SuperMICE: An Ensemble Machine Learning Approach to Multiple Imputation by Chained Equations
AMERICAN JOURNAL OF EPIDEMIOLOGY(2022)
摘要
Researchers often face the problem of how to address missing data. Multiple imputation is a popular approach, with multiple imputation by chained equations (MICE) being among the most common and flexible methods for execution. MICE iteratively fits a predictive model for each variable with missing values, conditional on other variables in the data. In theory, any imputation model can be used to predict the missing values. However, if the predictive models are incorrectly specified, they may produce biased estimates of the imputed data, yielding inconsistent parameter estimates and invalid inference. Given the set of modeling choices that must be made in conducting multiple imputation, in this paper we propose a data-adaptive approach to model selection. Specifically, we adapt MICE to incorporate an ensemble algorithm, Super Learner, to predict the conditional mean for each missing value, and we also incorporate a local kernel-based estimate of variance. We present a set of simulations indicating that this approach produces final parameter estimates with lower bias and better coverage than other commonly used imputation methods. These results suggest that using a flexible machine learning imputation approach can be useful in settings where data are missing at random, especially when the relationships among the variables are complex.
更多查看译文
关键词
machine learning, missing data, missingness at random, multiple imputation by chained equations, simulation
AI 理解论文
溯源树
样例
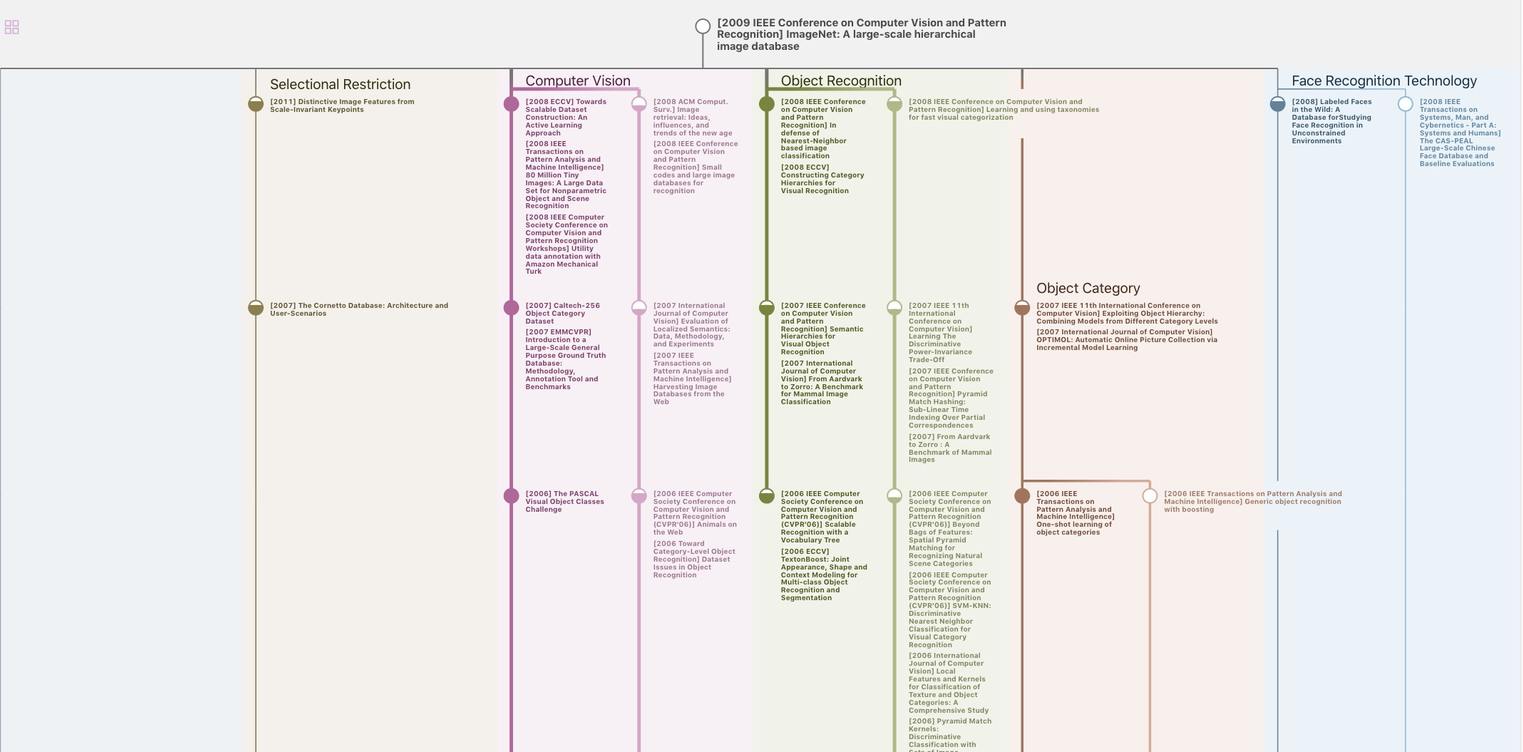
生成溯源树,研究论文发展脉络
Chat Paper
正在生成论文摘要