FLAR - A Unified Prototype Framework for Few-sample Lifelong Active Recognition.
ICCV(2021)
摘要
Intelligent agents with visual sensors are allowed to actively explore their observations for better recognition performance. This task is referred to as Active Recognition (AR). Currently, most methods toward AR are implemented under a fixed-category setting, which constrains their applicability in realistic scenarios that need to incrementally learn new classes without retraining from scratch. Further, collecting massive data for novel categories is expensive. To address this demand, in this paper, we propose a unified framework towards Few-sample Lifelong Active Recognition (FLAR), which aims at performing active recognition on progressively arising novel categories that only have few training samples. Three difficulties emerge with FLAR: the lifelong recognition policy learning, the knowledge preservation of old categories, and the lack of training samples. To this end, our approach integrates prototypes, a robust representation for limited training samples, into a reinforcement learning solution, which motivates the agent to move towards views resulting in more discriminative features. Catastrophic forgetting during lifelong learning is then alleviated with knowledge distillation. Extensive experiments across two datasets, respectively for object and scene recognition, demonstrate that even without large training samples, the proposed approach could learn to actively recognize novel categories in a class-incremental behavior.
更多查看译文
关键词
Vision for robotics and autonomous vehicles,Recognition and classification,Transfer/Low-shot/Semi/Unsupervised Learning
AI 理解论文
溯源树
样例
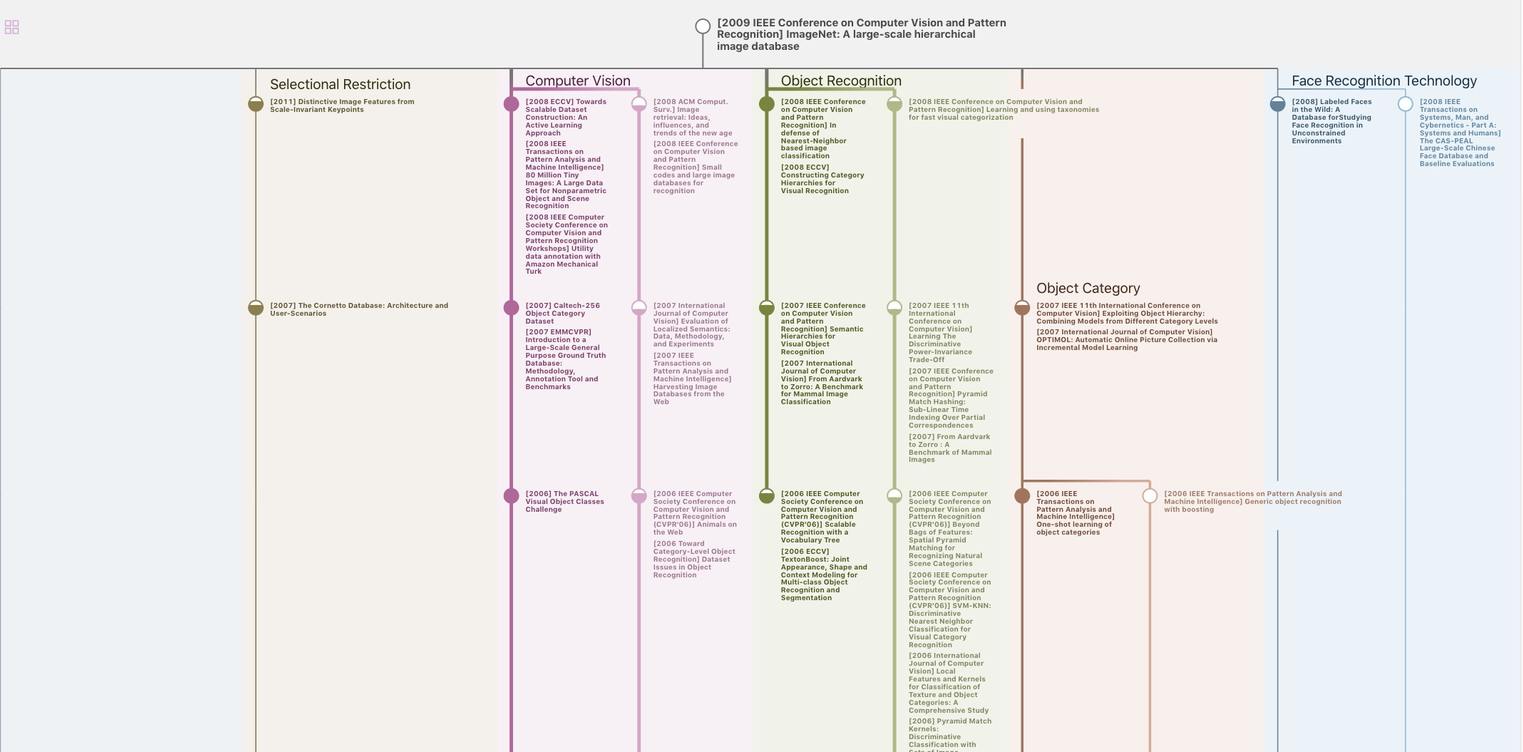
生成溯源树,研究论文发展脉络
Chat Paper
正在生成论文摘要