Deep-learning-based identification, tracking, pose estimation and behaviour classification of interacting primates and mice in complex environments
Nature Machine Intelligence(2022)
摘要
Quantification of behaviours of interest from video data is commonly used to study brain function, the effects of pharmacological interventions, and genetic alterations. Existing approaches lack the capability to analyse the behaviour of groups of animals in complex environments. We present a novel deep learning architecture for classifying individual and social animal behaviour—even in complex environments directly from raw video frames—that requires no intervention after initial human supervision. Our behavioural classifier is embedded in a pipeline (SIPEC) that performs segmentation, identification, pose-estimation and classification of complex behaviour, outperforming the state of the art. SIPEC successfully recognizes multiple behaviours of freely moving individual mice as well as socially interacting non-human primates in three dimensions, using data only from simple mono-vision cameras in home-cage set-ups. The use of deep neural networks for the automated analysis of behavioural videos has emerged as a tool in neuroscience, medicine and psychology. Marks and colleagues present a pipeline capable of tracking and identifying animals, as well as classifying individual and interacting animal behaviour in video recordings and even in complex environments.
更多查看译文
AI 理解论文
溯源树
样例
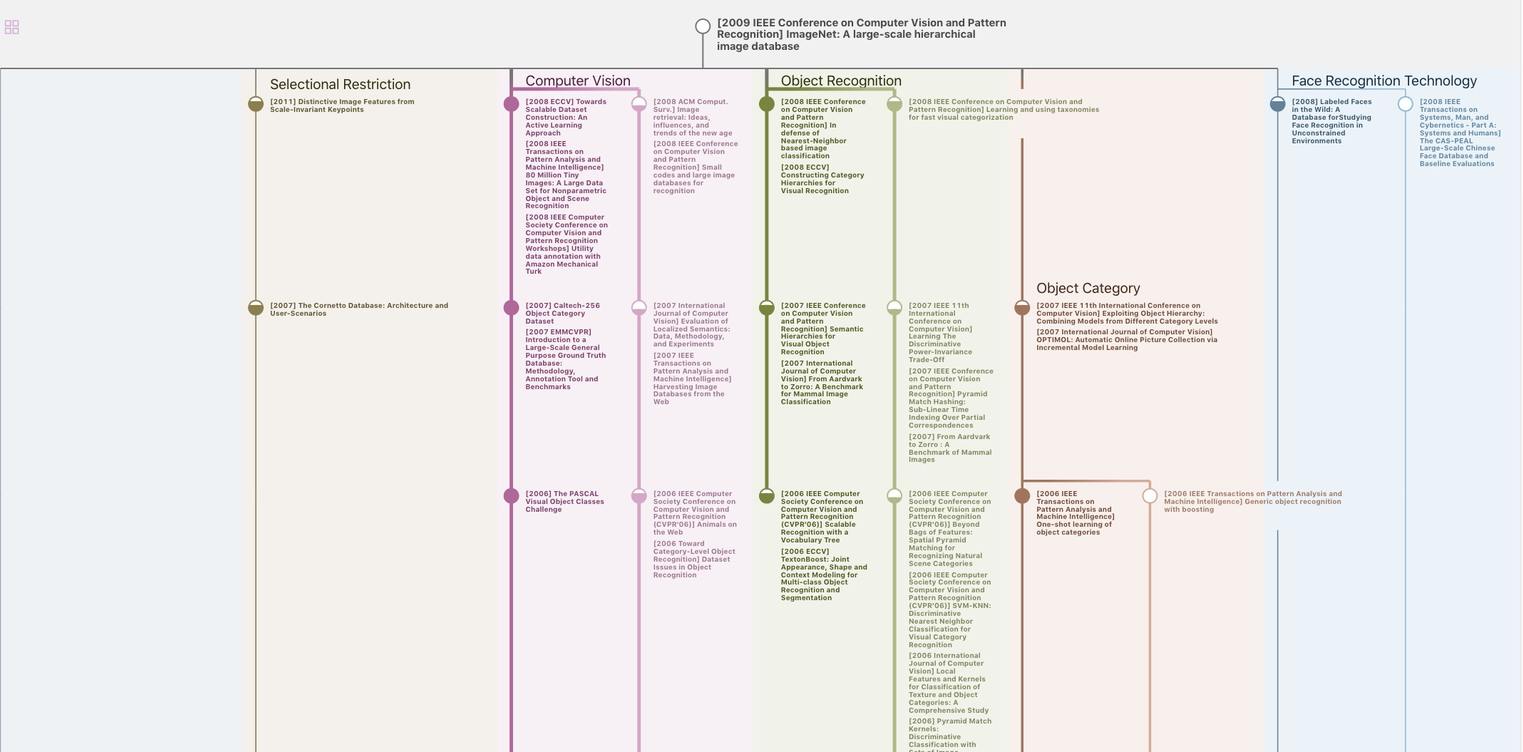
生成溯源树,研究论文发展脉络
Chat Paper
正在生成论文摘要