An Attention-Based Deep Learning Model with Interpretable Patch-Weight Sharing for Diagnosing Cervical Dysplasia.
IntelliSys (3)(2021)
摘要
Diagnosis of cervical dysplasia by visual inspection is a difficult problem. Most of the recent approaches use deep learning techniques to extract features and detect a region of interest (RoI) in the image. Such approaches can lead to loss of visual detail that appears weak and local within the cervical image. Also, it requires manual annotation to extract the RoI. Moreover, there are not many labeled data due to the medical image’s characteristics. To mitigate the problem, we present an approach that extracts global and local features in the image without manual annotation when there is a shortage of data. The proposed approach is applied to classify cervix cancer, and the results are demonstrated. First of all, we divide the cervix image into nine patches to extract visual features when high-resolution images are unavailable. Second, we generate a deep learning model sharing a weight between patches of the image by considering the patch-patch and patch-image relationship. We also apply an attention mechanism to the model to train the visual features of the image and show an interpretable result. Finally, we add a loss weighting inspired by the domain knowledge to the training process, which guides the learning better while preventing overfitting. The evaluation results indicate improvements over the state-of-the-art methods in sensitivity.
更多查看译文
关键词
Dysplasia,Deep learning,Machine learning,Medicine,Artificial intelligence
AI 理解论文
溯源树
样例
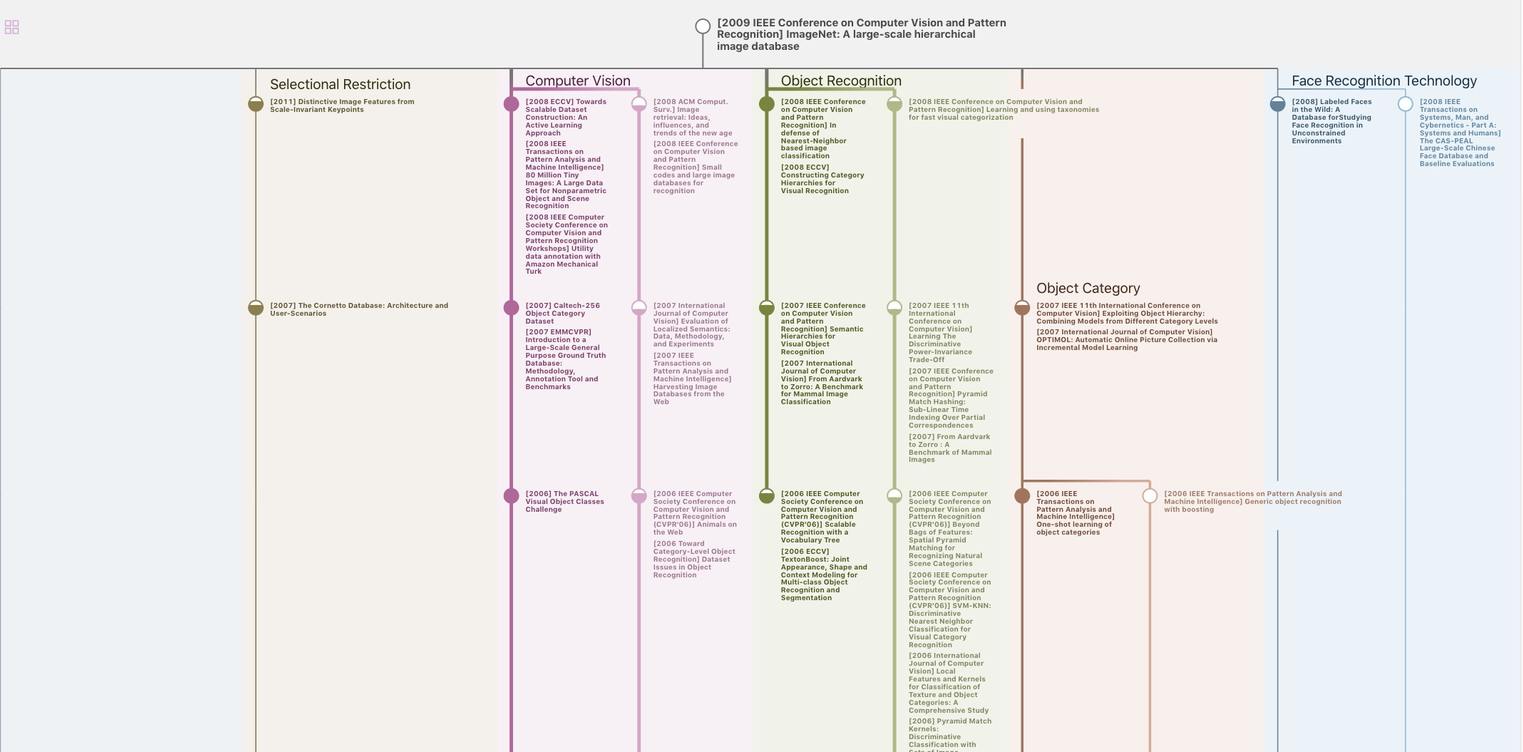
生成溯源树,研究论文发展脉络
Chat Paper
正在生成论文摘要