Classification for Breast Ultrasound Using Convolutional Neural Network with Multiple Time-Domain Feature Maps
APPLIED SCIENCES-BASEL(2021)
摘要
Ultrasound (US) imaging is widely utilized as a diagnostic screening method, and deep learning has recently drawn attention for the analysis of US images for the pathological status of tissues. While low image quality and poor reproducibility are the common obstacles in US analysis, the small size of the dataset is a new limitation for deep learning due to lack of generalization. In this work, a convolutional neural network (CNN) using multiple feature maps, such as entropy and phase images, as well as a B-mode image, was proposed to classify breast US images. Although B-mode images contain both anatomical and textual information, traditional CNNs experience difficulties in abstracting features automatically, especially with small datasets. For the proposed CNN framework, two distinct feature maps were obtained from a B-mode image and utilized as new inputs for training the CNN. These feature maps can also be made from the evaluation data and applied to the CNN separately for the final classification decision. The experimental results with 780 breast US images in three categories of benign, malignant, and normal, showed that the proposed CNN framework using multiple feature maps exhibited better performances than the traditional CNN with B-mode only for most deep network models.
更多查看译文
关键词
medical ultrasound, breast US images, deep learning, convolutional neural network, B-mode image, entropy image, phase image
AI 理解论文
溯源树
样例
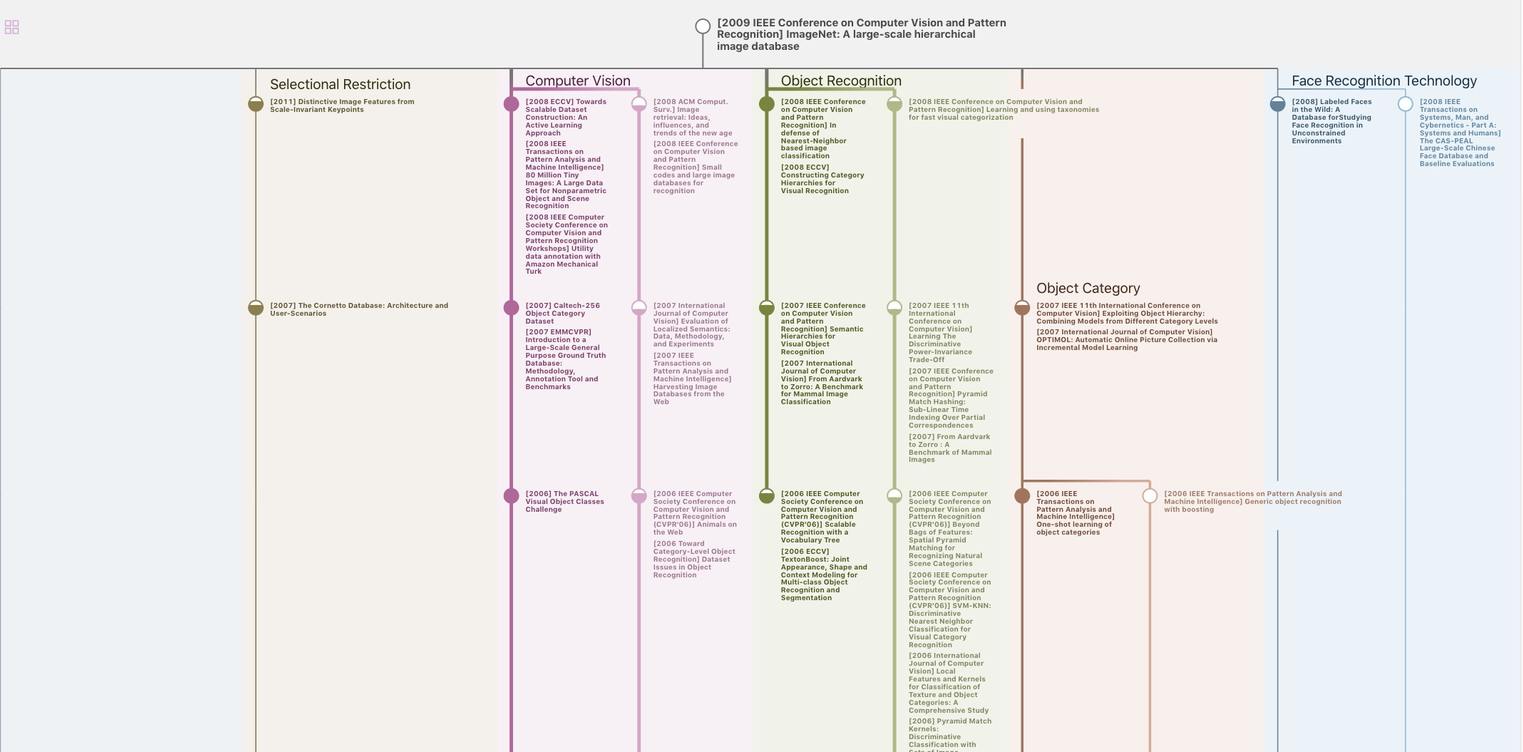
生成溯源树,研究论文发展脉络
Chat Paper
正在生成论文摘要