Bag of groups of convolutional features model for visual object recognition
2021 IEEE 31ST INTERNATIONAL WORKSHOP ON MACHINE LEARNING FOR SIGNAL PROCESSING (MLSP)(2021)
摘要
Deep convolutional neural networks (CNNs) are only equivariant to translation. Recently, equivariant CNNs are proposed for the task of image classification which are not only equivariant to translation but also to other affine geometric transformations. Moreover, CNNs and equivariant CNNs require a large amount of labeled training data to generalize its parameters which also limit their application areas. We propose a bag of groups of convolutional features (BoGCFs) model for the CNNs and group-equivariant CNNs (G-CNNs)[1], which preserves the fundamental property of equivariance of G-CNNs and generate the global invariant features by dividing the convolutional feature maps of the deeper layers of the network into groups. The proposed model for CNNs and G-CNNs, referred as CNN-BoGCFs and G-CNN-BoGCFs, performs significantly high when trained on a small amount of labeled data for image classification. The proposed method is evaluated using rotated MNIST, SIMPLIcity and Oxford flower 17 datasets.
更多查看译文
关键词
Rotation,equivariance,invariance,classification
AI 理解论文
溯源树
样例
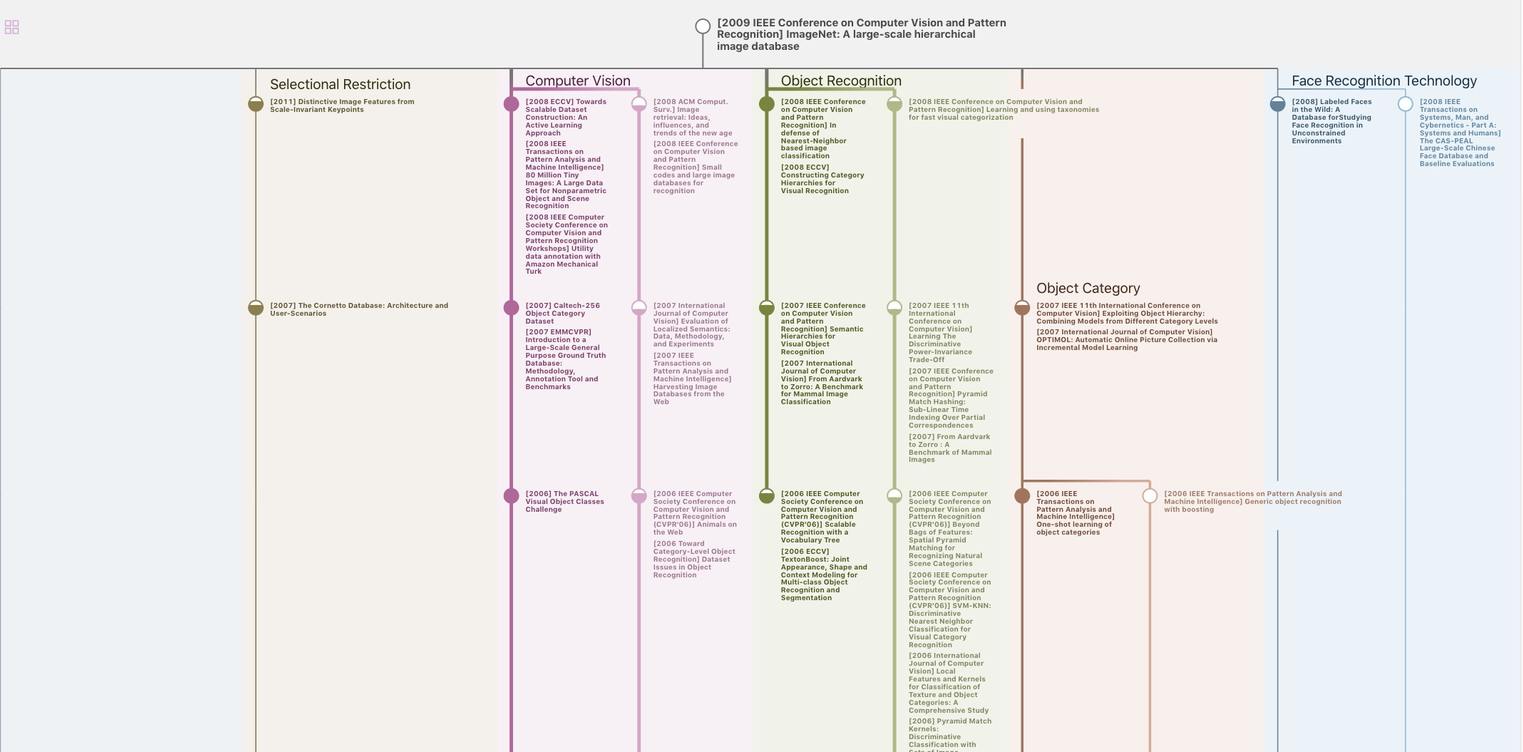
生成溯源树,研究论文发展脉络
Chat Paper
正在生成论文摘要