Convergence of Anisotropic Consensus-Based Optimization in Mean-Field Law.
EvoStar Conferences (EvoStar)(2022)
摘要
In this paper we study anisotropic consensus-based optimization (CBO), a multi-agent metaheuristic derivative-free optimization method capable of globally minimizing nonconvex and nonsmooth functions in high dimensions. CBO is based on stochastic swarm intelligence, and inspired by consensus dynamics and opinion formation. Compared to other metaheuristic algorithms like particle swarm optimization, CBO is of a simpler nature and therefore more amenable to theoretical analysis. By adapting a recently established proof technique, we show that anisotropic CBO converges globally with a dimension-independent rate for a rich class of objective functions under minimal assumptions on the initialization of the method. Moreover, the proof technique reveals that CBO performs a convexification of the optimization problem as the number of agents goes to infinity, thus providing an insight into the internal CBO mechanisms responsible for the success of the method. To motivate anisotropic CBO from a practical perspective, we further test the method on a complicated high-dimensional benchmark problem, which is well understood in the machine learning literature.
更多查看译文
关键词
High-dimensional global optimization,Metaheuristics,Consensus-based optimization,Mean-field limit,Anisotropy
AI 理解论文
溯源树
样例
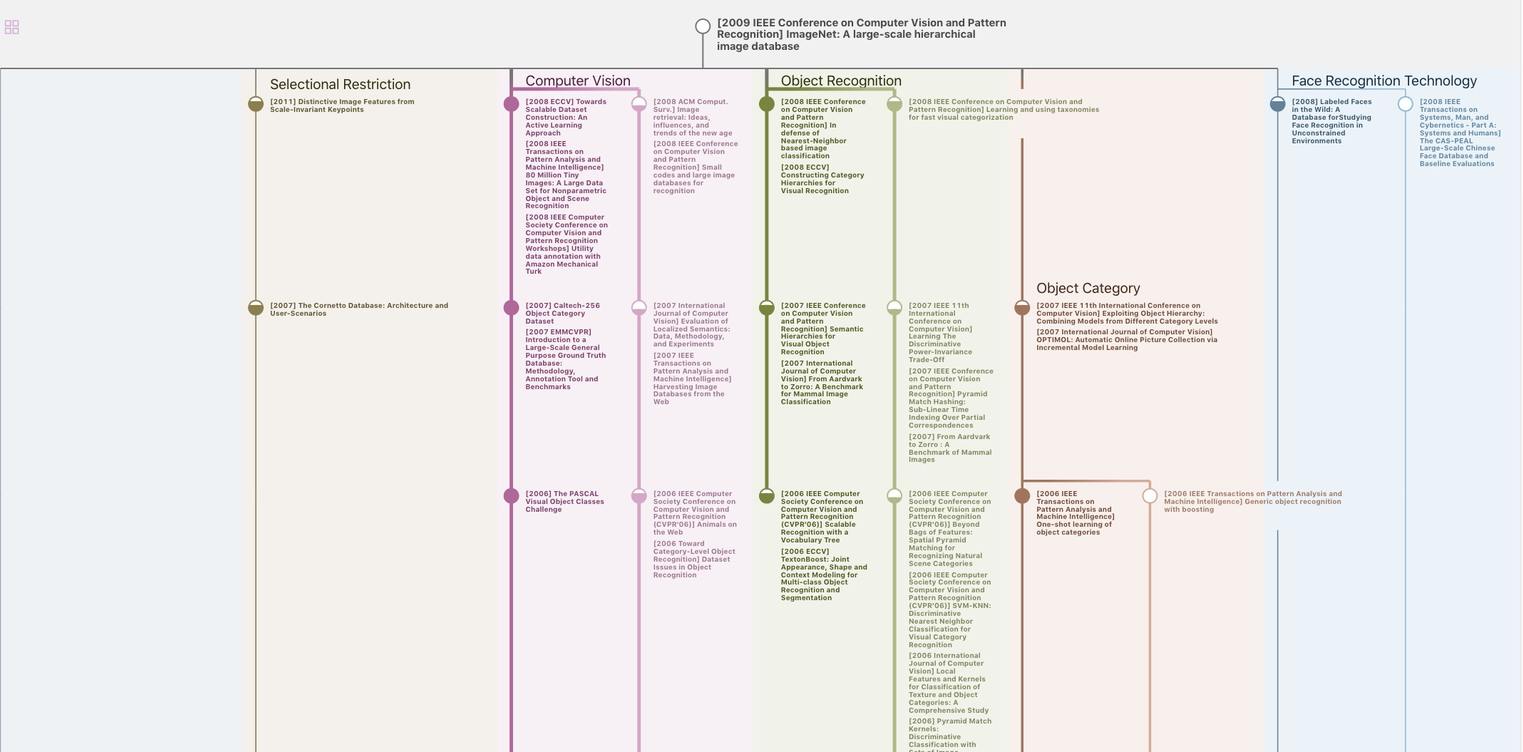
生成溯源树,研究论文发展脉络
Chat Paper
正在生成论文摘要