Crowdsourcing and Evaluating Concept-driven Explanations of Machine Learning Models.
Proc. ACM Hum. Comput. Interact.(2021)
摘要
An important challenge in building explainable artificially intelligent (AI) systems is designing interpretable explanations. AI models often use low-level data features which may be hard for humans to interpret. Recent research suggests that situating machine decisions in abstract, human understandable concepts can help. However, it is challenging to determine the right level of conceptual mapping. In this research, we explore granularity (of data features) and context (of data instances) as dimensions underpinning conceptual mappings. Based on these measures, we explore strategies for designing explanations in classification models. We introduce an end-to-end concept elicitation pipeline that supports gathering high-level concepts for a given data set. Through crowd-sourced experiments, we examine how providing conceptual information shapes the effectiveness of explanations, finding that a balance between coarse and fine-grained explanations help users better estimate model predictions. We organize our findings into systematic themes that can inform design considerations for future systems.
更多查看译文
关键词
classification,concepts,explanations,machine learning
AI 理解论文
溯源树
样例
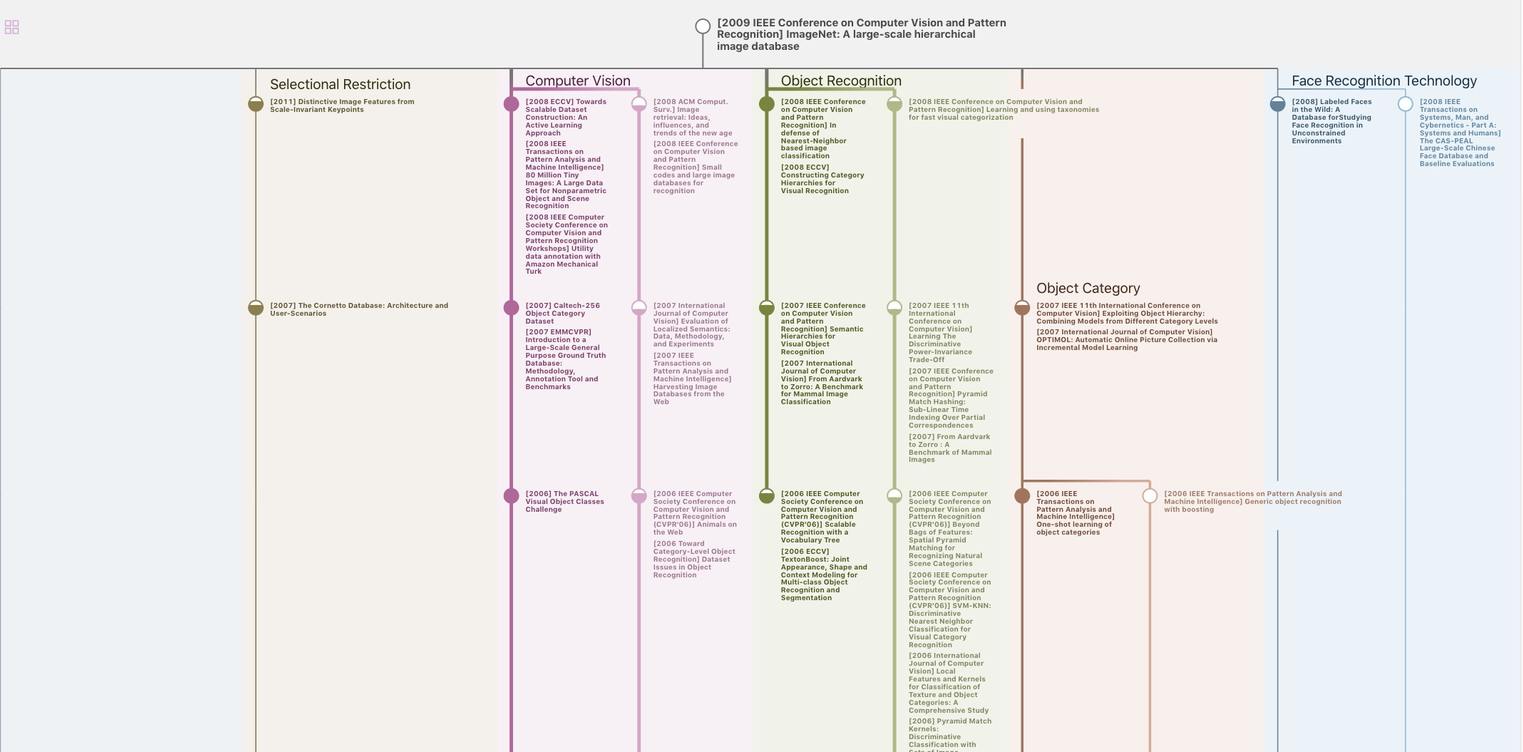
生成溯源树,研究论文发展脉络
Chat Paper
正在生成论文摘要