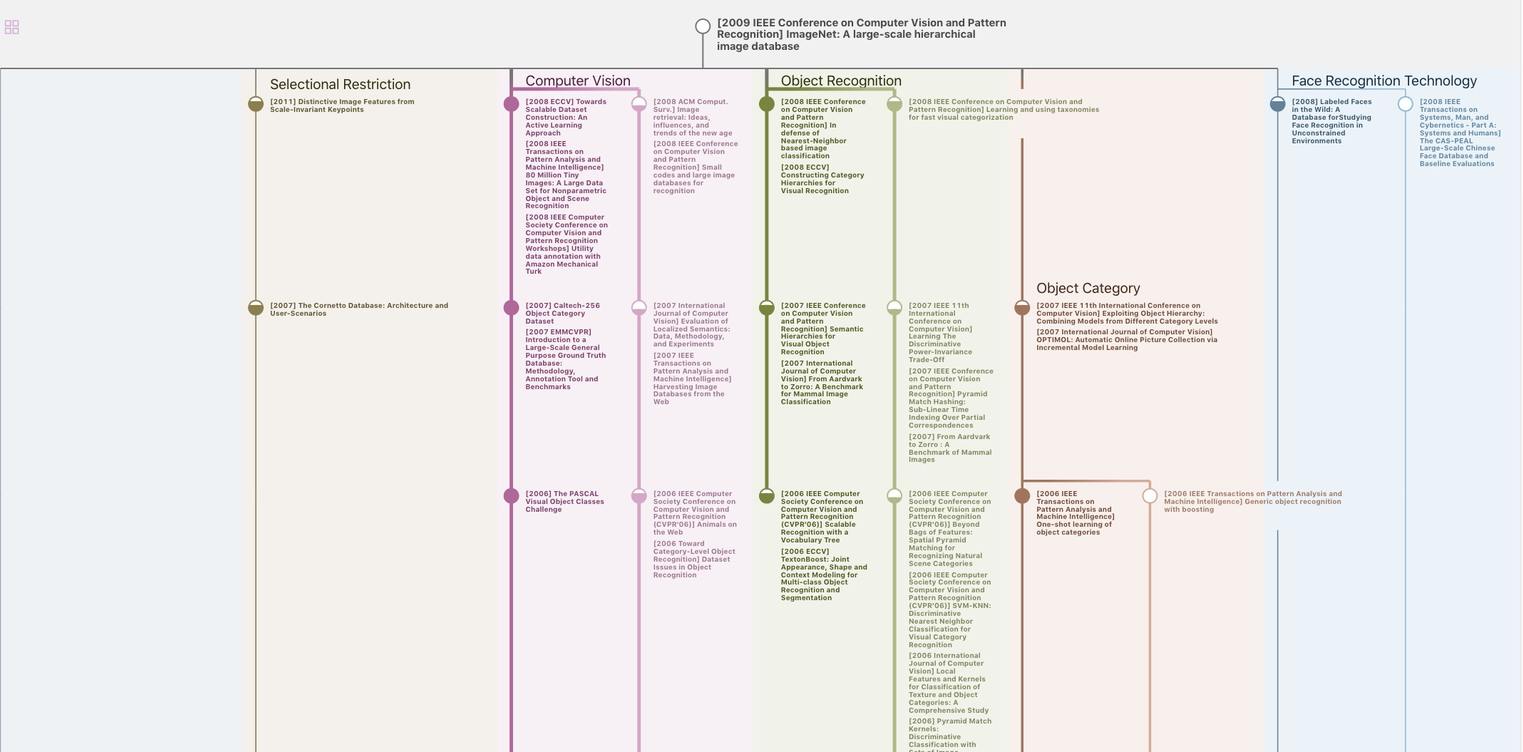
生成溯源树,研究论文发展脉络
Chat Paper
正在生成论文摘要