Soft Sensing Model Visualization - Fine-tuning Neural Network from What Model Learned.
IEEE BigData(2021)
摘要
The growing availability of the data collected from smart manufacturing is changing the paradigms of production monitoring and control. The increasing complexity and content of the wafer manufacturing process in addition to the time-varying unexpected disturbances and uncertainties, make it infeasible to do the control process with model-based approaches. As a result, data-driven soft-sensing modeling has become more prevalent in wafer process diagnostics. Recently, deep learning has been utilized in soft sensing system with promising performance on highly nonlinear and dynamic time-series data. Despite its successes in soft-sensing systems, however, the underlying logic of the deep learning framework is hard to understand. In this paper, we propose a deep learning-based model for defective wafer detection using a highly imbalanced dataset. To understand how the proposed model works, the deep visualization approach is applied. Additionally, the model is then fine-tuned guided by the deep visualization. Extensive experiments are performed to validate the effectiveness of the proposed system. The results provide an interpretation of how the model works and an instructive fine-tuning method based on the interpretation.
更多查看译文
关键词
Deep Learning,Deep Visualization,Defective wafer detection
AI 理解论文
溯源树
样例
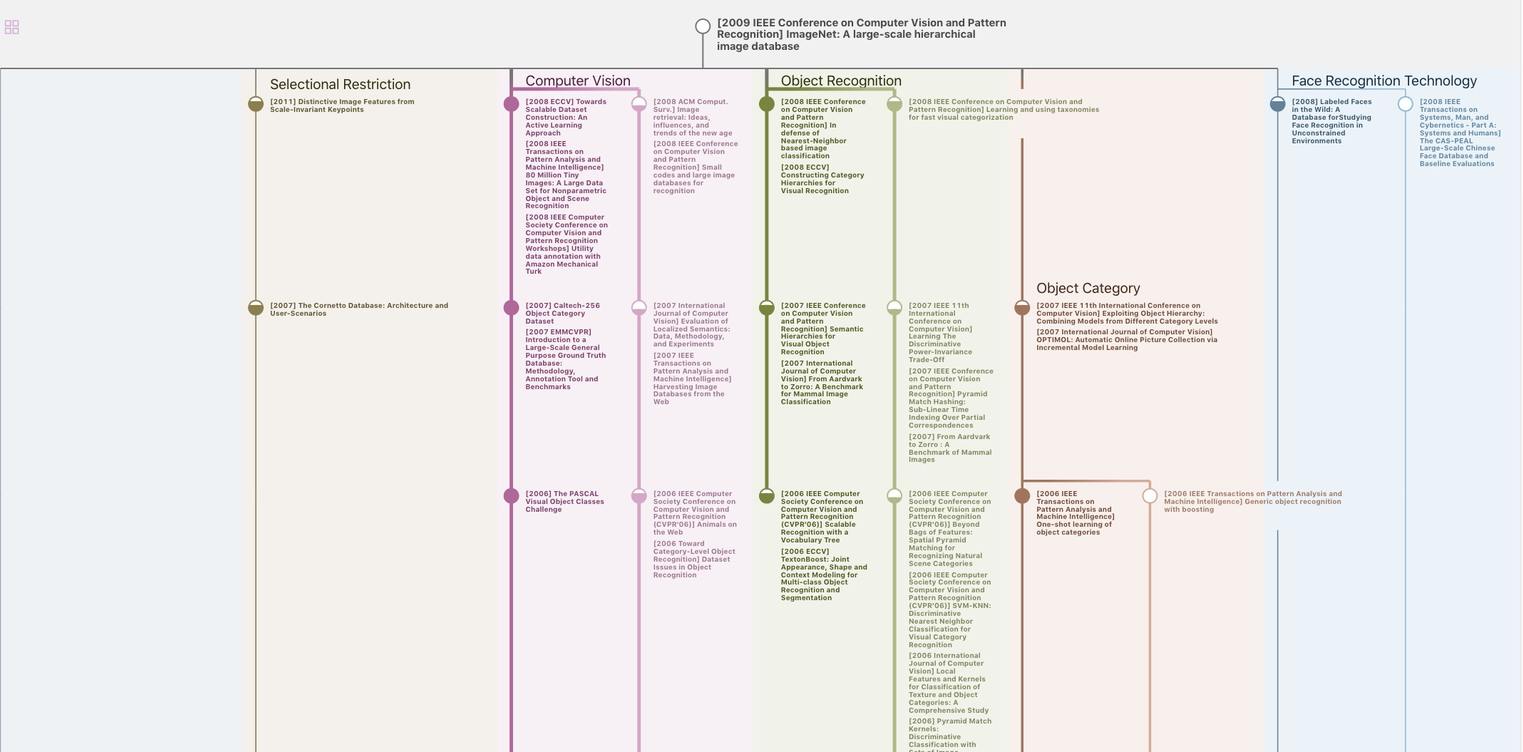
生成溯源树,研究论文发展脉络
Chat Paper
正在生成论文摘要