Latent Transformations via NeuralODEs for GAN-based Image Editing.
ICCV(2021)
摘要
Recent advances in high-fidelity semantic image editing heavily rely on the presumably disentangled latent spaces of the state-of-the-art generative models, such as StyleGAN. Specifically, recent works show that it is possible to achieve decent controllability of attributes in the face images via linear shifts along with latent directions. Several recent methods address the discovery of such directions, implicitly assuming that the state-of-the-art GANs learn the latent spaces with inherently linearly separable attribute distributions and semantic vector arithmetic properties. In our work, we show that nonlinear latent code manipulations realized as flows of a trainable Neural ODE are beneficial for many practical non-face image domains with more complex non-textured factors of variation. In particular, we investigate a large number of datasets with known attributes and demonstrate that certain attribute manipulations are challenging to be obtained with linear shifts only.
更多查看译文
关键词
image editing,latent transformations,neuralodes,gan-based
AI 理解论文
溯源树
样例
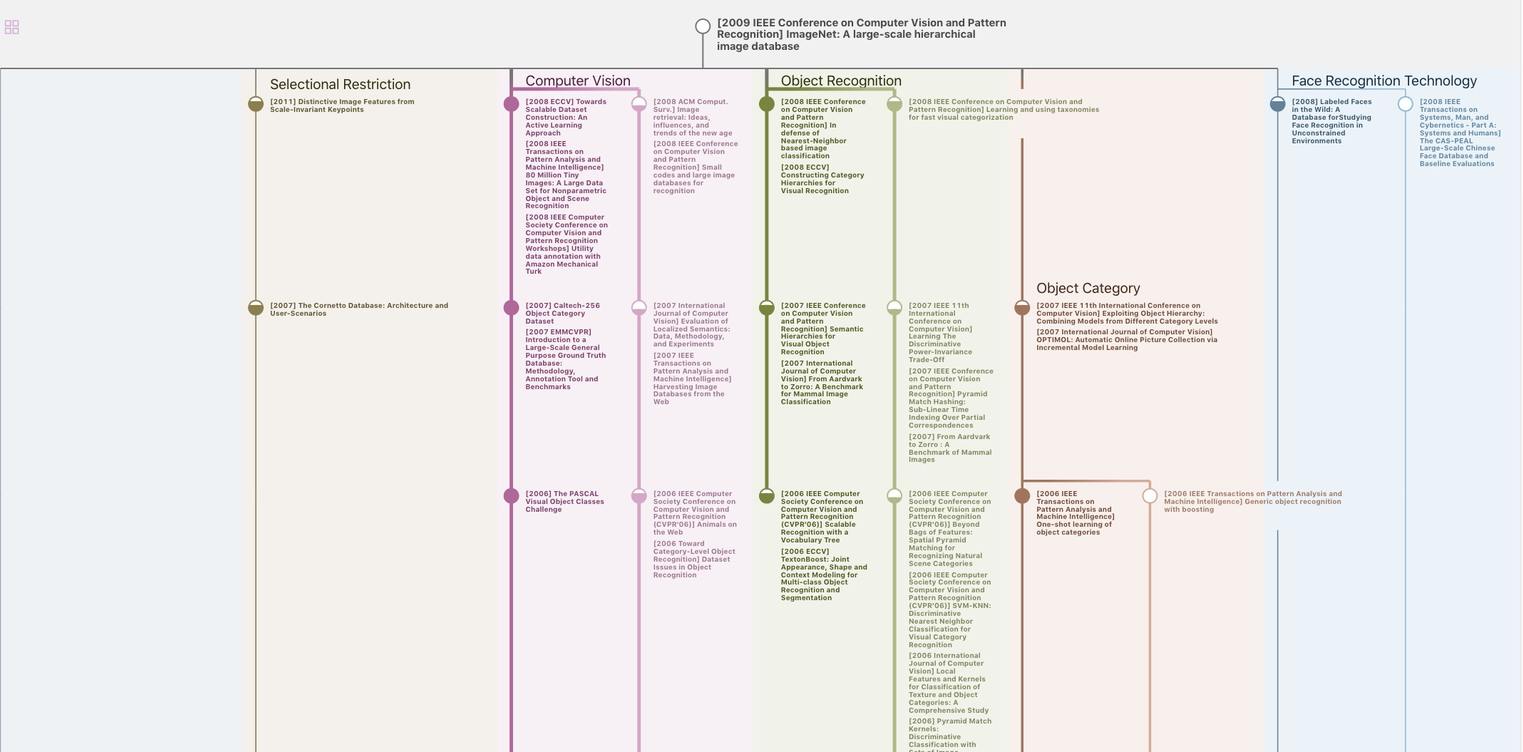
生成溯源树,研究论文发展脉络
Chat Paper
正在生成论文摘要