Decomposition-and-Fusion Network for HE-Stained Pathological Image Classification.
ICIC (3)(2021)
摘要
Building upon the clinical evidence supporting that decomposing a pathological image into different components can improve diagnostic value, in this paper we propose a Decomposition-and-Fusion Network (DFNet) for HE-stained pathological image classification. The medical goal of using HE-stained pathological images is to distinguish between nucleus, cytoplasm and extracellular matrix, thereby displaying the overall layouts of cells and tissues. We embed this most basic medical knowledge into a deep learning framework that decomposes a pathological image into cell nuclei and the remaining structures (that is, cytoplasm and extracellular matrix). With such decomposed pathological images, DFNet first extracts independent features using three independent CNN branches, and then gradually merges these features together for final classification. In this way, DFNet is able to learn more representative features with respect to different structures and hence improve the classification performance. Experimental results on two different datasets with various cancer types show that the DFNet achieves competitive performance.
更多查看译文
关键词
pathological image classification,decomposition-and-fusion,he-stained
AI 理解论文
溯源树
样例
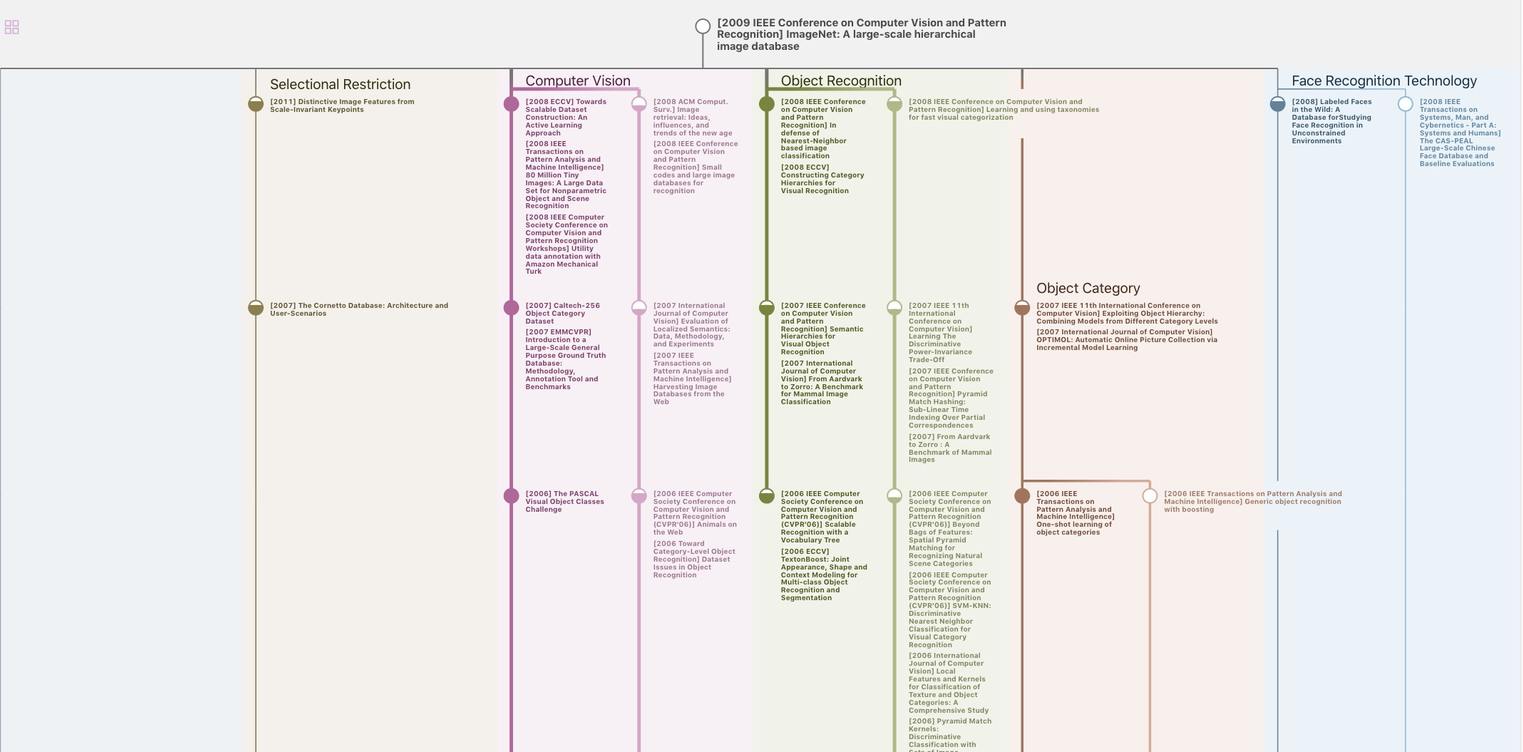
生成溯源树,研究论文发展脉络
Chat Paper
正在生成论文摘要