EMGraph: Fast Learning-Based Electromigration Analysis for Multi-Segment Interconnect Using Graph Convolution Networks
2021 58TH ACM/IEEE DESIGN AUTOMATION CONFERENCE (DAC)(2021)
摘要
Electromigration (EM) becomes a major concern for VLSI circuits as the technology advances in the nanometer regime. With Korhonen equations, EM assessment for VLSI circuits remains challenged due to the increasing integrated density. VLSI multisegment interconnect trees can be naturally viewed as graphs. Based on this observation, we propose a new graph convolution network (GCN) model, which is called EMGraph considering both node and edge embedding features, to estimate the transient EM stress of interconnect trees. Compared with recently proposed generative adversarial network (GAN) based stress image-generation method, EMGraph model can learn more transferable knowledge to predict stress distributions on new graphs without retraining via inductive learning. Trained on the large dataset, the model shows less than 1.5% averaged error compared to the ground truth results and is orders of magnitude faster than both COMSOL and state-of-the-art method. It also achieves smaller model size, 4x accuracy and 14x speedup over the GAN-based method.
更多查看译文
关键词
Electromigration (EM),graph convolution network (GCN),multisegment interconnect,hydrostatic stress assessment
AI 理解论文
溯源树
样例
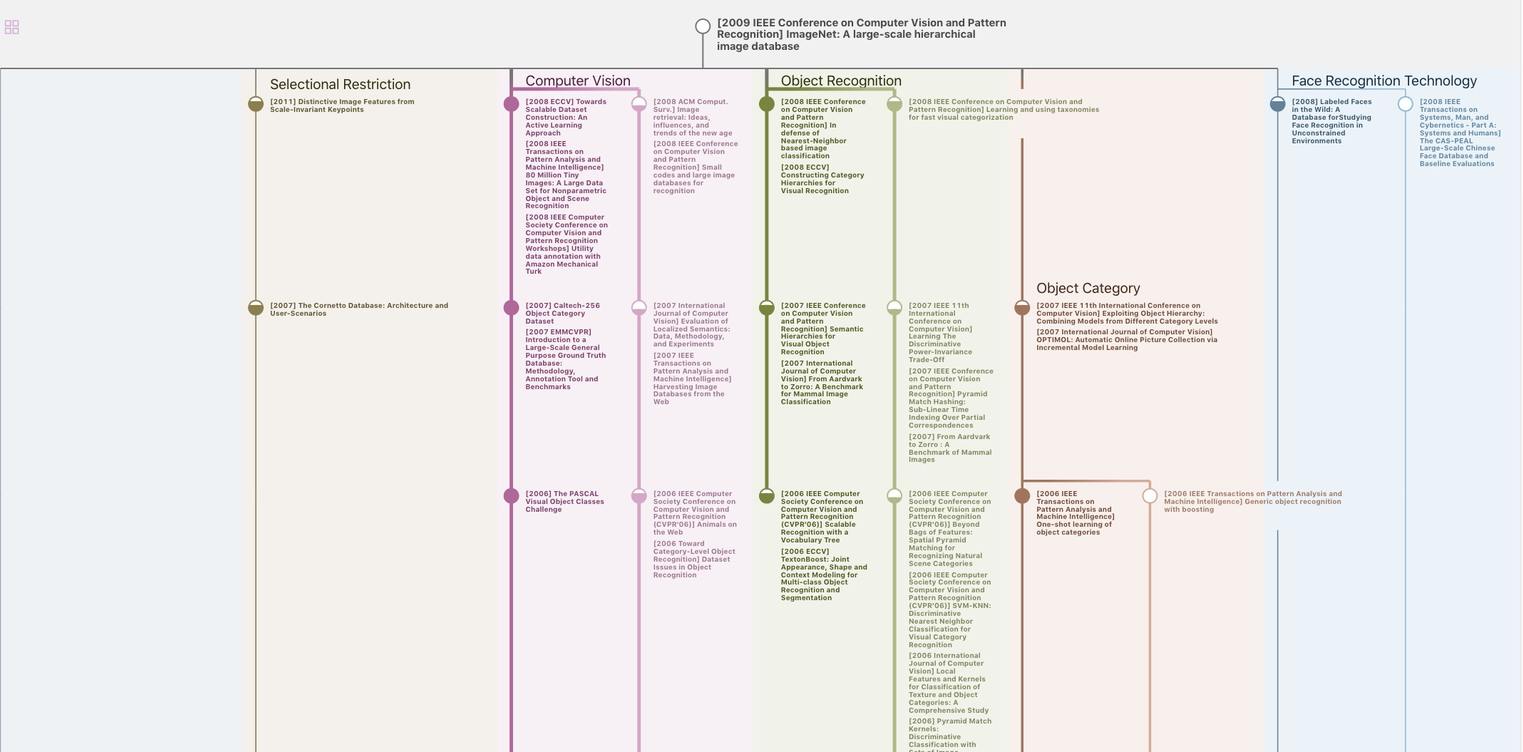
生成溯源树,研究论文发展脉络
Chat Paper
正在生成论文摘要