Effects of short-term plasticity in early olfactory information processing in Drosophila
biorxiv(2021)
摘要
In Drosophila , olfactory information received by the olfactory receptor neurons (ORNs) is first processed by an incoherent feed forward neural circuit in the antennal lobe (AL) that consists of ORNs (input), the inhibitory local neurons (LNs), and projection neurons (PNs). This “early” olfactory information process has two important characteristics. First, response of a PN to its cognate ORN is normalized by the overall activity of other ORNs, a phenomenon termed “divisive normalization”. Second, PNs respond strongly to the onset of ORN activities, but they adapt to prolonged or continuously increasing inputs. Despite the importance of these characteristics for learning and memory, their underlying mechanism remains not fully understood. Here, we develop a circuit model for describing the ORN-LN-PN dynamics by including key features of neuron-neuron interactions, in particular short-term plasticity (STP) and presynaptic inhibition (PI). Our model shows that STP is critical in shaping PN’s steady-state response properties. By fitting our model to experimental data quantitatively, we found that strong and balanced short-term facilitation (STF) and short-term depression (STD) in STP is crucial for the observed nonlinear divisive normalization in Drosophila . By comparing our model with the observed adaptive response to time-varying signals quantitatively, we find that both STP and PI contribute to the highly adaptive response with the latter being the dominant factor for a better fit with experimental data. Our model not only helps reveal the mechanisms underlying two main characteristics of the early olfactory process, it can also be used to predict the PN responses to arbitrary time-dependent signals and to infer microscopic properties of the circuit (such as the strengths of STF and STD) from the measured input-output relation.
### Competing Interest Statement
The authors have declared no competing interest.
更多查看译文
AI 理解论文
溯源树
样例
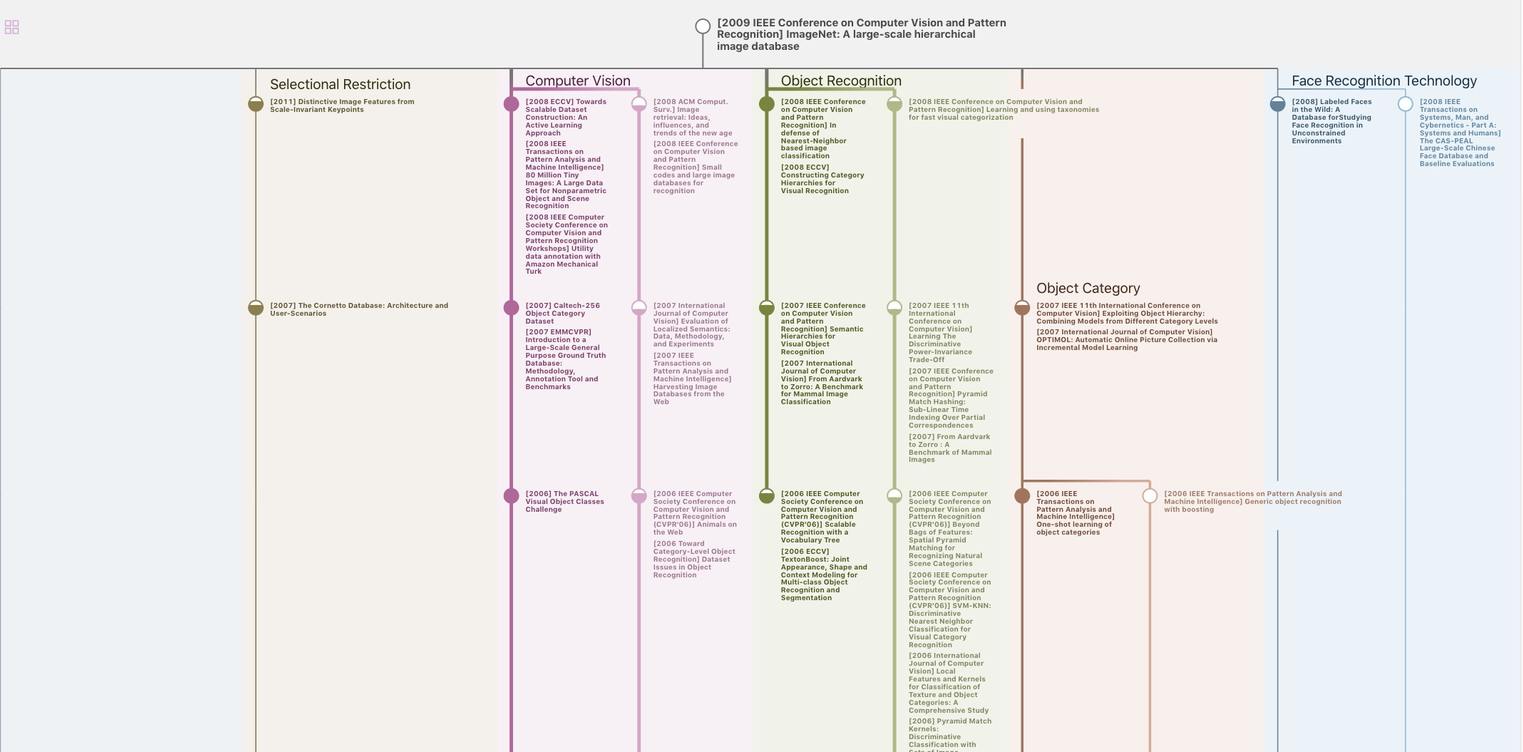
生成溯源树,研究论文发展脉络
Chat Paper
正在生成论文摘要