Double Ensemble Soft Transfer Network for Unsupervised Domain Adaptation.
DASFAA (2)(2021)
摘要
Domain adaptation aims to transfer the enriched label knowledge from large amounts of source data to unlabeled target data. Recent methods start to solve the class-wise domain adaptation problem by incorporating the soft labels to each target data. Although the soft label strategy could alleviate the negative influence caused by the hard label strategy to some extent, the improper propagation sequence ignoring the labeling difficulties of different target examples will lead to confusing probabilities problem. Moreover, the instability of a single propagation model in dealing with various data may also hinder the performance of target label inference. To address these limitations, we propose a Double Ensemble Soft Transfer Network (DESTN) to jointly optimize the class-wise adaptation and learn the discriminative domain-invariant features with clear soft target labels. Our motivation is to construct a Label Propagation Ensemble (LPE) model by various feature subspaces so as to get robust and clear soft target labels for class-wise domain adaptation. Meanwhile, the other Classifiers Ensemble Framework (CEF) is trained on the labeled source samples and the reliable pseudo-labeled target samples for learning the discriminative features during the iteration. Extensive experiments show that DESTN significantly outperforms several state-of-the-art methods.
更多查看译文
关键词
Unsupervised domain adaptation,Deep ensemble network,Soft labels,Discriminative feature learning
AI 理解论文
溯源树
样例
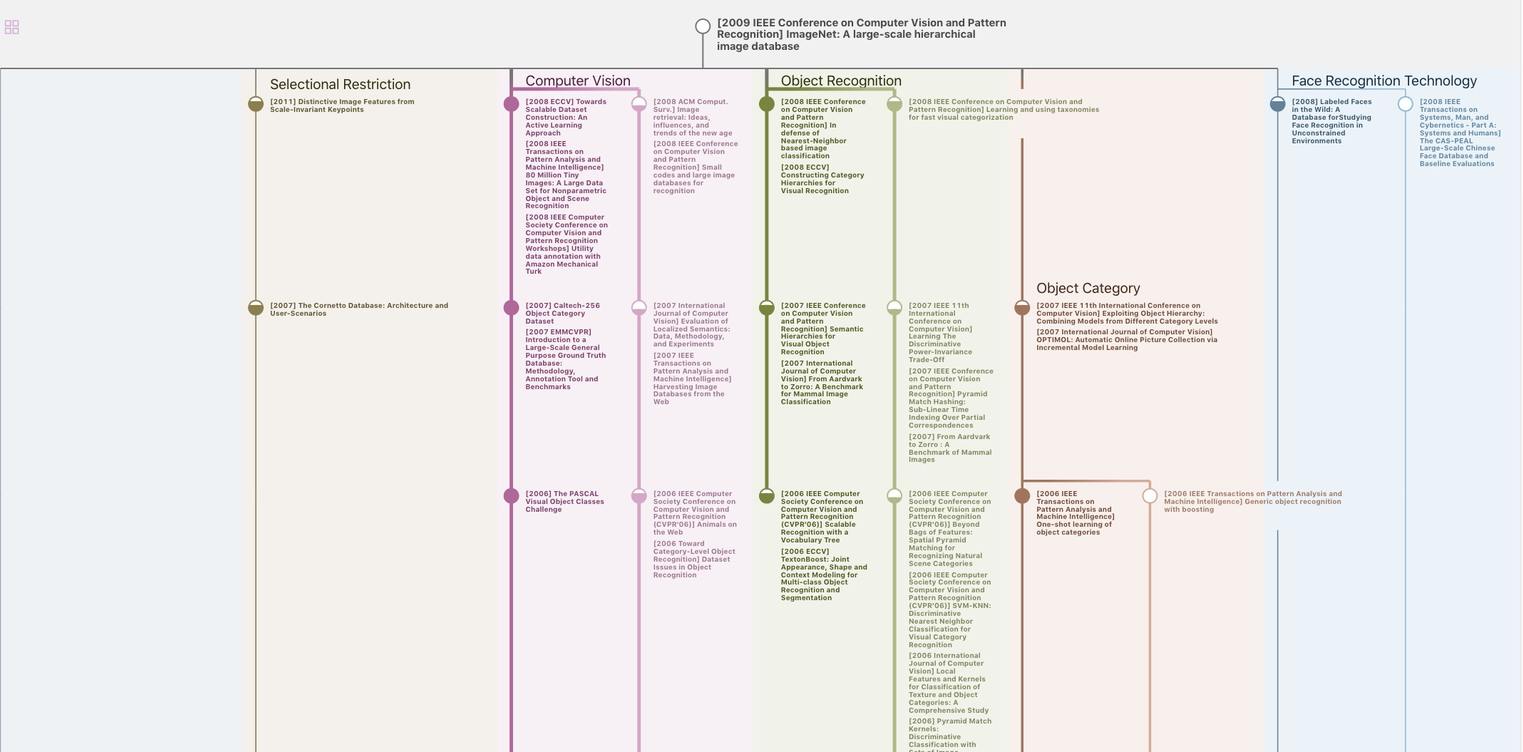
生成溯源树,研究论文发展脉络
Chat Paper
正在生成论文摘要