Machine Learning to Summarize and Provide Context for Sleep and Eating Schedules
biorxiv(2021)
摘要
The relative timing of sleep and of eating within the circadian day is important for human health. Despite much data on sleep, and a growing data set for eating, there remains a need for an interpretative framework for the understanding of this data for health decisions. This study provides a new statistical and machine learning analysis of more than 500 participants in the Daily24 project. From their data, and the analysis, we propose a framework for determining the classification of participants into different chronotypes and with that the ability to realize the potential impact of daily circadian habits on health. We propose that our resulting distribution curves could be used, similar to NHANES (National Health and Nutrition Examination Survey) data for pediatric growth, as a measure for circadian misalignment and used to help guide re-entrainment schedules.
Author summary Daily habits can be positive, negative or neutral for human health. Generally sleep and eating schedules are assumed without thought for their potential to help or interfere with health. In this study we propose a framework, based on data from more than 500 participants, for evaluating the relative timing of meals and sleep schedules. This evaluation, similar to pediatric growth charts, can guide clinical suggestions for those at the extremes, while helping others to realize that they are unusual relative to the population average
### Competing Interest Statement
The authors have declared no competing interest.
更多查看译文
关键词
eating schedules,machine learning,sleep
AI 理解论文
溯源树
样例
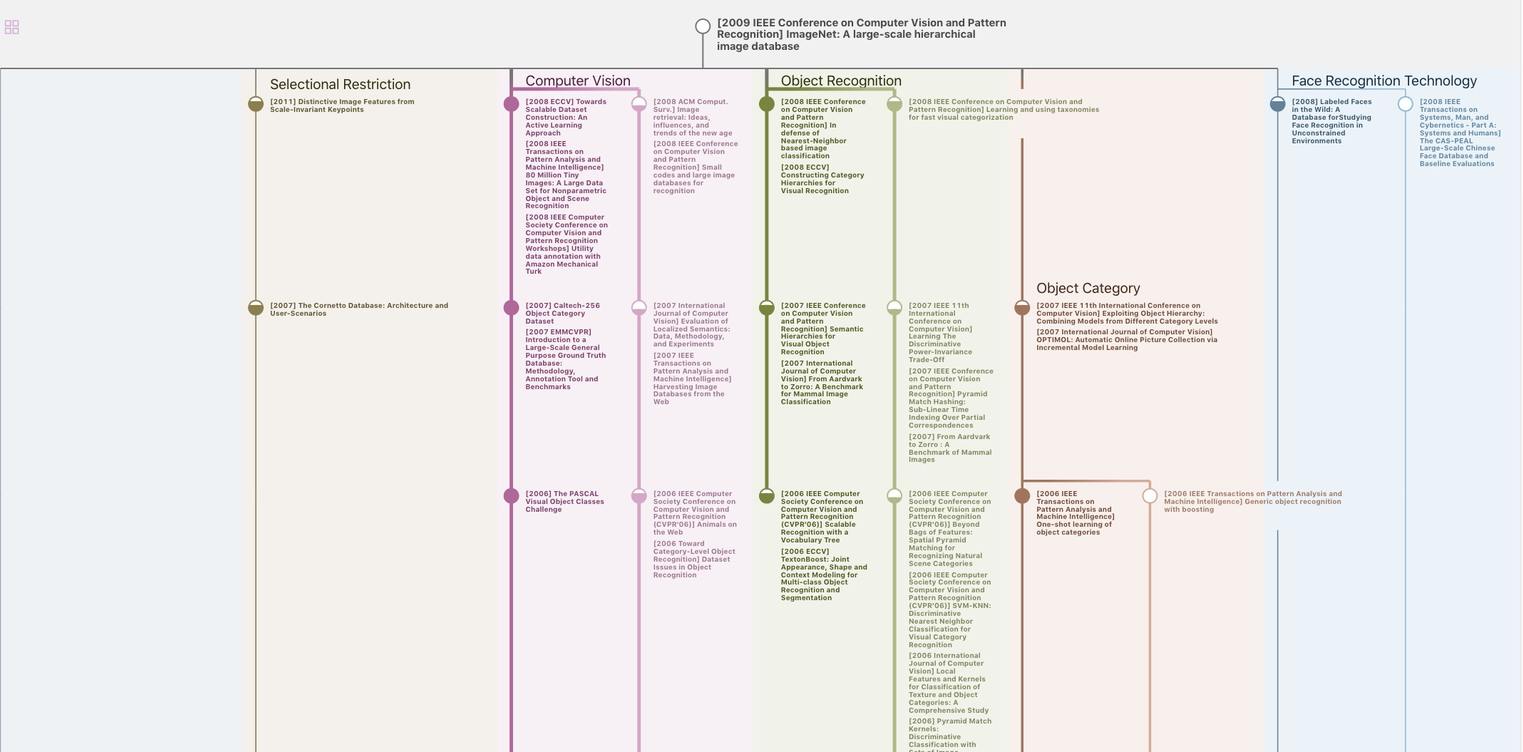
生成溯源树,研究论文发展脉络
Chat Paper
正在生成论文摘要