Direct Antimicrobial Resistance Prediction from clinical MALDI-TOF mass spectra using Machine Learning
bioRxiv (Cold Spring Harbor Laboratory)(2021)
摘要
Early administration of effective antimicrobial treatments is critical for the outcome of infections. Antimicrobial resistance testing enables the selection of optimal antibiotic treatments, but current culture-based techniques take up to 72 hours. We have developed a novel machine learning approach to predict antimicrobial resistance directly from MALDI-TOF mass spectra profiles of clinical samples. We trained calibrated classifiers on a newly-created publicly available database of mass spectra profiles from clinically most relevant isolates with linked antimicrobial susceptibility phenotypes. The dataset combines more than 300,000 mass spectra with more than 750,000 antimicrobial resistance phenotypes from four medical institutions. Validation against a panel of clinically important pathogens, including Staphylococcus aureus , Escherichia coli , and Klebsiella pneumoniae , resulting in AUROC values of 0.8, 0.74, and 0.74 respectively, demonstrated the potential of using machine learning to substantially accelerate antimicrobial resistance determination and change of clinical management. Furthermore, a retrospective clinical case study found that implementation of this approach would have resulted in a beneficial change in the clinical treatment in 88% (8/9) of cases. MALDI-TOF mass spectra based machine learning may thus be an important new tool for antibiotic stewardship.
### Competing Interest Statement
The authors have declared no competing interest.
更多查看译文
关键词
antimicrobial,machine learning,resistance,maldi-tof
AI 理解论文
溯源树
样例
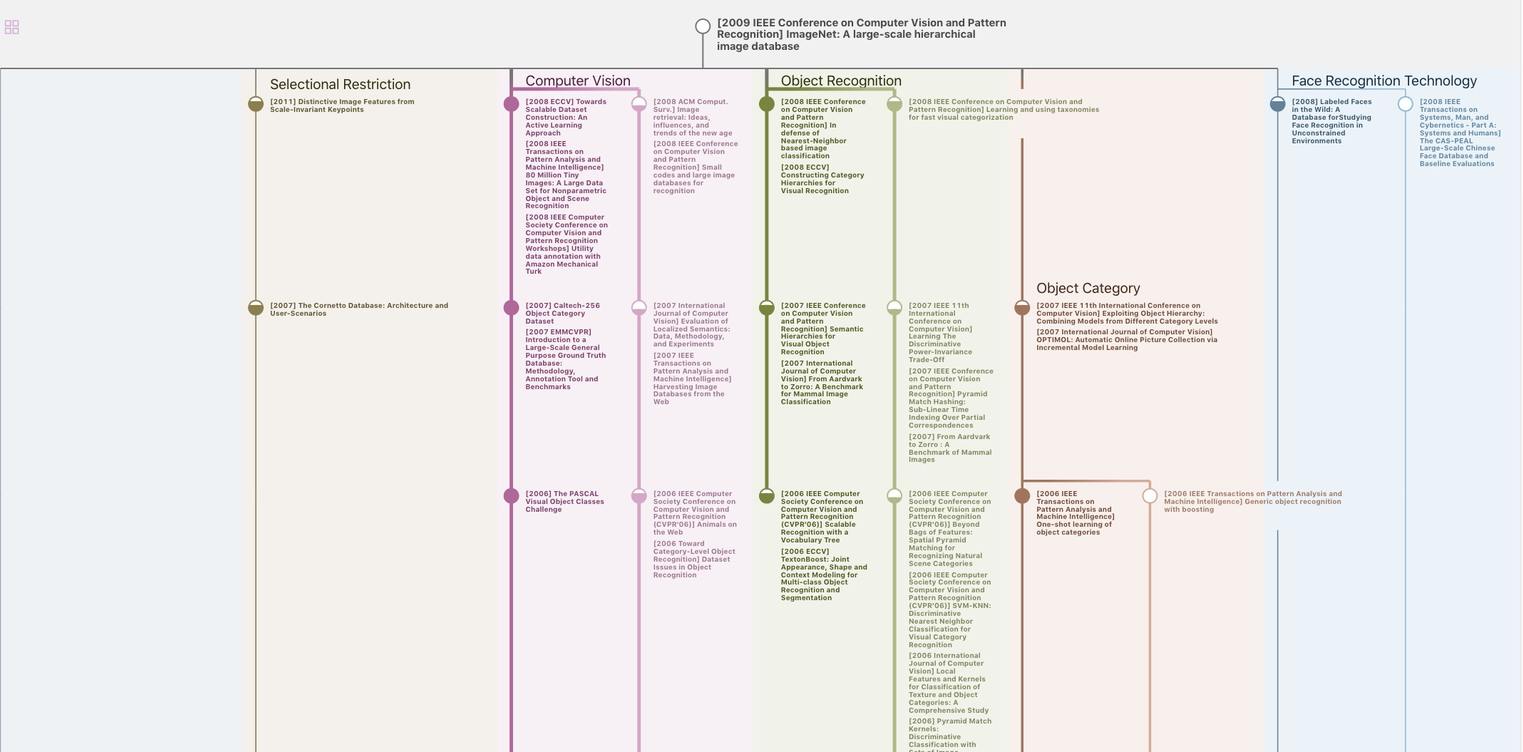
生成溯源树,研究论文发展脉络
Chat Paper
正在生成论文摘要