A Combined Model Based On Eobl-Cssa-Lssvm For Power Load Forecasting
SYMMETRY-BASEL(2021)
摘要
Inaccurate electricity load forecasting can lead to the power sector gaining asymmetric information in the supply and demand relationship. This asymmetric information can lead to incorrect production or generation plans for the power sector. In order to improve the accuracy of load forecasting, a combined power load forecasting model based on machine learning algorithms, swarm intelligence optimization algorithms, and data pre-processing is proposed. Firstly, the original signal is pre-processed by the VMD-singular spectrum analysis data pre-processing method. Secondly, the noise-reduced signals are predicted using the Elman prediction model optimized by the sparrow search algorithm, the ELM prediction model optimized by the chaotic adaptive whale algorithm (CAWOA-ELM), and the LSSVM prediction model optimized by the chaotic sparrow search algorithm based on elite opposition-based learning (EOBL-CSSA-LSSVM) for electricity load data, respectively. Finally, the weighting coefficients of the three prediction models are calculated using the simulated annealing algorithm and weighted to obtain the prediction results. Comparative simulation experiments show that the VMD-singular spectrum analysis method and two improved intelligent optimization algorithms proposed in this paper can effectively improve the prediction accuracy. Additionally, the combined forecasting model proposed in this paper has extremely high forecasting accuracy, which can help the power sector to develop a reasonable production plan and power generation plans.
更多查看译文
关键词
neural network, sparrow search algorithm, whale optimization algorithm, power load forecast, machine learning
AI 理解论文
溯源树
样例
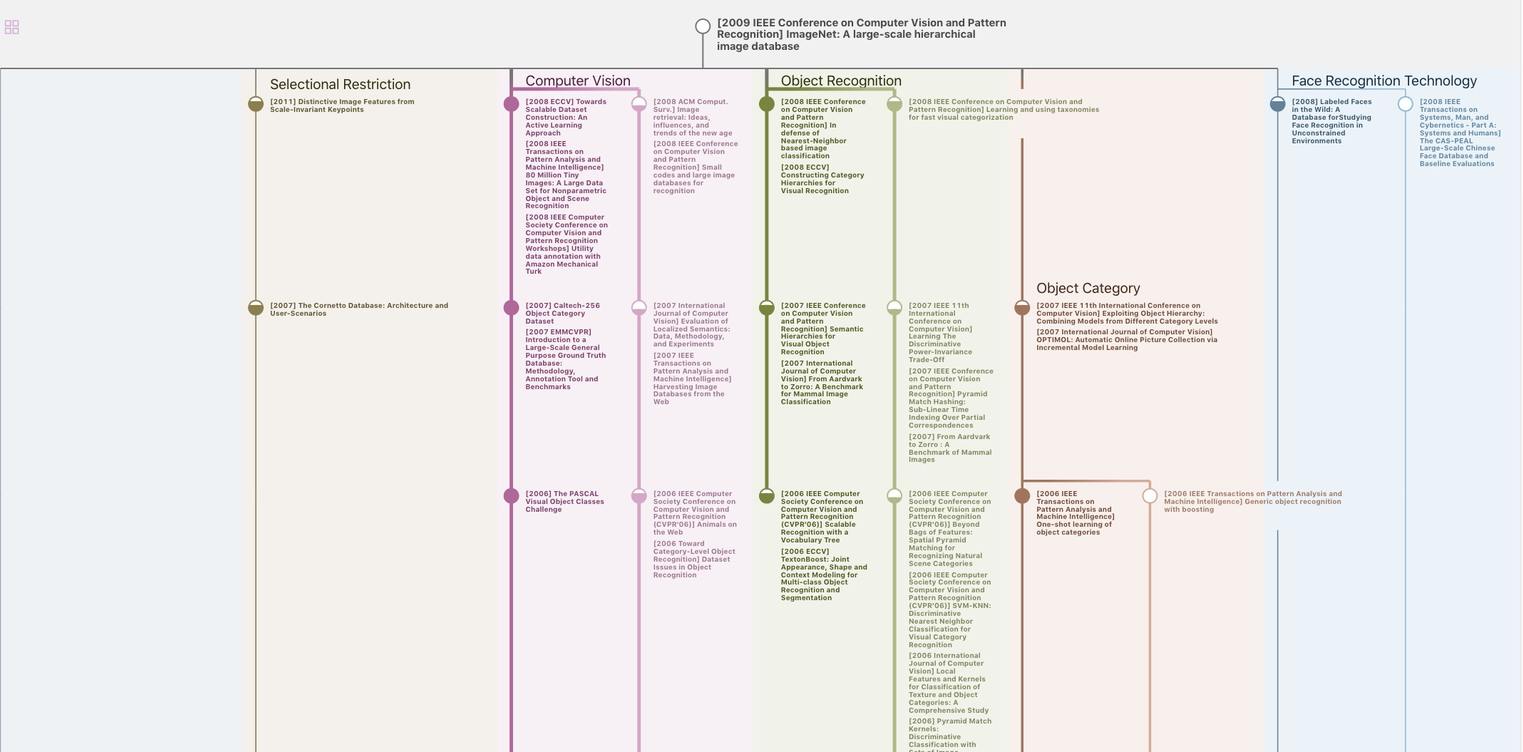
生成溯源树,研究论文发展脉络
Chat Paper
正在生成论文摘要