Learning decomposed hierarchical feature for better transferability of deep models
Information Sciences(2021)
摘要
Deep models have achieved prominent results in pattern recognition tasks, especially computer vision and natural language processing. However, the dataset bias caused by the distribution discrepancy between the training and testing data hinders the generalization ability of deep models. Though many domain adaptation approaches have been proposed to mitigate such negative effect, most of them improve the transferability of features by aligning global distributions of deep models. Few researchers pay attention to the versatility of deep features which can play a vital role in cross-domain recognition. In this paper, we propose to enrich the classic deep learning models by capturing high-low-frequency information and multi-scale features, which deal with the domain shift that cannot be easily addressed by merely feature-level alignment. The Hierarchical Transfer Network (HTN) leverages octave convolution, pyramid features, and self-attention mechanism for revamping the classic models, which can be further integrated with any domain alignment approaches by replacing the feature extractor with the proposed HTN. Extensive experiments have been conducted on three public domain adaptation benchmarks. The results show that the proposed HTN can effectively improve adversarial-based, statistics-based, and norm-based domain adaptation approaches, achieving competitive performance without involving model complexity.
更多查看译文
关键词
Unsupervised domain adaptation,Representation learning,Hierarchical transfer network,Cross-domain classification
AI 理解论文
溯源树
样例
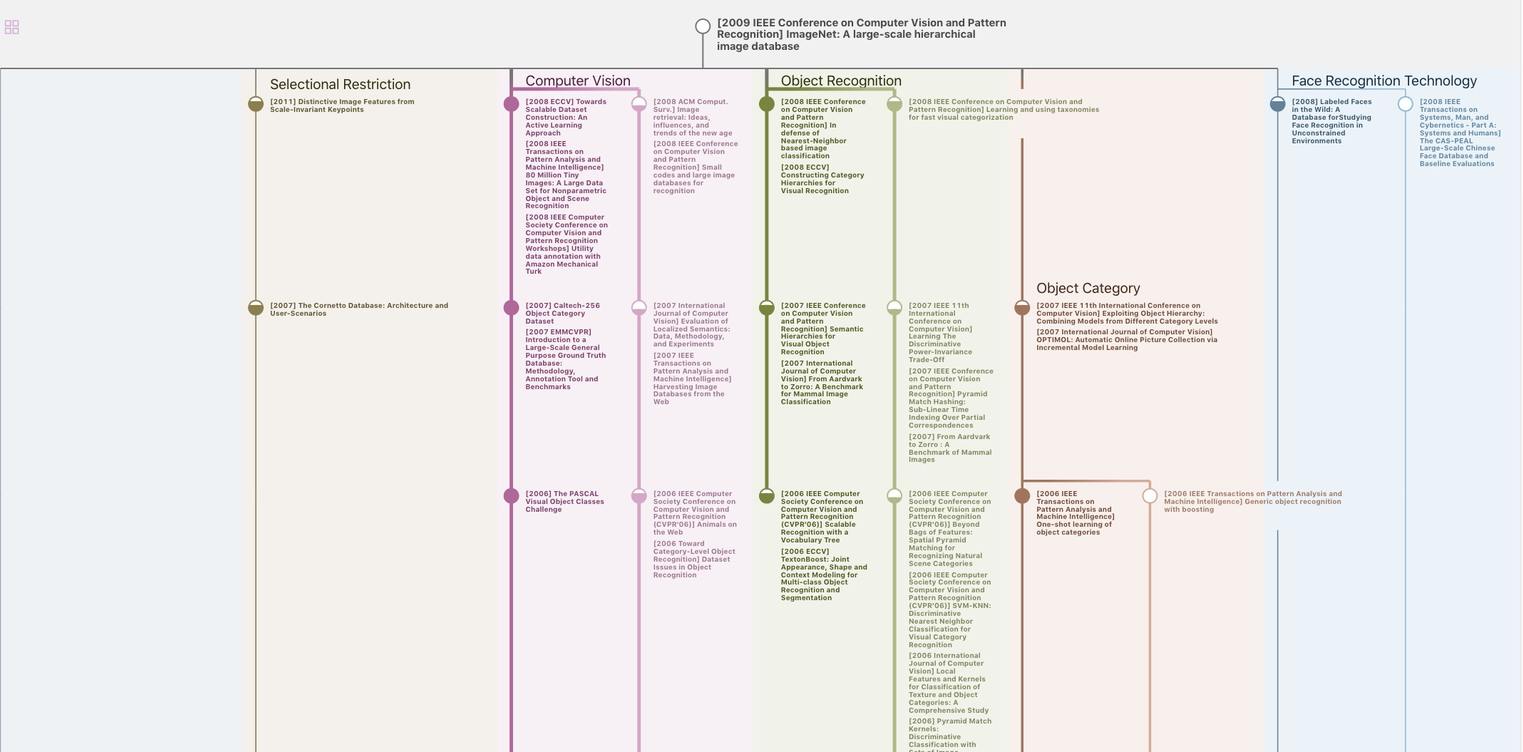
生成溯源树,研究论文发展脉络
Chat Paper
正在生成论文摘要