Contribution D’informations Syntaxiques Aux Capacités De Généralisation Compositionelle Des Modèles Seq2seq Convolutifs (assessing the Contribution of Syntactic Information for Compositional Generalization of Seq2seq Convolutional Networks)
TALN (1)(2021)
Abstract
Les modeles neuronaux de type seq2seq manifestent d’etonnantes capacites de prediction quand ils sont entraines sur des donnees de taille suffisante. Cependant, ils echouent a generaliser de maniere satisfaisante quand la tâche implique d’apprendre et de reutiliser des regles systematiques de composition et non d’apprendre simplement par imitation des exemples d’entrainement. Le jeu de donnees SCAN, constitue d’un ensemble de commandes en langage naturel associees a des sequences d’action, a ete specifiquement concu pour evaluer les capacites des reseaux de neurones a apprendre ce type de generalisation compositionnelle. Dans cet article, nous nous proposons d’etudier la contribution d’informations syntaxiques sur les capacites de generalisation compositionnelle des reseaux de neurones seq2seq convolutifs.
MoreTranslated text
求助PDF
上传PDF
View via Publisher
AI Read Science
AI Summary
AI Summary is the key point extracted automatically understanding the full text of the paper, including the background, methods, results, conclusions, icons and other key content, so that you can get the outline of the paper at a glance.
Example
Background
Key content
Introduction
Methods
Results
Related work
Fund
Key content
- Pretraining has recently greatly promoted the development of natural language processing (NLP)
- We show that M6 outperforms the baselines in multimodal downstream tasks, and the large M6 with 10 parameters can reach a better performance
- We propose a method called M6 that is able to process information of multiple modalities and perform both single-modal and cross-modal understanding and generation
- The model is scaled to large model with 10 billion parameters with sophisticated deployment, and the 10 -parameter M6-large is the largest pretrained model in Chinese
- Experimental results show that our proposed M6 outperforms the baseline in a number of downstream tasks concerning both single modality and multiple modalities We will continue the pretraining of extremely large models by increasing data to explore the limit of its performance
Upload PDF to Generate Summary
Must-Reading Tree
Example
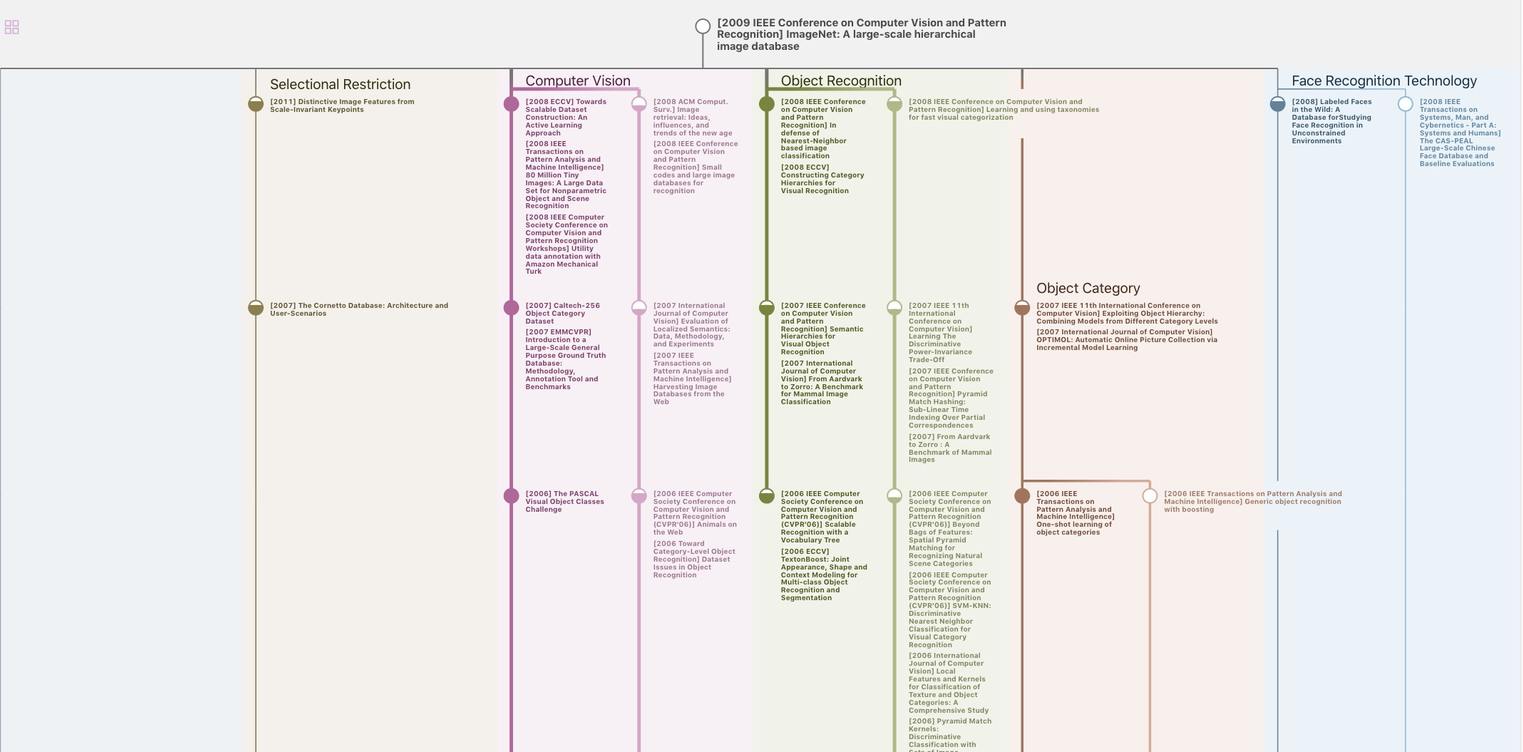
Generate MRT to find the research sequence of this paper
Data Disclaimer
The page data are from open Internet sources, cooperative publishers and automatic analysis results through AI technology. We do not make any commitments and guarantees for the validity, accuracy, correctness, reliability, completeness and timeliness of the page data. If you have any questions, please contact us by email: report@aminer.cn
Chat Paper
GPU is busy, summary generation fails
Rerequest