Deep learning-based subcellular phenotyping of protrusion dynamics reveals fine differential drug responses at subcellular and single-cell levels
BIOPHYSICAL JOURNAL(2022)
摘要
Intracellular processes such as cytoskeletal organization and organelle dynamics exhibit massive subcellular heterogeneity. Although recent advances in fluorescence microscopy allow researchers to acquire an unprecedented amount of live cell image data at high spatiotemporal resolutions, the traditional ensemble-averaging of uncharacterized subcellular heterogeneity could mask important activities. Moreover, the curse of dimensionality of these complex dynamic datasets prevents access to critical mechanistic details of subcellular processes. Here, we establish an unsupervised machine learning framework called DeepHACKS (Deep phenotyping of Heterogeneous Activities in the Coordination of cytosKeleton at the Subcellular level) for “deep phenotyping,” which identifies rare subcellular phenotypes specifically sensitive to molecular perturbations. DeepHACKS dissects the heterogeneity of subcellular time-series datasets by allowing bi-directional LSTM (Long-Short Term Memory) neural networks to extract fine-grained temporal features by integrating autoencoders with conventional machine learning outcomes. We applied DeepHACKS to subcellular protrusion dynamics in pharmacologically perturbed epithelial cells, revealing fine differential responses of leading edge dynamics specific to each perturbation. Particularly, DeepHACKS in conjunction with blebistantin treatment revealed the emergence of rare subcellular and single-cell phenotypes driven by “bursting” protrusion. This suggests that the temporal features directly learned from leading edge dynamics enable fine-grained identification of drug-related phenotypes, which may not be accessible from static cell images. In summary, our study provides an analytical framework for detailed and quantitative understandings of molecular mechanisms hidden in their heterogeneity. DeepHACKS can be potentially applied to analyze various time-series data measured from other subcellular processes.
### Competing Interest Statement
The authors have declared no competing interest.
更多查看译文
关键词
subcellular phenotyping,protrusion dynamics,fine differential drug responses,learning-based,single-cell
AI 理解论文
溯源树
样例
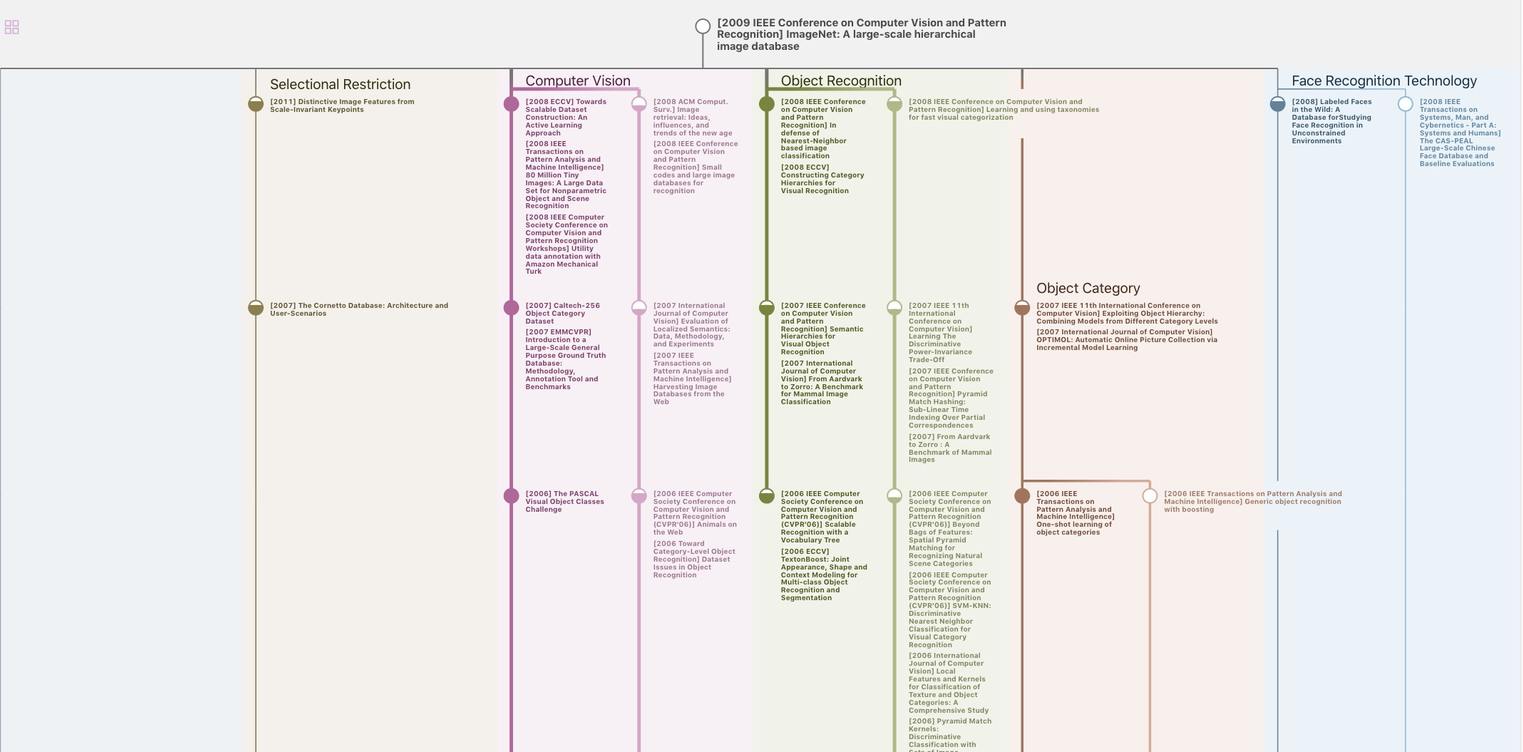
生成溯源树,研究论文发展脉络
Chat Paper
正在生成论文摘要