Transfer Learning for Event Detection From PMU Measurements With Scarce Labels
IEEE Access(2021)
摘要
Event detection in electrical grids is a challenging problem for machine learning methods due to spatiotemporally nonstationary systems and the inability to automate event labeling in high-volume data such as PMU measurements. As a result, the existing historical event logs created manually do not correlate well with the corresponding PMU measurements due to scarce and temporally imprecise labels. Trying to overcome this problem by extending event logs to a complete set of labeled events is very costly and often infeasible. We focused on utilizing a transfer learning model to reduce the need for additional data labeling by leveraging some labeled data instances available from a small number of well-defined event detection task. To demonstrate the feasibility, we tested our approach on a large dataset collected by 38 PMUs from the Western Interconnection of the U.S.A. over two years. The model evaluation performed based on varying percentages of labeled source data corresponding to
$\sim 20$
-700 characteristic events on different sizes of time windows ranging from 2-seconds to 1-minute demonstrates that the developed method can significantly improve automated event detection based on PMU measurements when extensive labeling is costly or impossible to obtain. When compared to the state-of-the-art machine learning algorithms (unsupervised, semi-supervised, and supervised), the results show that the transfer learning method has significantly better performances when detecting events by learning from as low as 20 representative labeled data instances.
更多查看译文
关键词
Big data applications, event detection, machine learning, phasor measurement units, power system faults, signal sampling, smart grids, time series analysis
AI 理解论文
溯源树
样例
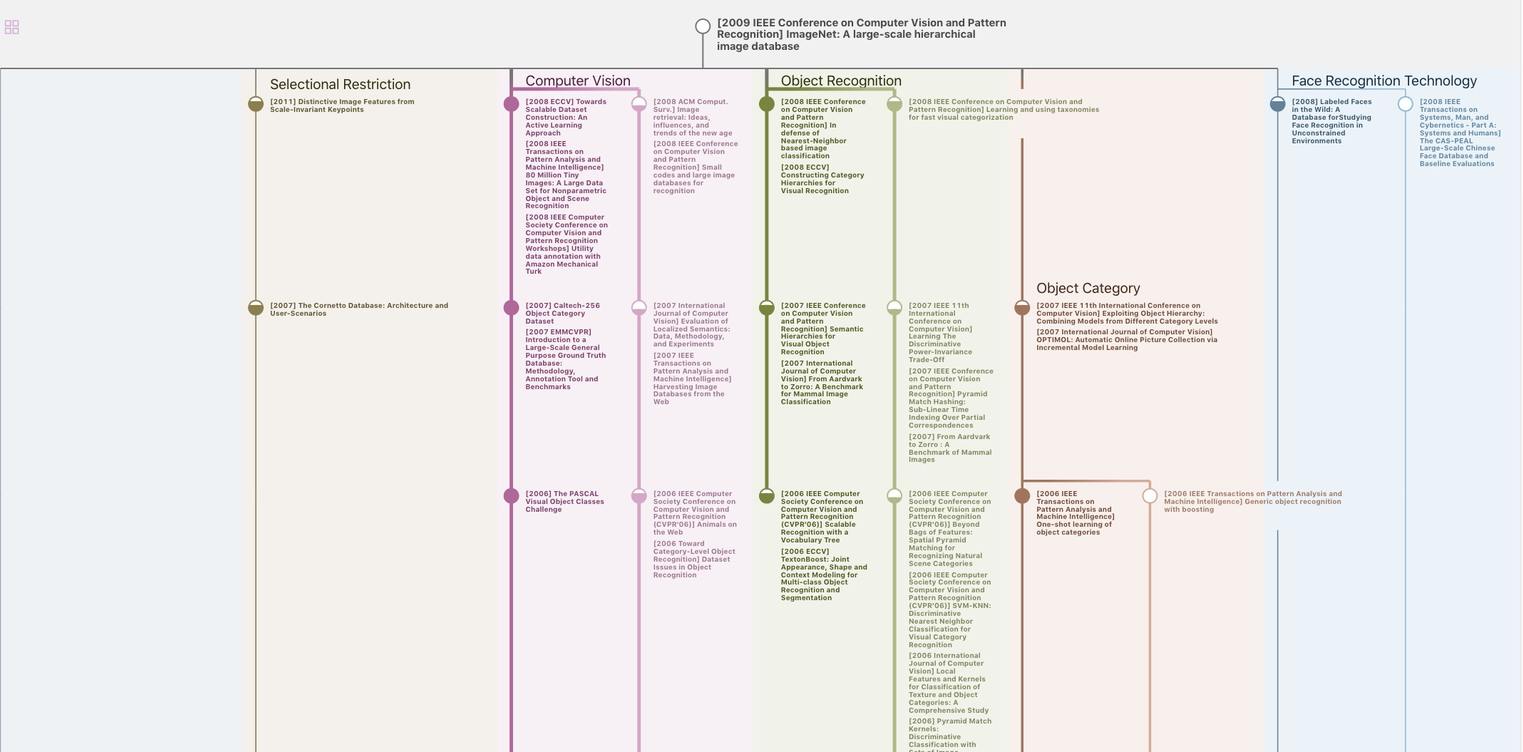
生成溯源树,研究论文发展脉络
Chat Paper
正在生成论文摘要