Rethinking 360 degrees Image Visual Attention Modelling with Unsupervised Learning.
ICCV(2021)
摘要
Despite the success of self-supervised representation learning on planar data, to date it has not been studied on 360 degrees images. In this paper, we extend recent advances in contrastive learning to learn latent representations that are sufficiently invariant to be highly effective for spherical saliency prediction as a downstream task. We argue that omni-directional images are particularly suited to such an approach due to the geometry of the data domain. To verify this hypothesis, we design an unsupervised framework that effectively maximizes the mutual information between the different views from both the equator and the poles. We show that the decoder is able to learn good quality saliency distributions from the encoder embeddings. Our model compares favorably with fully-supervised learning methods on the Salient360!, VR-EyeTracking and Sitzman datasets. This performance is achieved using an encoder that is trained in a completely unsupervised way and a relatively lightweight supervised decoder (3.8 x fewer parameters in the case of the ResNet50 encoder). We believe that this combination of supervised and unsupervised learning is an important step toward flexible formulations of human visual attention. The results can be reproduced on GitHub
更多查看译文
AI 理解论文
溯源树
样例
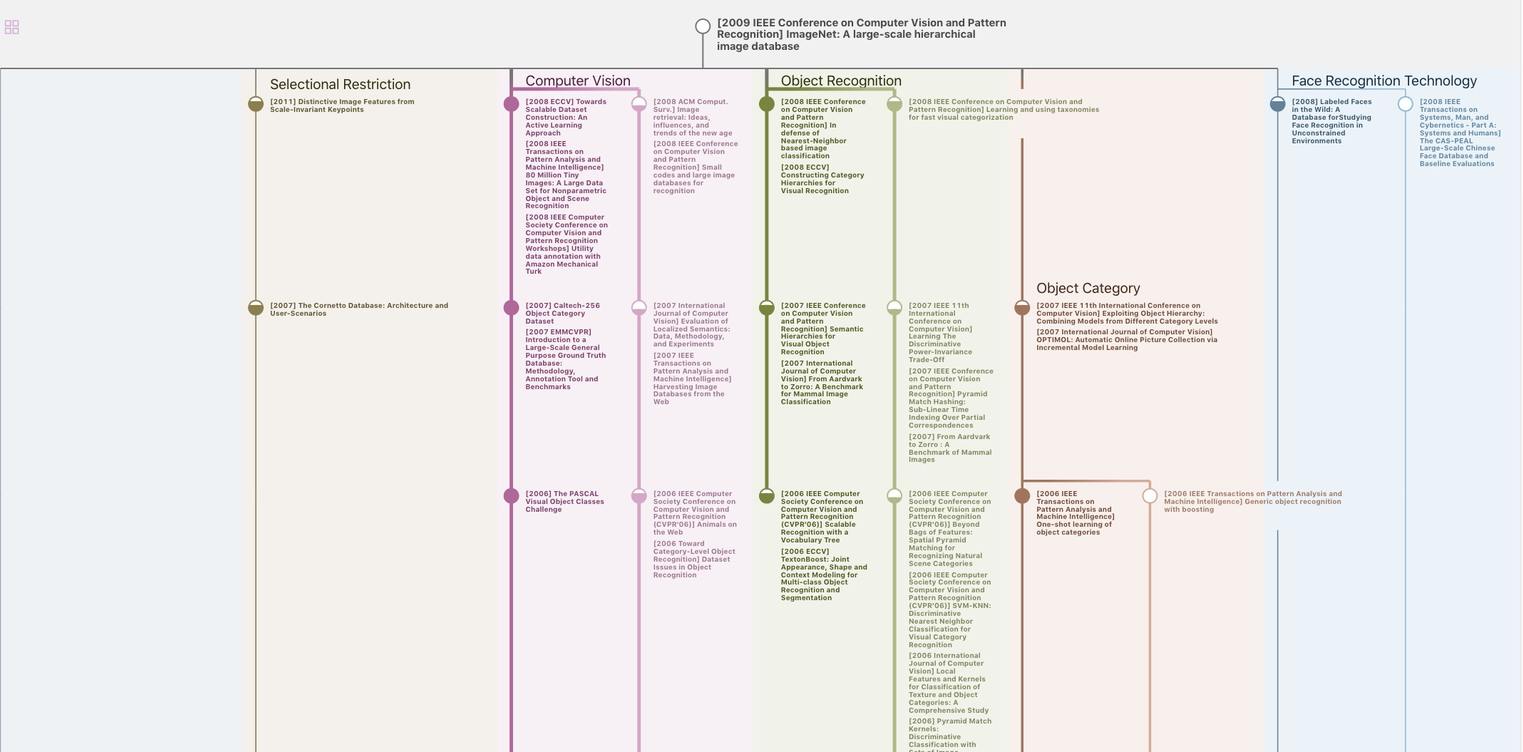
生成溯源树,研究论文发展脉络
Chat Paper
正在生成论文摘要