Data-driven filtration and segmentation of mesoscale neural dynamics
biorxiv(2021)
摘要
Recording neuronal group activity across the cortical hemispheres from awake, behaving mice is essential for understanding information flow across cerebral networks. Video recordings of cerebral function comes with challenges, including optical and movement-associated vessel artifacts, and limited references for time series extraction. Here we present a data-driven workflow that isolates artifacts from calcium activity patterns, and segments independent functional units across the cortical surface. Independent Component Analysis utilizes the statistical interdependence of pixel activation to completely unmix signals from background noise, given sufficient spatial and temporal samples. We also utilize isolated signal components to produce segmentations of the cortical surface, unique to each individual’s functional patterning. Time series extraction from these maps maximally represent the underlying signal in a highly compressed format. These improved techniques for data pre-processing, spatial segmentation, and time series extraction result in optimal signals for further analysis.
### Competing Interest Statement
The authors have declared no competing interest.
* ΔF/F (dFoF)
: change in fluorescence over mean fluorescence
ICA
: Independent Component Analysis
PCA
: Principal Component Analysis
Domain Map
: maximum projection map of ICA components
Domain
: A single contiguous unit from a domain map, represents an ICA component’s maximal region of influence
Mosiac Movie
: a video representation of the time series extracted under each domain in the domain map
更多查看译文
关键词
mesoscale,segmentation,dynamics,filtration,data-driven
AI 理解论文
溯源树
样例
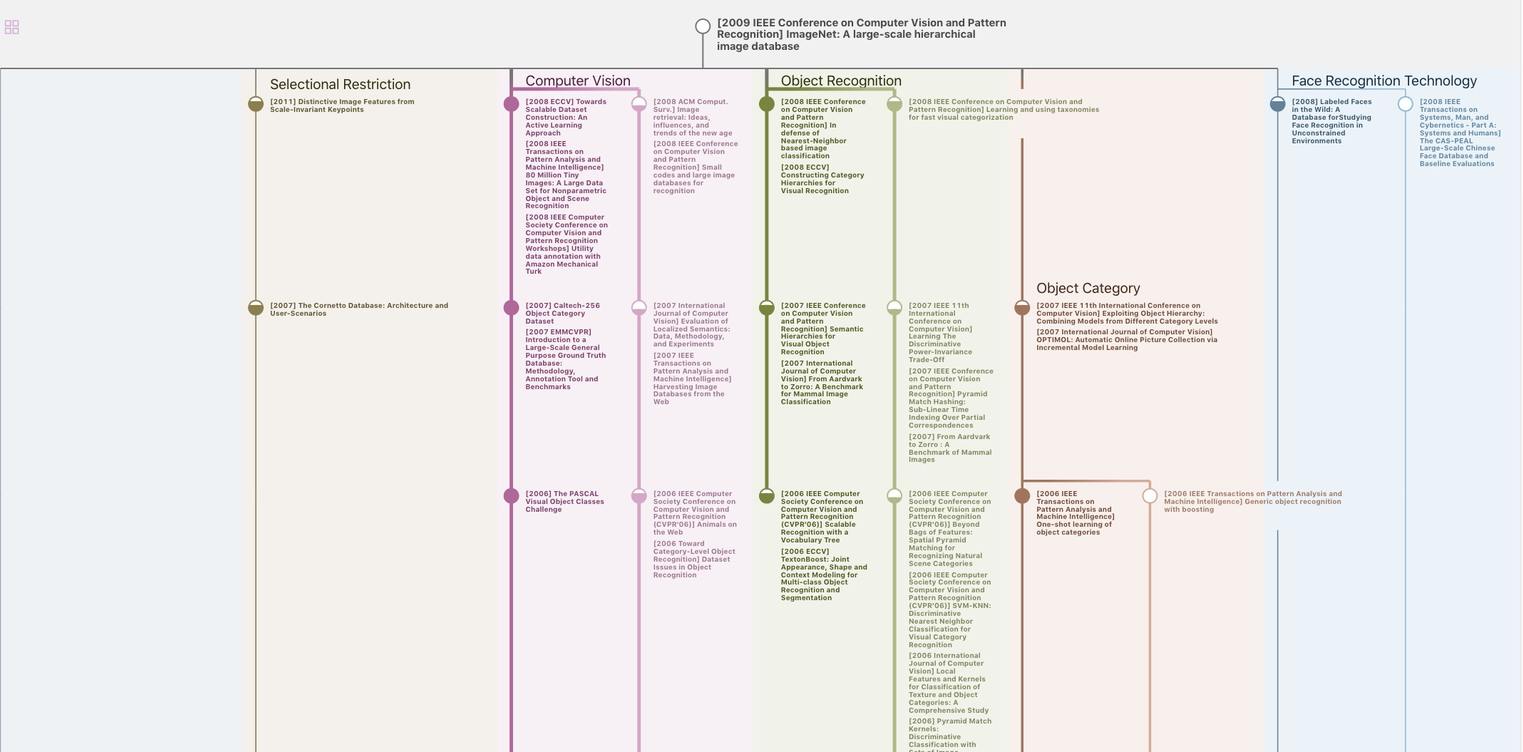
生成溯源树,研究论文发展脉络
Chat Paper
正在生成论文摘要