A Quantum Leap for Fairness: Quantum Bayesian Approach for Fair Decision Making
HCI INTERNATIONAL 2021 - LATE BREAKING PAPERS: MULTIMODALITY, EXTENDED REALITY, AND ARTIFICIAL INTELLIGENCE(2021)
摘要
With the increasing demand for using artificial intelligence algorithms, the need for a fairness-oriented design in automated decision-making systems emerges as a major concern. Since poorly designed algorithms that ignore the fairness criterion in sensitive attributes (e.g., age, race, and gender) may generate or strengthen bias towards specific groups, researchers try to improve the fairness of AI algorithms without compromising their accuracy. Although many studies focused on the optimization of the trade-off between fairness and accuracy in recent years, understanding the sources of unfairness in decision-making is an essential challenge. To tackle this problem, researchers proposed fair causal learning approaches, which enable us to model cause and effects knowledge structure, to discover the sources of the bias, and to prevent unfair decision-making by amplifying transparency and explainability of AI algorithms. These studies consider fair causal learning problems based on the assumption that the underlying probabilistic model of the world is known; whereas, it is well-known that humans do not obey the classical probability rules in making decisions due to emotional changes, subconscious feelings, and subjective biases, and this yields uncertainty in underlying probabilistic models. In this study, we aim to introduce quantum Bayesian approach as a candidate for fair decision-making in causal learning, motivated by the human decision-making literature in cognitive science. We demonstrated that quantum Bayesian perspective creates well-performing fair decision rules under high uncertainty on the well-known COMPAS (Correctional Offender Management Profiling for Alternative Sanctions) data set.
更多查看译文
关键词
Algorithmic bias, Algorithmic fairness, Bayesian decision making, Fair decision making, Quantum Bayesian method
AI 理解论文
溯源树
样例
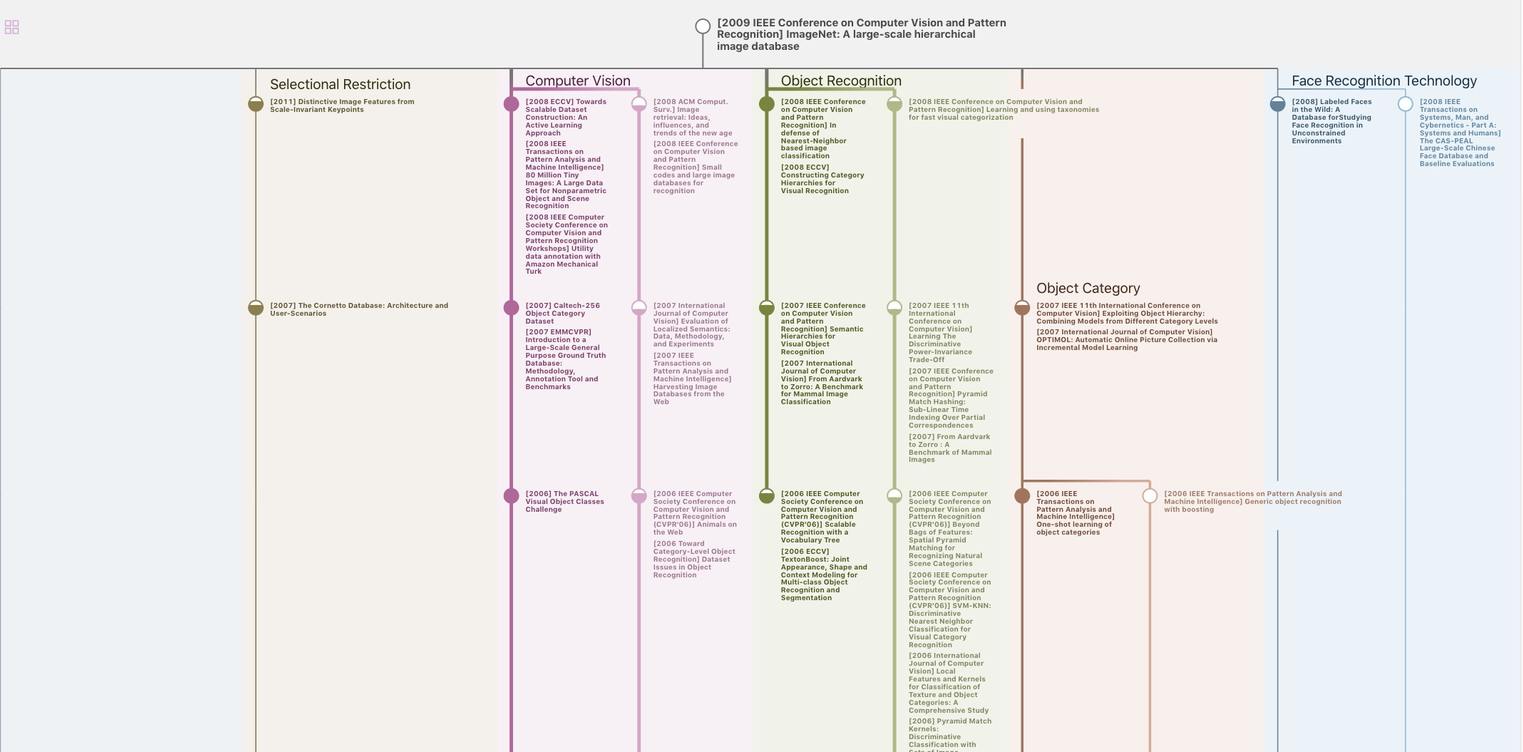
生成溯源树,研究论文发展脉络
Chat Paper
正在生成论文摘要