Toward the Minimum Number of Wearables to Recognize Signer-Independent Italian Sign Language with Machine-Learning Algorithms
IEEE transactions on instrumentation and measurement(2021)
摘要
Sign languages (SLs) can aid in improving the communication between hearing impaired and hearing communities, but only a limited number of hearing individuals understand it. SL recognition systems tackle this issue by using sensors to acquire gesture data later converted into spoken or written language by means of machine-learning algorithms. Within this frame, we propose a wearable electronic-based system for achieving automatic signer-independent recognition of Italian SL. The system is used for recognizing ten signs performed by 17 inexperienced signers. The wearable electronics measure hand, arm, and forearm gestures, by means of a sensory glove (equipped with ten flex sensors) and six inertial measurement units (IMUs). We adopt three classifiers: artificial neural network (ANN), k-nearest neighbors, and support vector machine, with ANN outperforming the others with an accuracy of 95.07%. However, the real key and novel aspect of this work concerns exploring the importance of each sensor for the overall classification accuracy, with the aim of determining the minimum number of sensors (and so minimizing the complexity of the wearable system) necessary to guarantee an acceptable accuracy. As a result, we could drop out three of the six IMUs, obtaining an accuracy as high as 93.91%. In addition, this valuable result was obtained using a signer-independent approach, which makes the application closer to a real-life situation.
更多查看译文
关键词
Flex sensors,inertial measurement unit (IMU),machine learning,sensory glove,sign language (SL) recognition
AI 理解论文
溯源树
样例
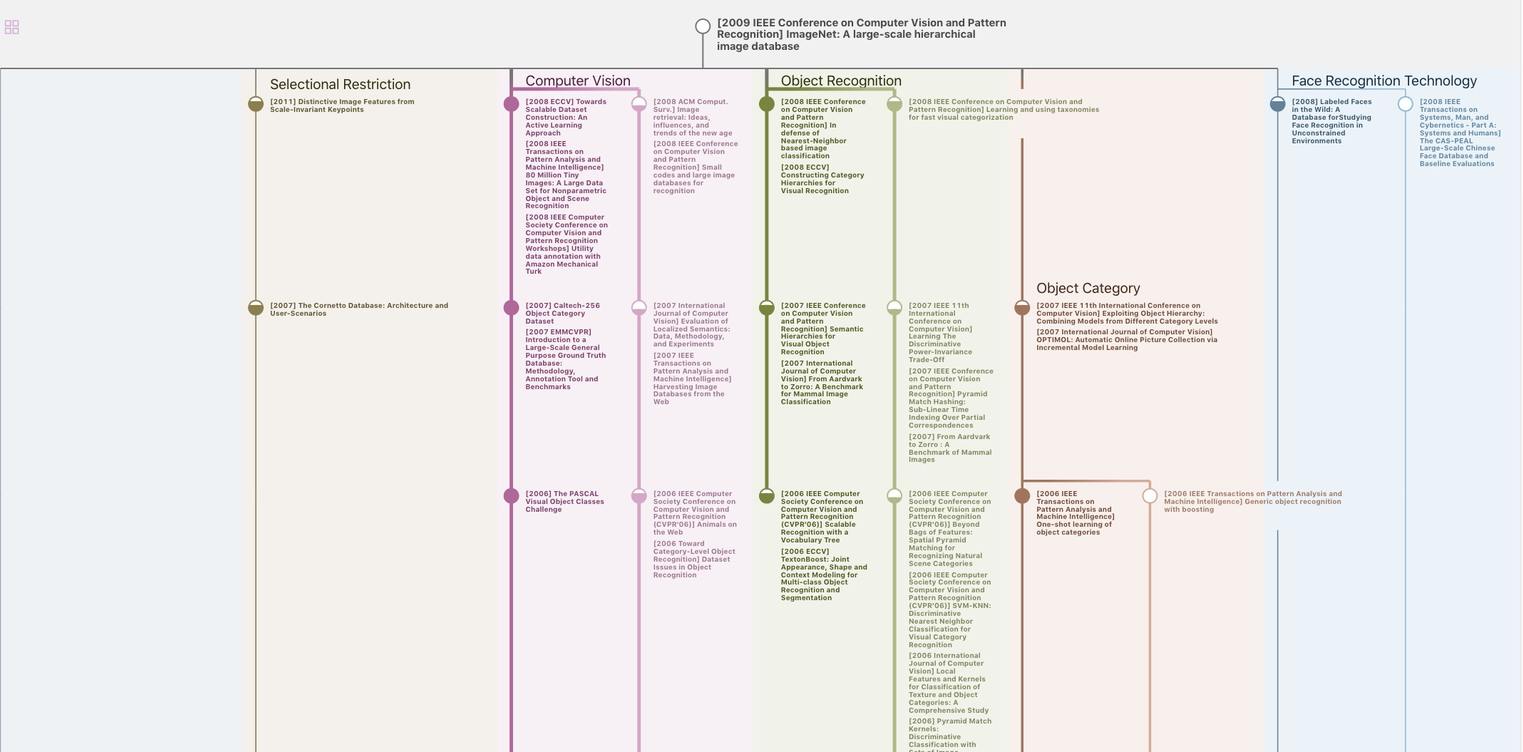
生成溯源树,研究论文发展脉络
Chat Paper
正在生成论文摘要