DeepMosaic: Control-independent mosaic single nucleotide variant detection using deep convolutional neural networks
biorxiv(2021)
摘要
Mosaic variants (MVs) reflect mutagenic processes during embryonic development[1][1] and environmental exposure[2][2], accumulate with aging, and underlie diseases such as cancer and autism[3][3]. The detection of MVs has been computationally challenging due to sparse representation in non-clonally expanded tissues. While heuristic filters and tools trained on clonally expanded MVs with high allelic fractions are proposed, they show relatively lower sensitivity and more false discoveries[4][4]–[9][5]. Here we present DeepMosaic, combining an image-based visualization module for single nucleotide MVs, and a convolutional neural networks-based classification module for control-independent MV detection. DeepMosaic achieved higher accuracy compared with existing methods on biological and simulated sequencing data, with a 96.34% (158/164) experimental validation rate. Of 932 mosaic variants detected by DeepMosaic in 16 whole genome sequenced samples, 21.89-58.58% (204/932-546/932) MVs were overlooked by other methods. Thus, DeepMosaic represents a highly accurate MV classifier that can be implemented as an alternative or complement to existing methods.
### Competing Interest Statement
The authors have declared no competing interest.
[1]: #ref-1
[2]: #ref-2
[3]: #ref-3
[4]: #ref-4
[5]: #ref-9
更多查看译文
关键词
deepmosaic convolutional neural networks,control-independent
AI 理解论文
溯源树
样例
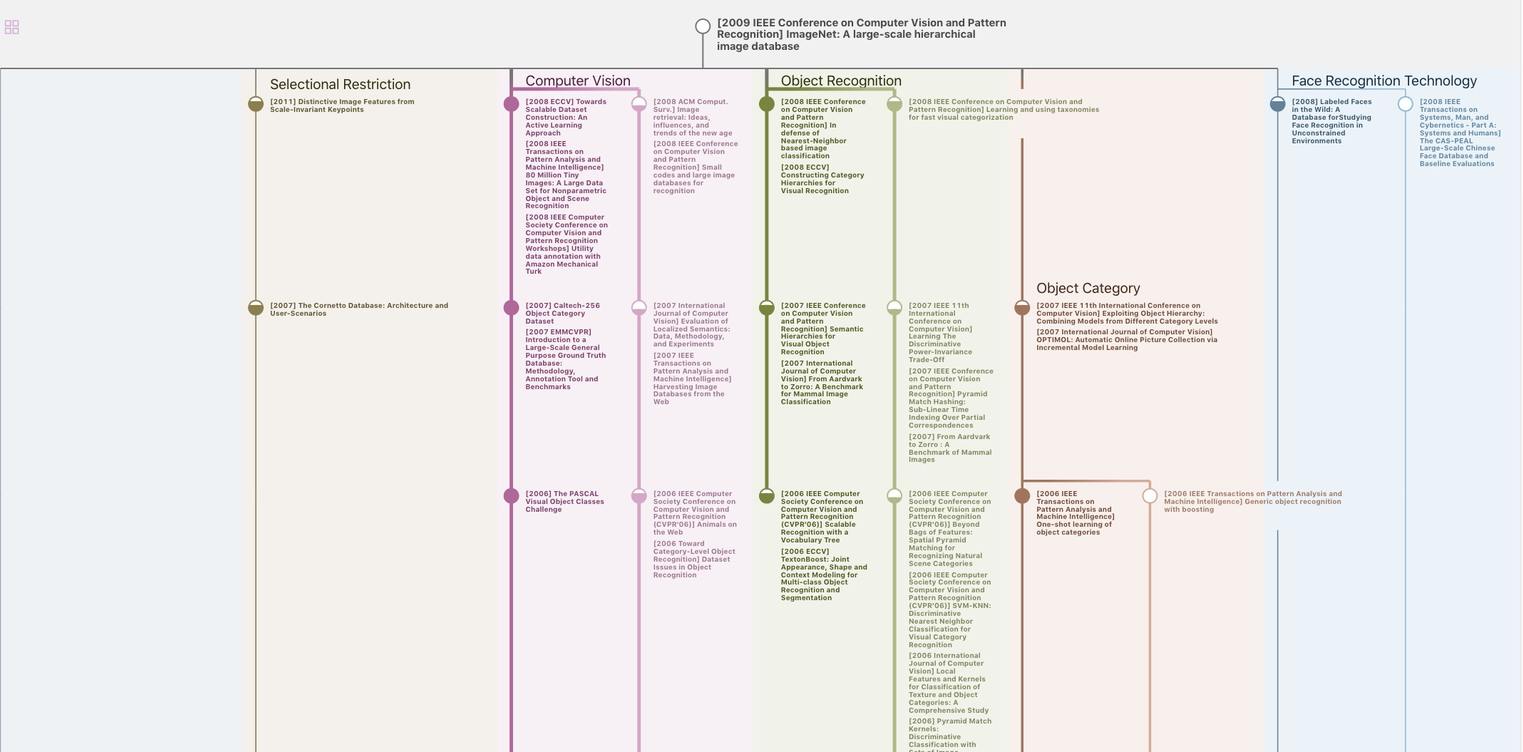
生成溯源树,研究论文发展脉络
Chat Paper
正在生成论文摘要