Improved Accuracy in Predicting the Best Sensor Fusion Architecture for Multiple Domains
SENSORS(2021)
摘要
Multi-sensor fusion intends to boost the general reliability of a decision-making procedure or allow one sensor to compensate for others' shortcomings. This field has been so prominent that authors have proposed many different fusion approaches, or "architectures " as we call them when they are structurally different, so it is now challenging to prescribe which one is better for a specific collection of sensors and a particular application environment, other than by trial and error. We propose an approach capable of predicting the best fusion architecture (from predefined options) for a given dataset. This method involves the construction of a meta-dataset where statistical characteristics from the original dataset are extracted. One challenge is that each dataset has a different number of variables (columns). Previous work took the principal component analysis's first k components to make the meta-dataset columns coherent and trained machine learning classifiers to predict the best fusion architecture. In this paper, we take a new route to build the meta-dataset. We use the Sequential Forward Floating Selection algorithm and a T transform to reduce the features and match them to a given number, respectively. Our findings indicate that our proposed method could improve the accuracy in predicting the best sensor fusion architecture for multiple domains.
更多查看译文
关键词
sensor fusion, classification, SFFS, metadata, statistical signature
AI 理解论文
溯源树
样例
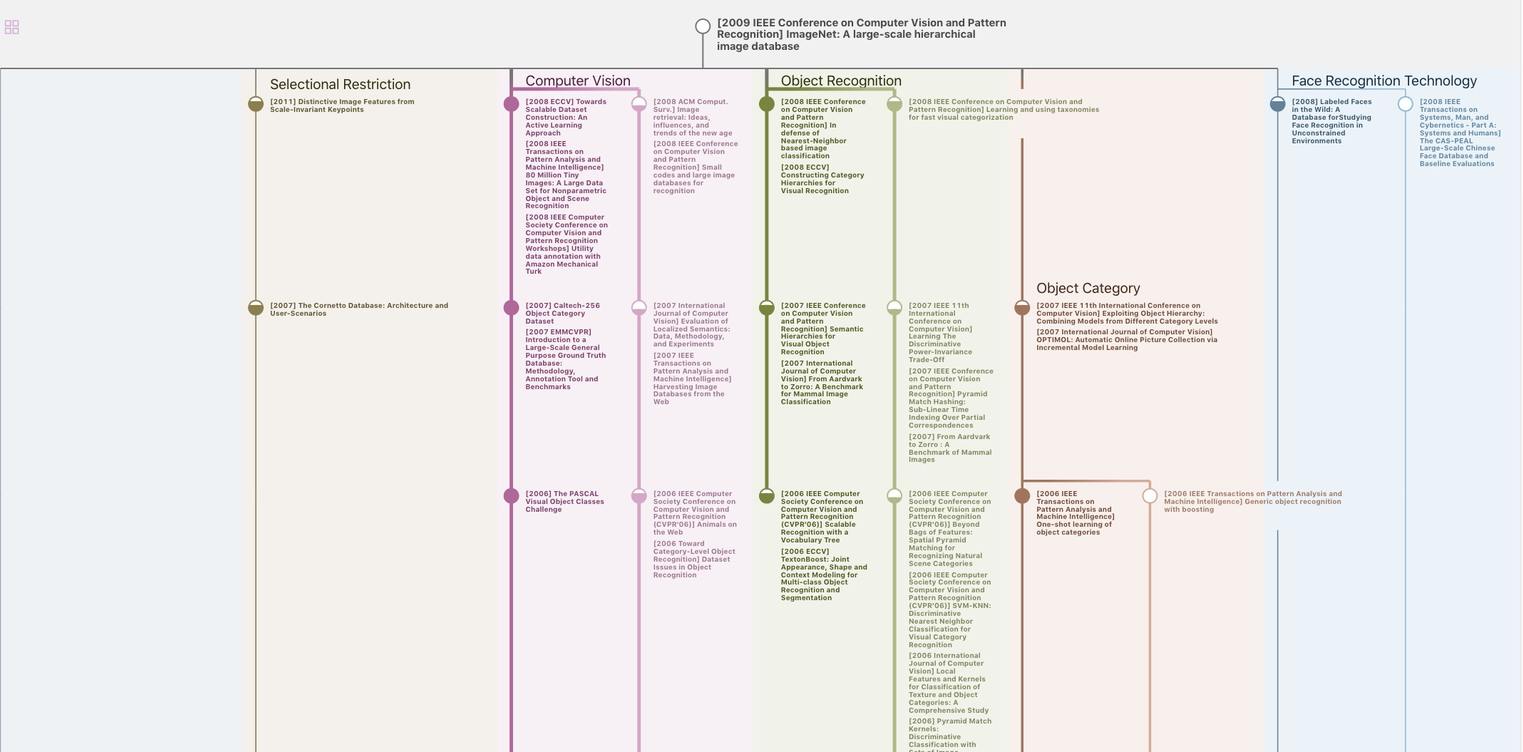
生成溯源树,研究论文发展脉络
Chat Paper
正在生成论文摘要