Classifying Functional Data from Orthogonal Projections - Model, Properties and Fast Implementation.
ICCS (3)(2021)
摘要
We consider the problem of functional, random data classification from equidistant samples. Such data are frequently not easy for classification when one has a large number of observations that bear low information for classification. We consider this problem using tools from the functional analysis. Therefore, a mathematical model of such data is proposed and its correctness is verified. Then, it is shown that any finite number of descriptors, obtained by orthogonal projections on any differentiable basis of L 2 ( 0 , T ) , can be consistently estimated within this model. Computational aspects of estimating descriptors, based on the fast implementation of the discrete cosine transform (DCT), are also investigated in conjunction with learning a classifier and using it on-line. Finally, the algorithm of learning descriptors and classifiers were tested on real-life random signals, namely, on accelerations, coming from large bucket-wheel excavators, that are transmitted to an operator’s cabin. The aim of these tests was also to select a classifier that is well suited for working with DCT-based descriptors.
更多查看译文
AI 理解论文
溯源树
样例
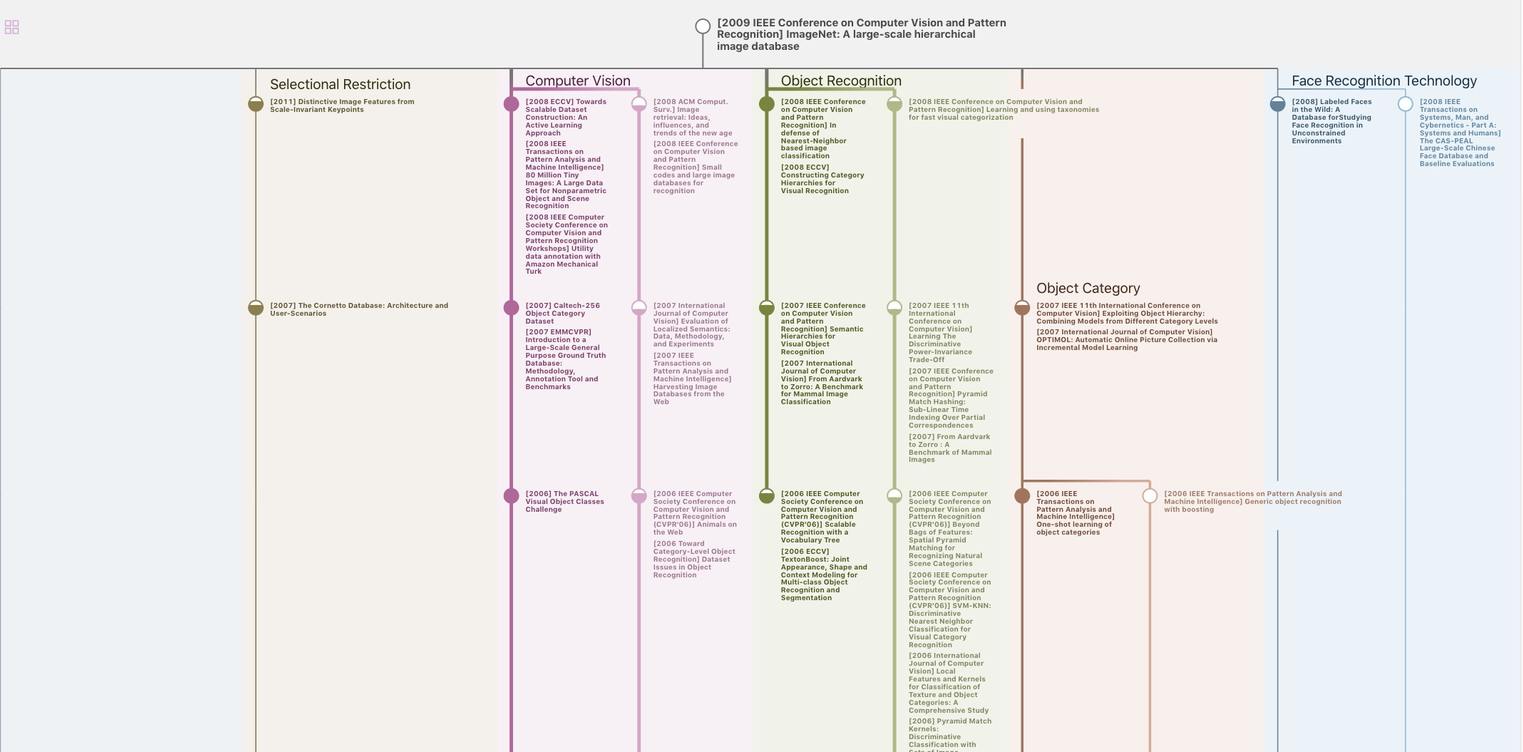
生成溯源树,研究论文发展脉络
Chat Paper
正在生成论文摘要