Prediction and Characterization of Disorder-Order Transition Regions in Proteins by Deep Learning
biorxiv(2021)
摘要
The biological functions of proteins are traditionally thought to depend on well-defined three-dimensional structures, but many experimental studies have shown that disordered regions lacking fixed three-dimensional structures also have crucial biological roles. In some of these regions, disorder–order transitions are also involved in various biological processes, such as protein-protein interaction and ligand binding. Therefore, it is crucial to study disordered regions and structural transitions for further understanding of protein functions and folding. Owing to the costs and time requirements of experimental identification of natively disordered or transitional regions, the development of effective computational methods is a key research goal. In this study, we used overall residue dependencies and deep representation learning for prediction and reused the obtained disordered regions for the prediction of disorder–order transitions. Two similar and related prediction tasks were combined. Firstly, we developed a novel deep learning method, Res-BiLstm, for residue-wise disordered region prediction. Our method outperformed other predictors with respect to almost all criteria, as evaluated using an independent test set. For disorder-order transition prediction, we proposed a transfer learning method, Res-BiLstm-NN, with an acceptable but unbalanced performance, yielding reasonable results. To grasp underlining biophysical principles of disorder-order transitions, we performed qualitative analyses on the obtained results and discovered that most transitions have strong disordered or ordered preferences, and more transitions are consistent with the ordered state than the disordered state, different from conventional wisdom. To the best of our knowledge, this is the first sizable-scale study of transition prediction.
Availability [https://github.com/Yanzziang/Transition\_Disorder\_Prediction][1]
Contact kengo{at}ecei.tohoku.ac.jp
### Competing Interest Statement
The authors have declared no competing interest.
[1]: https://github.com/Yanzziang/Transition_Disorder_Prediction
更多查看译文
AI 理解论文
溯源树
样例
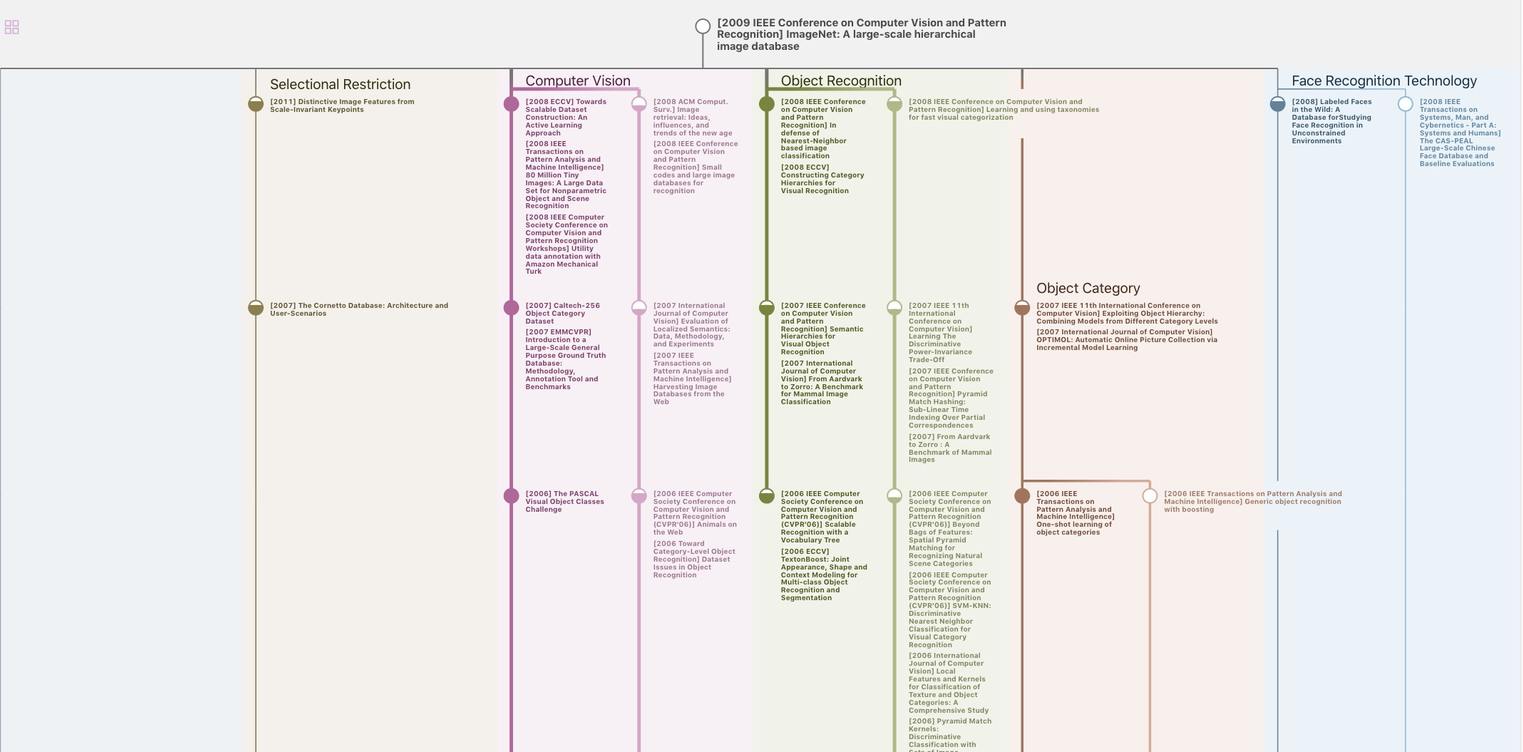
生成溯源树,研究论文发展脉络
Chat Paper
正在生成论文摘要