Unsupervised Evaluation and Optimization for Electrical Impedance Tomography
IEEE TRANSACTIONS ON INSTRUMENTATION AND MEASUREMENT(2021)
摘要
Electrical impedance tomography (EIT) is a radiation-free, fast, and convenient tomographic technology that uses only surface electrodes. EIT has the potential to provide continuous bedside monitoring of patients, for example, during mechanical ventilation. However, the soft-field nature of EIT leads to an ill-posed reconstruction problem that often results in multiple image solutions. This is exacerbated when EIT systems are used in variable measurement setups and scenarios, as few adjustment methods or EIT calibration approaches work reliably or require a reference phantom. The goals of this work are to design quality metrics to provide feedback for the EIT process to guide the system in adjusting the algorithm and parameters, for example, to alert the system in the event of poor image quality due to electrode connection variability. This then may be dealt with by physical electrode adjustment. In this article, the metrics, OAD and AL, representing object and artifact discreteness and artifact level, were designed to evaluate EIT image quality in an unsupervised way. Based on the quality index feedback, an image optimization process is proposed. The developed metrics and optimization methods were validated through data simulated in typical circular domain and thorax scenarios, and clinical data from human respiration. The conventional supervised evaluation metric, i.e., the correlation coefficient (COR) between the reference image and the reconstructed image, was used for comparison. The accuracy of new metrics was represented as the correlation coefficient of the new metric and COR. From the results, OAD and AL could improve the image quality by optimizing regularization parameters. The accuracy results of OAD and COR in the circular domain are 0.9429 and 0.9328, and those in thorax scenarios are 0.9741 and 0.8393. OAD and AL provide the evaluation of EIT images without using any prior knowledge or reference conductivity distribution, providing feedback to improve the reliability of EIT systems and reduce the risk of erroneous diagnosis.
更多查看译文
关键词
Artifact discreteness,artifact level (AL),electrical impedance tomography (EIT),image feature,image quality,unsupervised evaluation,ventilation monitoring
AI 理解论文
溯源树
样例
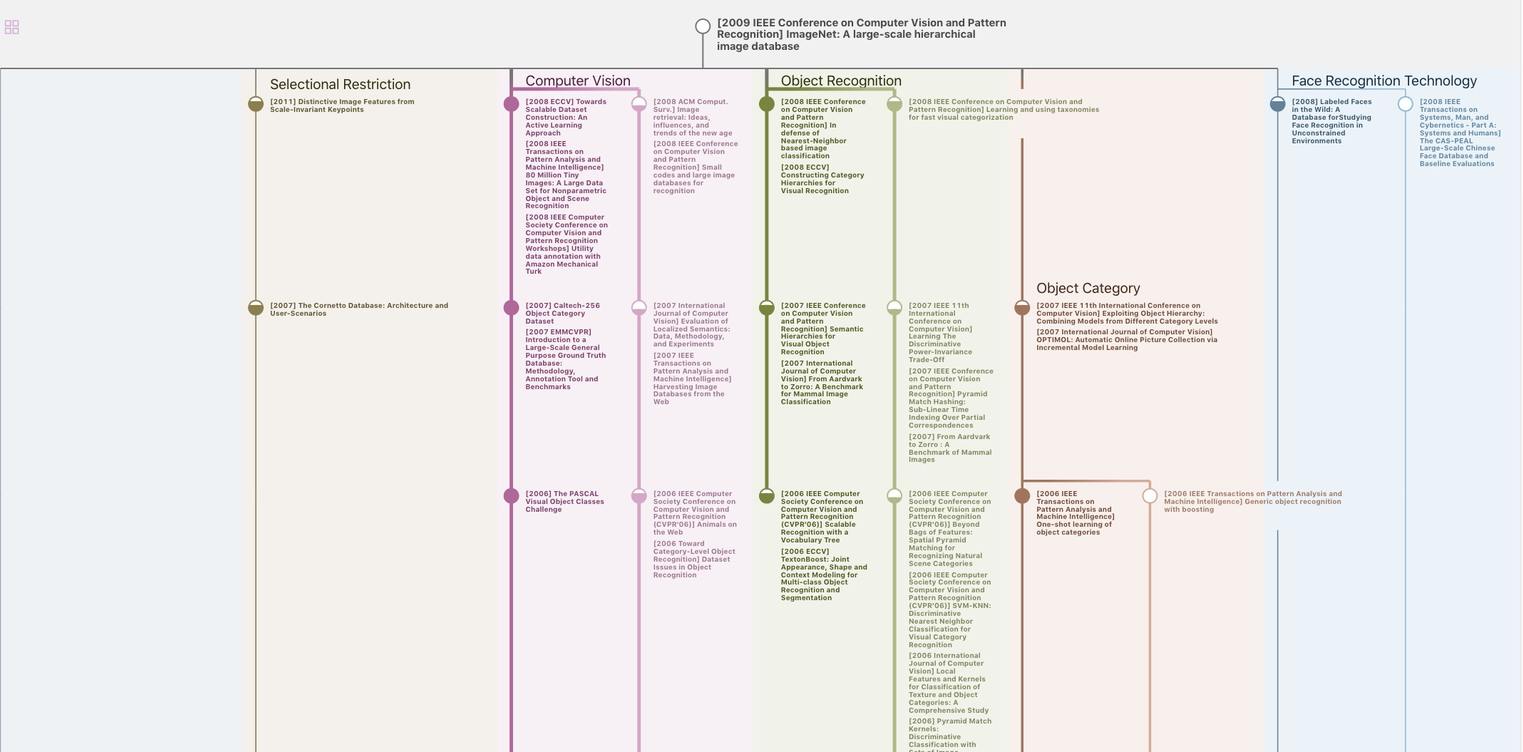
生成溯源树,研究论文发展脉络
Chat Paper
正在生成论文摘要