Spatial prediction of soil organic carbon stocks in an arid rangeland using machine learning algorithms
ENVIRONMENTAL MONITORING AND ASSESSMENT(2021)
摘要
Assessing the role of machine learning (ML) models concerning environmental predictors on spatial variation of soil organic carbon stocks (SOCS) in arid rangelands is very necessary. This study was conducted to explore the variability of surface SOCS in rangeland in the west of Iran using ML approaches. A number of 33 environmental predictors derived from Sentinel-2B and DEM were used. The optimal soil sampling ( n = 80) position was determined by Latin hypercube sampling (cLHS) method. Robust and popular random Forest (RF), cubist (CB) along with random forest-ordinary kriging (RF-OK), and cubist-ordinary kriging (CB-OK) hybrid ML models were applied to the prediction of SOCS. Ten-fold CV was implemented for modeling performance and uncertainty map. According to data analysis, the maximum, minimum, and average values of SOCS are 44.50, 10.50, and 20.50 (ton. ha −1 ) at the surface depth (0–30 cm), respectively. In general, normalized and standardized height covariates had a higher effect related to other predictors. On the other hand, two remote sensing (RS) indices, including salinity ratio (salinity) and GNDVI index, had a better impact on SOCS variability. The external validation of model performance indicated that RF-OK with ( R 2 = 0.75, RMSE = 6.33 ton. ha −1 ) with the high and low uncertainty range (3.33–9.50 ton. ha −1 ) was the outperformed ML model in compare with other models as RF ( R 2 = 0.65, RMSE = 7.38 ton. ha −1 ), CB-OK ( R 2 = 0.56, RMSE = 9.22 ton. ha −1 ), and CB ( R 2 = 0.33, RMSE = 10.42 ton. ha −1 ). In general, the hybrid models improved the accuracy of RF and CB with increased 0.11 until 0.23 of R 2 , and 1.05 to 1.2 (ton. ha −1 ) decreased RMSE of model’s prediction. Hence, we conclude that the topographic attributes (especially normalized and standardized height) were the most critical factors in controlling surface SOCS in arid rangelands when combining with robust RF ML model, and optimized soil sampling methods like RF-cLHS can prepare acceptable soil properties maps.
更多查看译文
关键词
Arid rangelands, Sentinel-2B indices, Soil organic carbon, Uncertainty, Hybrid models, Limited data
AI 理解论文
溯源树
样例
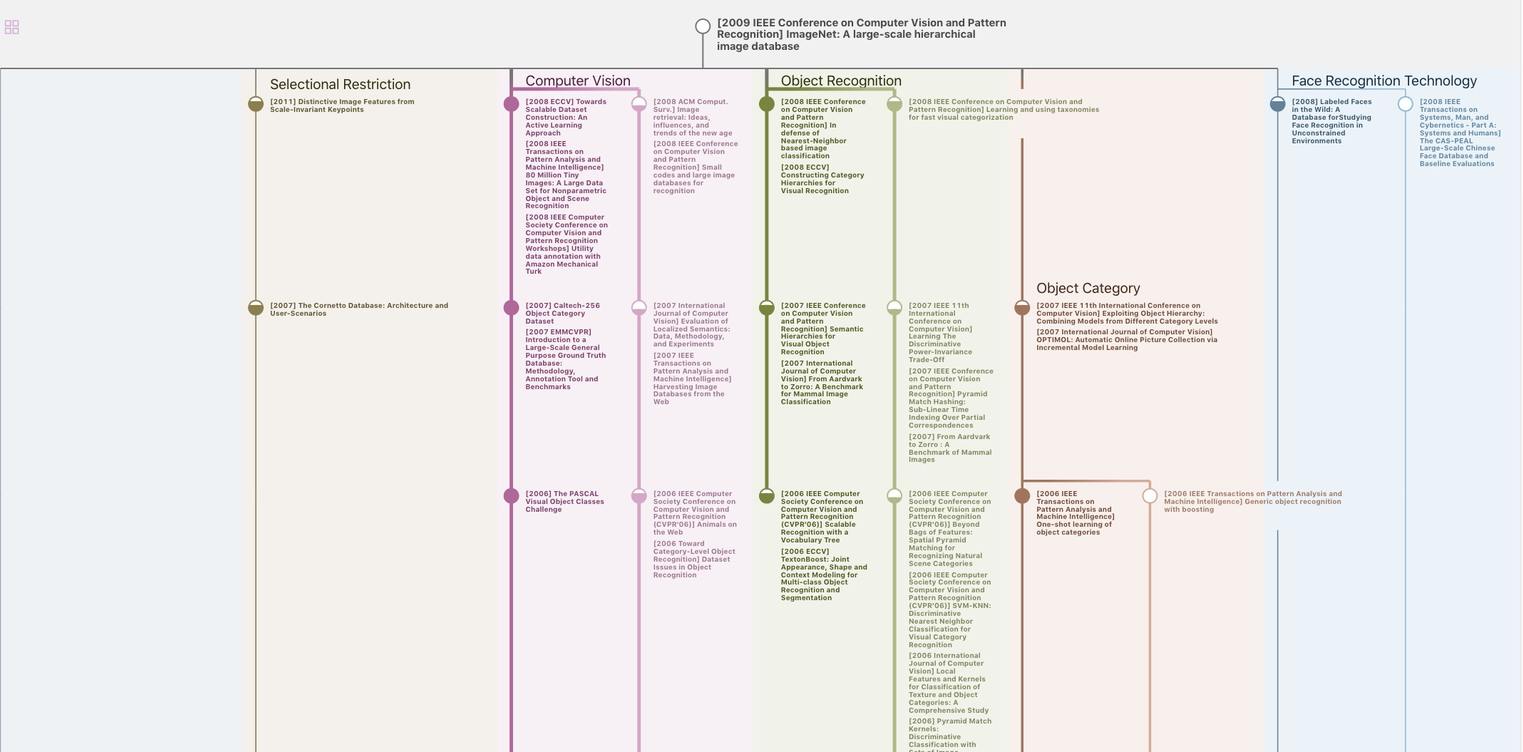
生成溯源树,研究论文发展脉络
Chat Paper
正在生成论文摘要