Joint inference of adaptive and demographic history from temporal population genomic data
biorxiv(2021)
摘要
Disentangling the effects of selection and drift is a long-standing problem in population genetics. Recently, simulations shows that pervasive selection may bias the inference of demography. Ideally, models for the inference of demography and selection should account for the interaction between these two forces. With simulation-based likelihood-free methods such as Approximate Bayesian Computation, demography and selection parameters can be jointly estimated (ABC). We propose a ABC-Random Forests framework to jointly infer demographic and selection parameters from temporal population genomic data (e.g. experimental evolution, monitored populations, ancient DNA). Our framework allowed the separation of demography (census size, N ) from the genetic drift (effective population size, N e), and the estimation of genome-wide parameters of selection. Selection parameters informed us about the adaptive potential of a population (the scale mutation rate of beneficial mutations, θ b), the realized potential, as the number of strong beneficial under selection, and fitness diversity as the population genetic load. We applied this approach to a dataset of feral populations of honey bees ( Apis mellifera ) collected in California, and we estimated parameters consistent with the biology and the recent history of this species.
### Competing Interest Statement
The authors have declared no competing interest.
更多查看译文
关键词
demographic history,joint inference,temporal,population
AI 理解论文
溯源树
样例
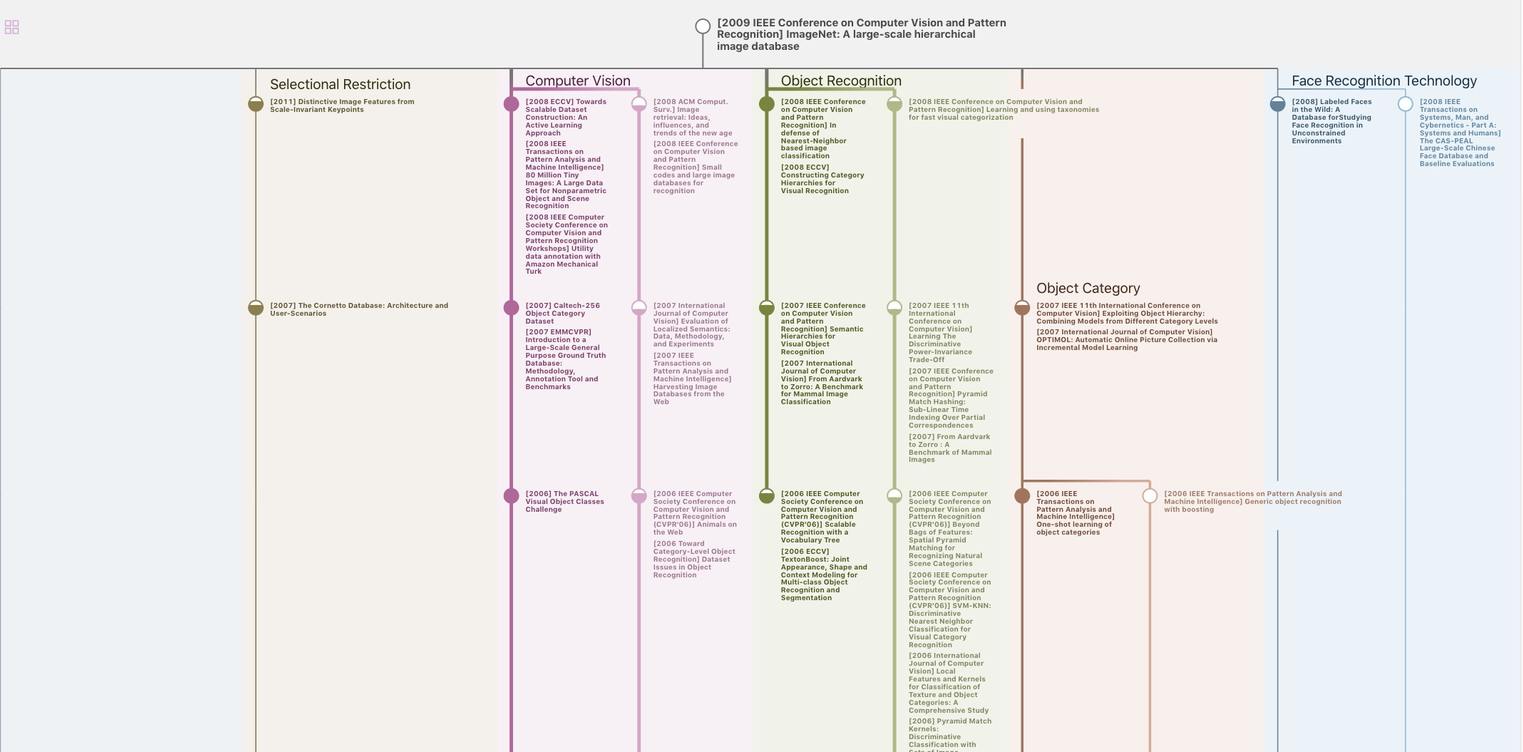
生成溯源树,研究论文发展脉络
Chat Paper
正在生成论文摘要