When Can We Ignore Measurement Error in the Running Variable?
arxiv(2023)
摘要
In many applications of regression discontinuity designs, the running variable used by the administrator to assign treatment is only observed with error. We show that, provided the observed running variable (i) correctly classifies the treatment assignment, and (ii) affects the conditional means of the potential outcomes smoothly, ignoring the measurement error nonetheless yields an estimate with a causal interpretation: the average treatment effect for units whose observed running variable equals to the cutoff. We show that, possibly after doughnut trimming, these assumptions accommodate a variety of settings where support of the measurement error is not too wide. We propose to conduct inference using bias-aware methods, which remain valid even when discreteness or irregular support in the observed running variable may lead to partial identification. We illustrate the results for both sharp and fuzzy designs in an empirical application.
更多查看译文
关键词
bias-aware inference,measurement error,regression discontinuity
AI 理解论文
溯源树
样例
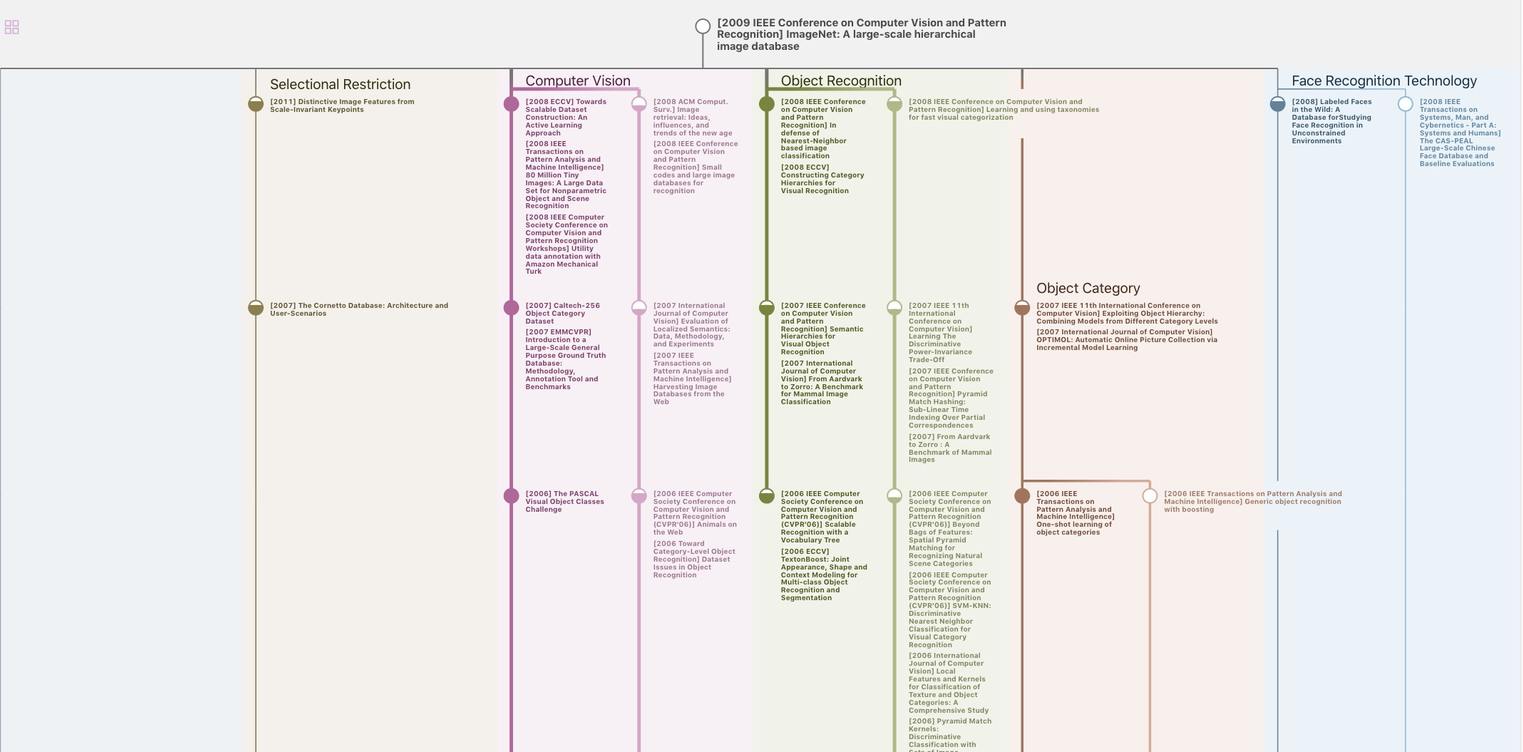
生成溯源树,研究论文发展脉络
Chat Paper
正在生成论文摘要